Chances and challenges of machine learning-based disease classification in genetic association studies illustrated on age-related macular degeneration.
GENETIC EPIDEMIOLOGY(2020)
摘要
Imaging technology and machine learning algorithms for disease classification set the stage for high-throughput phenotyping and promising new avenues for genome-wide association studies (GWAS). Despite emerging algorithms, there has been no successful application in GWAS so far. We establish machine learning-based phenotyping in genetic association analysis as misclassification problem. To evaluate chances and challenges, we performed a GWAS based on automatically classified age-related macular degeneration (AMD) in UK Biobank (images from 135,500 eyes; 68,400 persons). We quantified misclassification of automatically derived AMD in internal validation data (4,001 eyes; 2,013 persons) and developed a maximum likelihood approach (MLA) to account for it when estimating genetic association. We demonstrate that our MLA guards against bias and artifacts in simulation studies. By combining a GWAS on automatically derived AMD and our MLA in UK Biobank data, we were able to dissect true association (ARMS2/HTRA1,CFH) from artifacts (nearHERC2) and identified eye color as associated with the misclassification. On this example, we provide a proof-of-concept that a GWAS using machine learning-derived disease classification yields relevant results and that misclassification needs to be considered in analysis. These findings generalize to other phenotypes and emphasize the utility of genetic data for understanding misclassification structure of machine learning algorithms.
更多查看译文
关键词
age-related macular degeneration (AMD),genome-wide association study,machine learning-based disease classification,response misclassification,UK Biobank
AI 理解论文
溯源树
样例
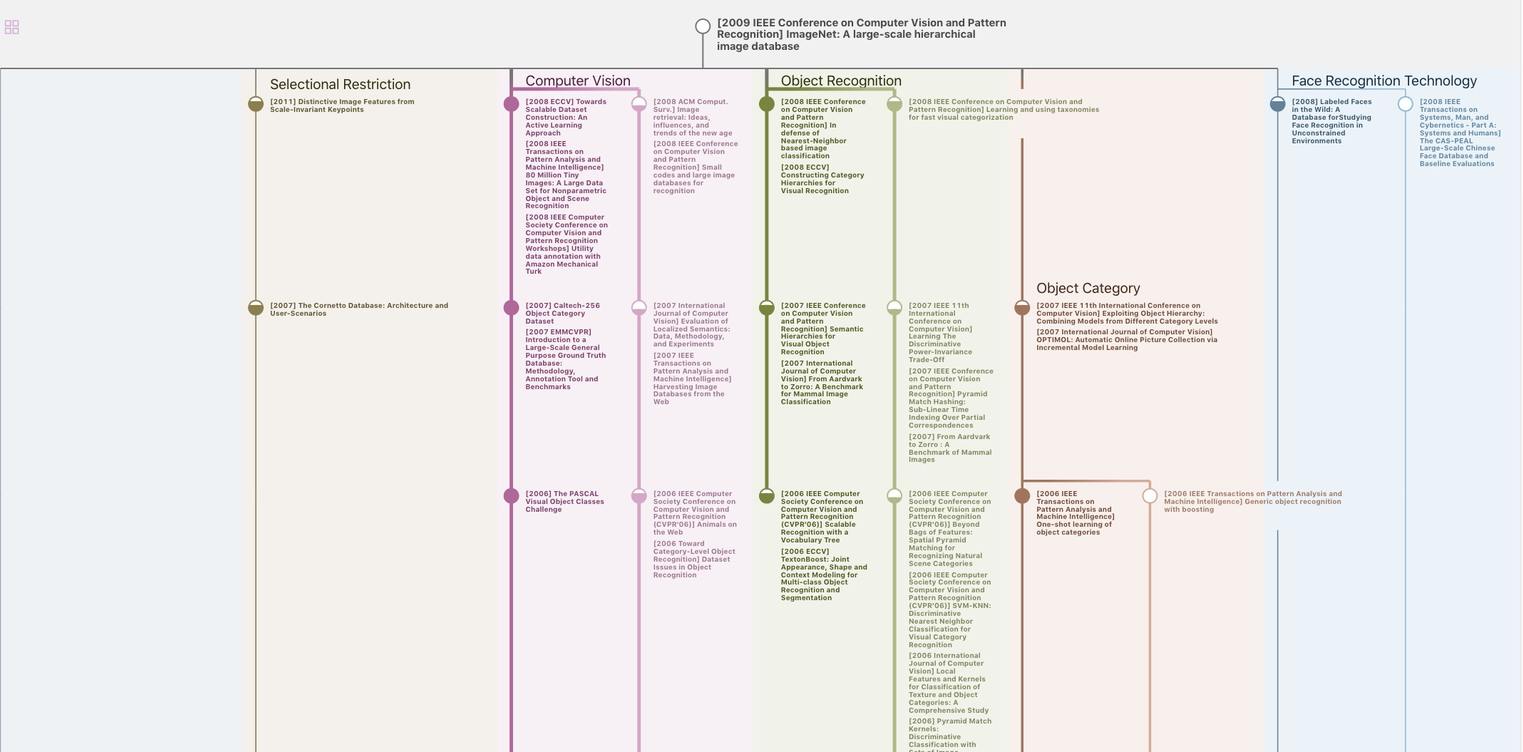
生成溯源树,研究论文发展脉络
Chat Paper
正在生成论文摘要