Automated Segmentation of Brain Gray Matter Nuclei on Quantitative Susceptibility Mapping Using Deep Convolutional Neural Network
arxiv(2020)
摘要
Abnormal iron accumulation in the brain subcortical nuclei has been reported to be correlated to various neurodegenerative diseases, which can be measured through the magnetic susceptibility from the quantitative susceptibility mapping (QSM). To quantitively measure the magnetic susceptibility, the nuclei should be accurately segmented, which is a tedious task for clinicians. In this paper, we proposed a double-branch residual-structured U-Net (DB-ResUNet) based on 3D convolutional neural network (CNN) to automatically segment such brain gray matter nuclei. To better tradeoff between segmentation accuracy and the memory efficiency, the proposed DB-ResUNet fed image patches with high resolution and the patches with low resolution but larger field of view into the local and global branches, respectively. Experimental results revealed that by jointly using QSM and T$_\text{1}$ weighted imaging (T$_\text{1}$WI) as inputs, the proposed method was able to achieve better segmentation accuracy over its single-branch counterpart, as well as the conventional atlas-based method and the classical 3D-UNet structure. The susceptibility values and the volumes were also measured, which indicated that the measurements from the proposed DB-ResUNet are able to present high correlation with values from the manually annotated regions of interest.
更多查看译文
关键词
brain gray matter nuclei,quantitative susceptibility mapping,neural,segmentation
AI 理解论文
溯源树
样例
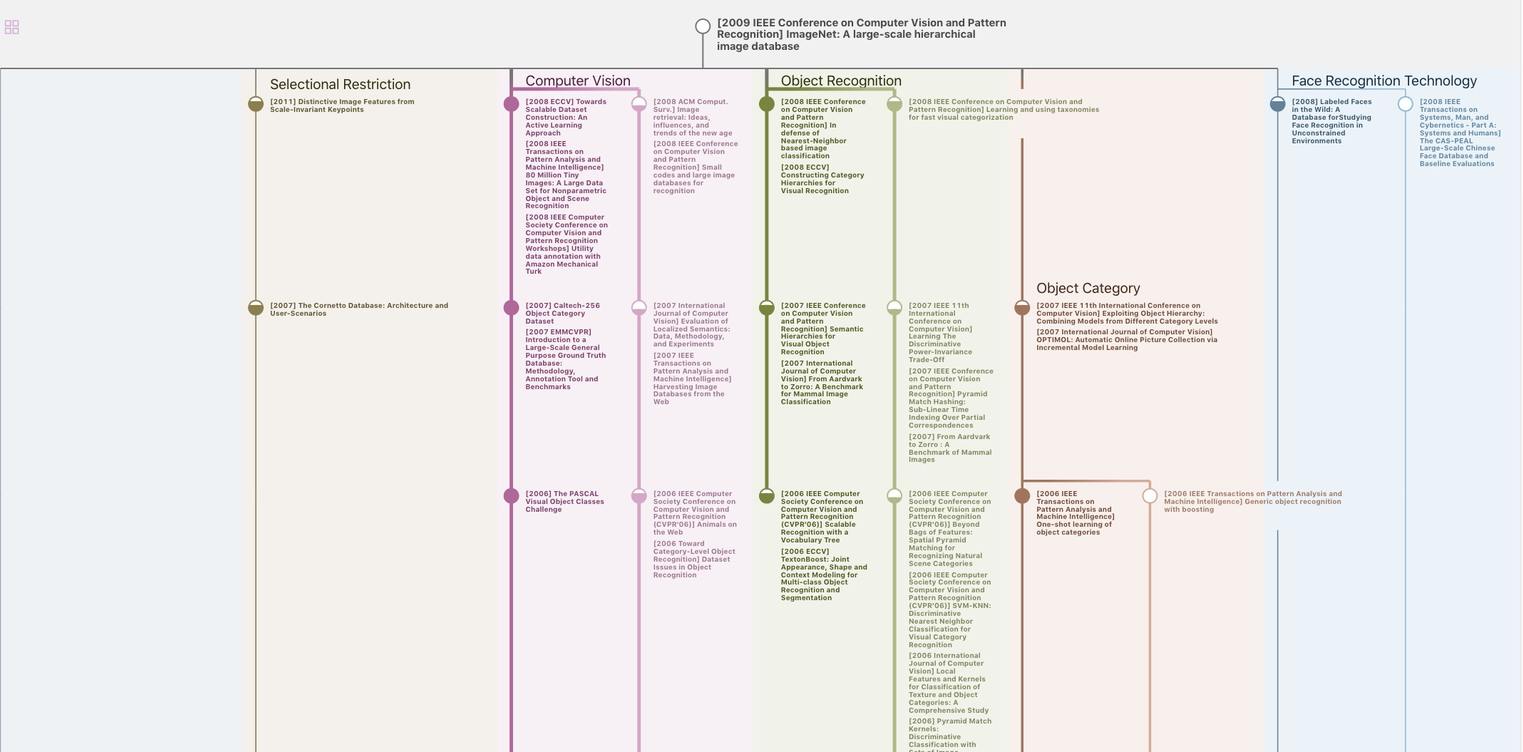
生成溯源树,研究论文发展脉络
Chat Paper
正在生成论文摘要