Deep traffic sign detection and recognition without target domain real images
Machine Vision and Applications(2022)
摘要
Deep learning has become a standard approach to machine vision in recent years. Despite several advances, it requires large amounts of annotated data. Nonetheless, in many applications, large-scale data acquisition and annotation is expensive and data imbalance is an intrinsic problem. To address these challenges, we propose a novel synthetic database generation method that only requires (i) arbitrary natural images, i.e., does not demand real images from the target domain, and (ii) templates of the traffic signs. Our method does not aim at overcoming the training with real data but to be a compatible option when there is a lack of real data. Results with data of multiple countries show that the synthetic database generated without human effort is effective for training a deep traffic sign detector. On large datasets, training with a fully synthetic dataset almost matches the performance of training with a real one. When compared to training with a smaller dataset of real images, training with synthetic images increased the accuracy by 12.25%. The proposed method also improves the performance of the detector when target-domain data are available.
更多查看译文
关键词
Traffic sign detection,Deep learning,Autonomous driving,Object detection
AI 理解论文
溯源树
样例
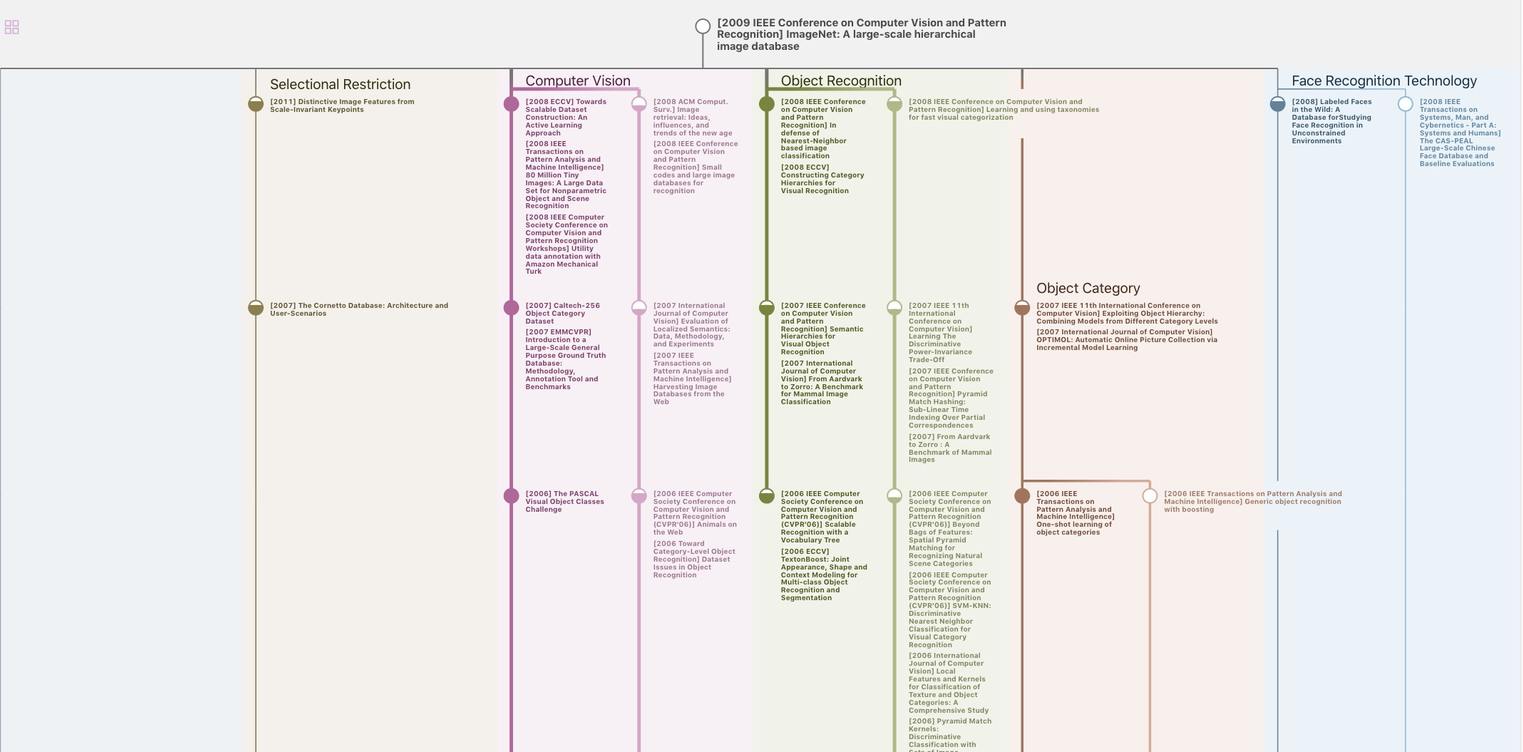
生成溯源树,研究论文发展脉络
Chat Paper
正在生成论文摘要