Assisting Intelligent Wireless Networks with Traffic Prediction: Exploring and Exploiting Predictive Causality in Wireless Traffic
IEEE Communications Magazine(2020)
摘要
Predicting traffic by exploiting its regularity in spatio- temporal variation enables intelligent resource provisioning and management in wireless networks. Traditional prediction techniques are largely based on exploiting traffic spatio-temporal correlation, that is, the statistical relationship between the current traffic and the historical data in a single cell (temporal correlation) or neighboring cells (spatial correlation). Such an approach is effective in predicting the regular components in traffic. However, as traffic types are becoming increasingly diversified, the random components in traffic are becoming dominant. This results in decreasing accuracy in correlation-based prediction techniques. In view of the limitation of existing techniques, we propose a new approach of traffic prediction by exploiting the predictable causality in wireless traffic, which arises from the causal relationship between the occurrences of special events and the triggered traffic variations. Building on the approach, we propose a novel framework of correlation- and causality-based prediction (Coca-Predict) that integrates the two types of prediction to exploit their complementary strengths to maximize prediction accuracy. Specifically, traffic information in spatio-temporal and other dimensions are fed into the correlation and causality sub-predictors to predict the regular component and variation tendency of the traffic, respectively. Experimental results based on realistic traffic data at a specific airport demonstrate that Coca-Predict outperforms the state-of-the-art prediction techniques by exploiting traffic causality. Finally, open challenges and opportunities for wireless traffic prediction are highlighted to shed light on this important direction in designing intelligent wireless networks.
更多查看译文
关键词
intelligent wireless networks,predictive causality,spatio- temporal variation,intelligent resource provisioning,traffic spatio-temporal correlation,spatial correlation,traffic types,correlation-based prediction techniques,predictable causality,causal relationship,triggered traffic variations,causality-based prediction,Coca-Predict,prediction accuracy,traffic information,regular component,variation tendency,realistic traffic data,state-of-the-art prediction techniques,traffic causality,wireless traffic prediction
AI 理解论文
溯源树
样例
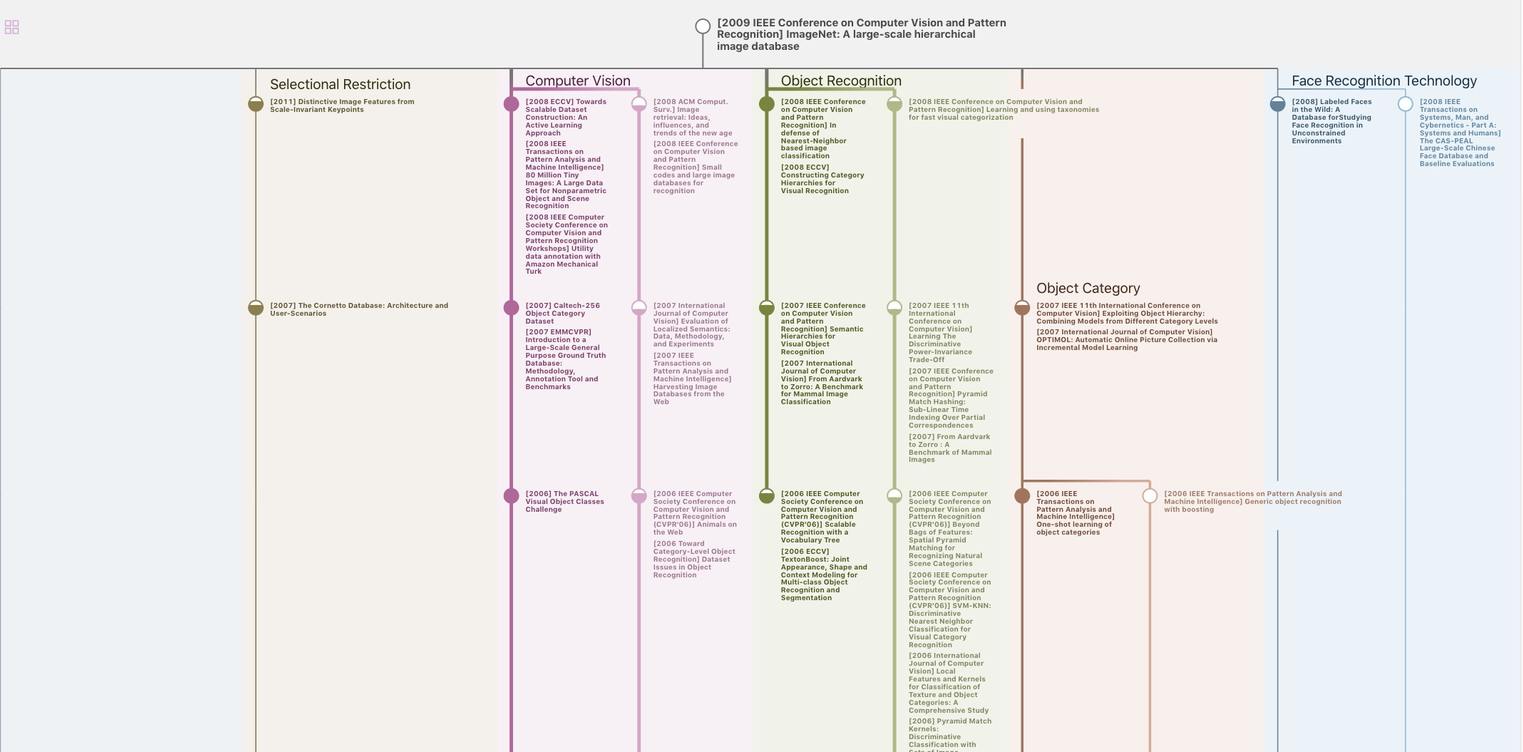
生成溯源树,研究论文发展脉络
Chat Paper
正在生成论文摘要