Instance Selection for GANs
NIPS 2020(2020)
摘要
Recent advances in Generative Adversarial Networks (GANs) have led to their widespread adoption for the purposes of generating high quality synthetic imagery. While capable of generating photo-realistic images, these models often produce unrealistic samples which fall outside of the data manifold. Several recently proposed techniques attempt to avoid spurious samples, either by rejecting them after generation, or by truncating the model's latent space. While effective, these methods are inefficient, as large portions of model capacity are dedicated towards representing samples that will ultimately go unused. In this work we propose a novel approach to improve sample quality: altering the training dataset via instance selection before model training has taken place. To this end, we embed data points into a perceptual feature space and use a simple density model to remove low density regions from the data manifold. By refining the empirical data distribution before training we redirect model capacity towards high-density regions, which ultimately improves sample fidelity. We evaluate our method by training a Self-Attention GAN on ImageNet at 64x64 resolution, where we outperform the current state-of-the-art models on this task while using 1/2 of the parameters. We also highlight training time savings by training a BigGAN on ImageNet at 128x128 resolution, achieving a 66% increase in Inception Score and a 16% improvement in FID over the baseline model with less than 1/4 the training time.
更多查看译文
关键词
gans,selection,instance
AI 理解论文
溯源树
样例
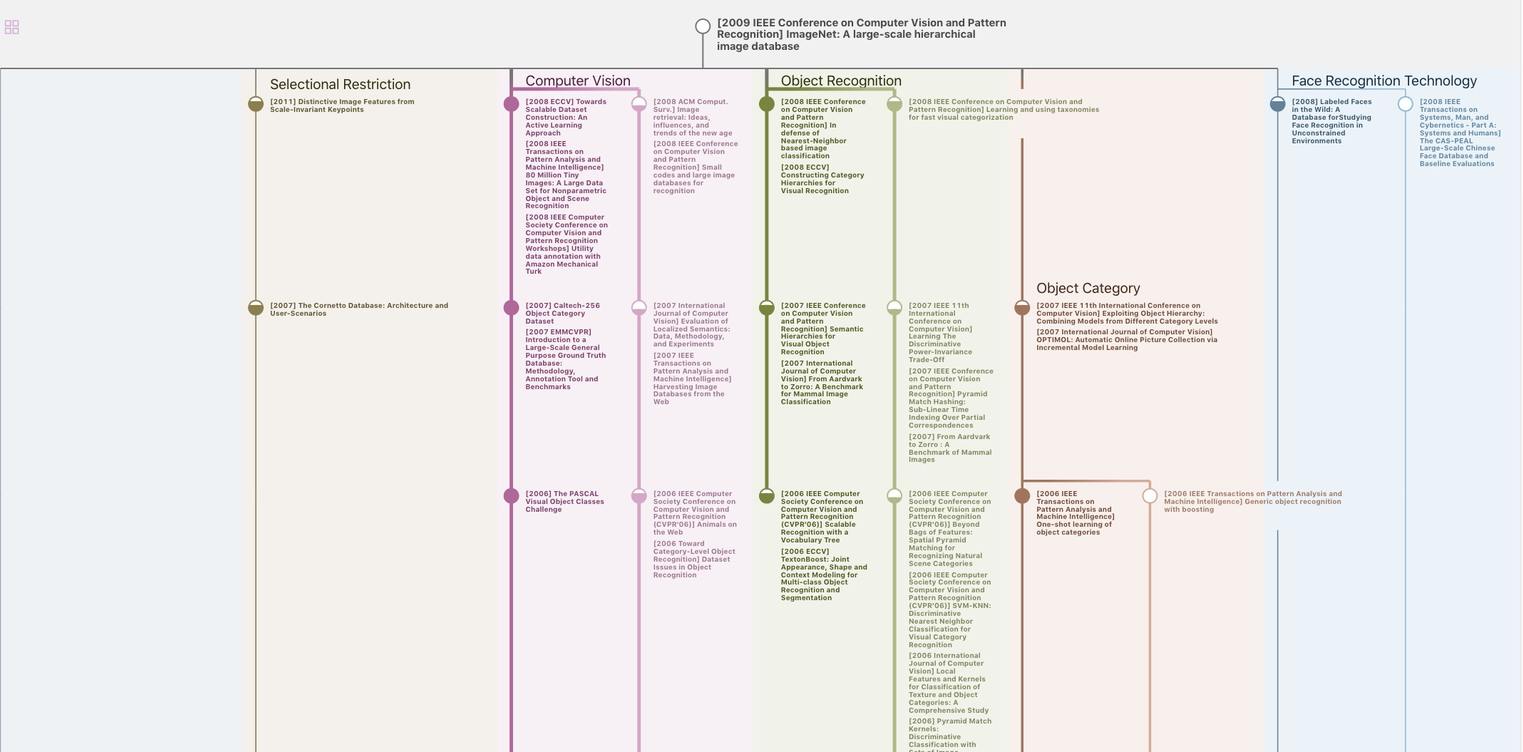
生成溯源树,研究论文发展脉络
Chat Paper
正在生成论文摘要