Multi-target tracking with an adaptive $\delta-$GLMB filter
arxiv(2020)
摘要
Abstract In multi-target tracking, targets can appear and disappear in the surveillance region, randomly varying the number of targets and their locations throughout the tracking process. Moreover, apart from measurement noise, observations of the targets are corrupted by misdetections, and false alarms. Therefore, prior information such as the target birth locations, amount of measurement clutter (false alarms) produced by the sensor, and the probability of detection targets have to be taken into account to model the multi-target system as realistic as possible. In general, such information is not available. As a result, the tracking algorithms have to be supplied with intuitive guesses of these values, which usually results in inferior performances. Therefore, accurate inference of these parameters is paramount for achieving acceptable tracking performance in practice. In this paper, we propose a plug-and-play multi-target tracking algorithm based on the recent $\delta$-Generalized Labeled Multi-Bernoulli $\delta$-GLMB) filter which remove the guess work in determining the parameters of the target birth process, the detection probability, and clutter rate online. The simulation results of a tracking scenario with targets having linear and nonlinear motion models prove the efficacy of the proposed algorithm.
更多查看译文
关键词
Adaptive birth model,Multi-object Bayes filter,Bootstrapping,GLMB Filter,Unknown clutter rate,Unknown detection probability
AI 理解论文
溯源树
样例
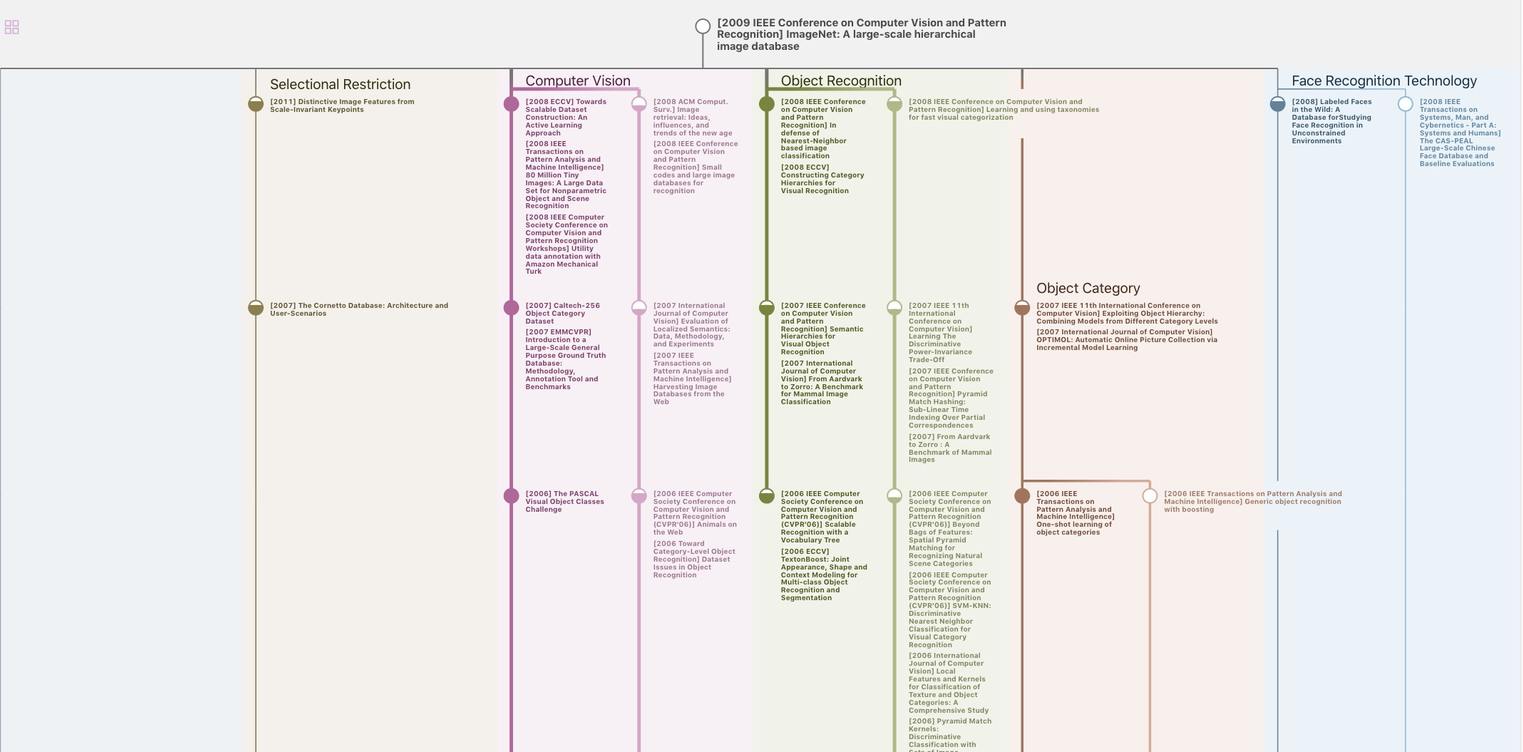
生成溯源树,研究论文发展脉络
Chat Paper
正在生成论文摘要