Shape Adaptor: A Learnable Resizing Module
European Conference on Computer Vision(2020)
摘要
We present a novel resizing module for neural networks: shape adaptor, a drop-in enhancement built on top of traditional resizing layers, such as pooling, bilinear sampling, and strided convolution. Whilst traditional resizing layers have fixed and deterministic reshaping factors, our module allows for a learnable reshaping factor. Our implementation enables shape adaptors to be trained end-to-end without any additional supervision, through which network architectures can be optimised for each individual task, in a fully automated way. We performed experiments across seven image classification datasets, and results show that by simply using a set of our shape adaptors instead of the original resizing layers, performance increases consistently over human-designed networks, across all datasets. Additionally, we show the effectiveness of shape adaptors on two other applications: network compression and transfer learning.
更多查看译文
关键词
shape adaptor
AI 理解论文
溯源树
样例
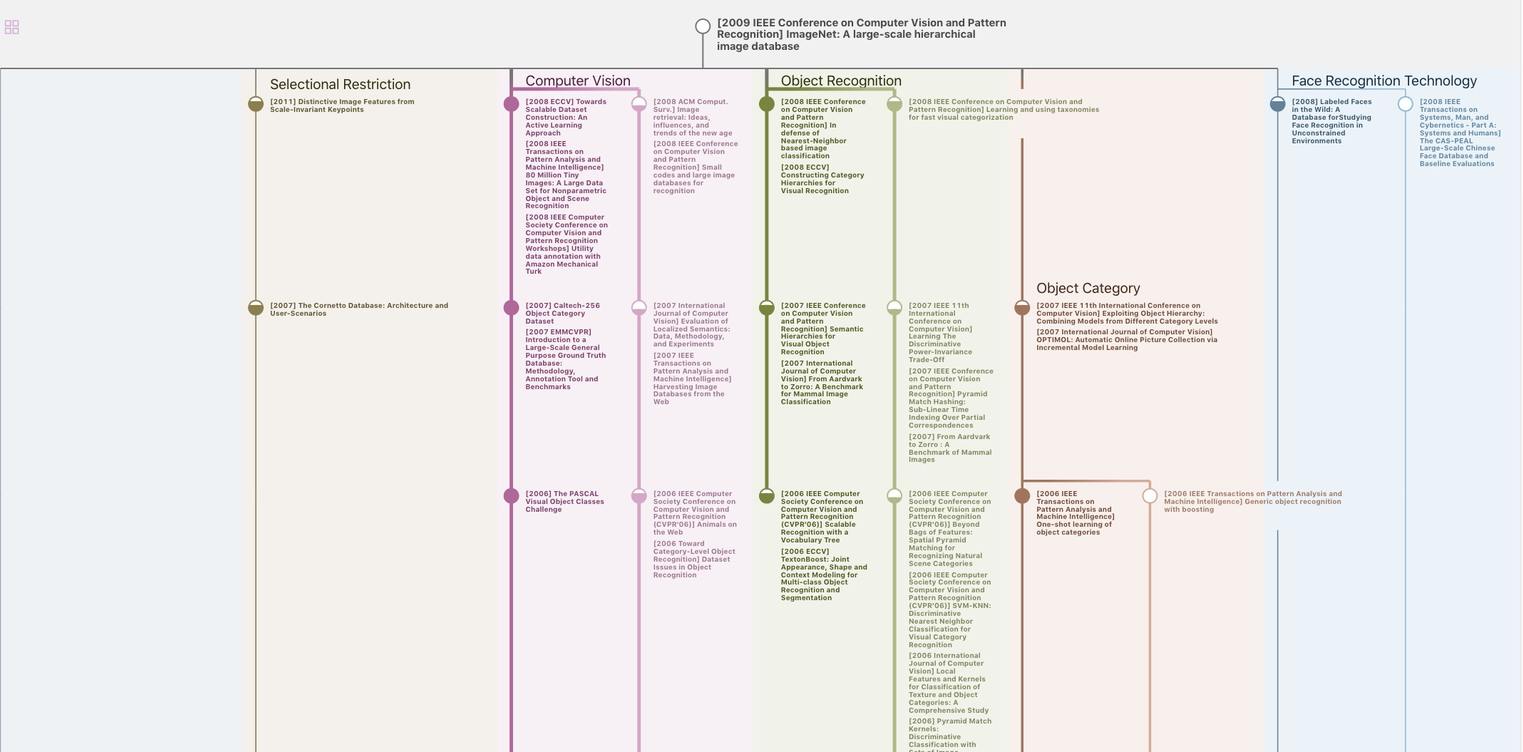
生成溯源树,研究论文发展脉络
Chat Paper
正在生成论文摘要