Context-Dependent Sequence-to-Sequence Turkish Spelling Correction
ACM Transactions on Asian and Low-Resource Language Information Processing(2020)
摘要
AbstractIn this article, we make use of sequence-to-sequence (seq2seq) models for spelling correction in the agglutinative Turkish language. In the baseline system, misspelled and target words are split into their letters and the letter sequences are fed into the seq2seq model. We prefer letters as the unit of the model due to the agglutinative nature of Turkish, which results in an impractical dictionary size when words are used as a dictionary unit. In order to improve the baseline performance, we incorporate right and left context of the misspelled words. All context words are represented with their first three consonants in the context-dependent model. We train the seq2seq models using a large text corpus collected automatically from the Internet. The corpus contains approximately 4 million sentences. We randomly introduce substitution, deletion, and insertion spelling errors to the words in the corpus. We test the performance of the proposed context-dependent seq2seq model using synthetic and realistic test sets. The synthetic test set is constructed similar to the training set. The realistic test set contains human-made misspellings from Twitter messages. In the experiments, we observed that the proposed context-dependent model performs significantly better than the baseline system. Its correction accuracy reaches 94% on the synthetic dataset. Additionally, the proposed method provides 2.1% absolute improvement over a state-of-the-art Turkish spelling correction system on the Twitter test set.
更多查看译文
关键词
Spelling correction, sequence-to-sequence models, agglutinative language
AI 理解论文
溯源树
样例
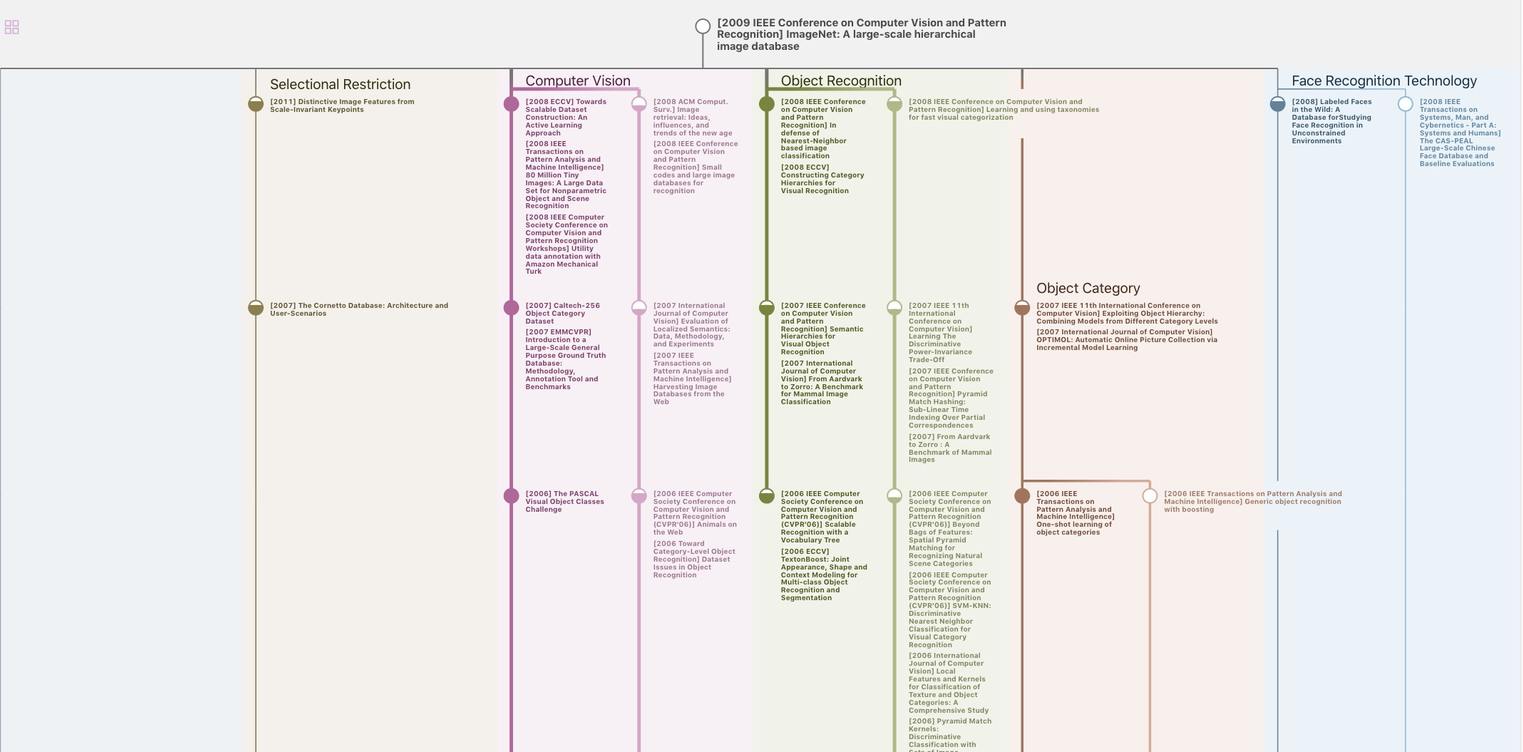
生成溯源树,研究论文发展脉络
Chat Paper
正在生成论文摘要