Deep Reinforcement Learning using Cyclical Learning Rates
2020 Third International Conference on Artificial Intelligence for Industries (AI4I)(2020)
摘要
Deep Reinforcement Learning (DRL) methods often rely on the meticulous tuning of hyperparameters to successfully resolve problems. One of the most influential parameters in optimization procedures based on stochastic gradient descent (SGD) is the learning rate. We investigate cyclical learning and propose a method for defining a general cyclical learning rate for various DRL problems. In this paper we present a method for cyclical learning applied to complex DRL problems. Our experiments show that, utilizing cyclical learning achieves similar or even better results than highly tuned fixed learning rates. This paper presents the first application of cyclical learning rates in DRL settings and is a step towards overcoming manual hyperparameter tuning.
更多查看译文
关键词
Deep Reinforcement Learning, Cyclic Learning Rates, OpenAI, Gym, Cyclic, Learning
AI 理解论文
溯源树
样例
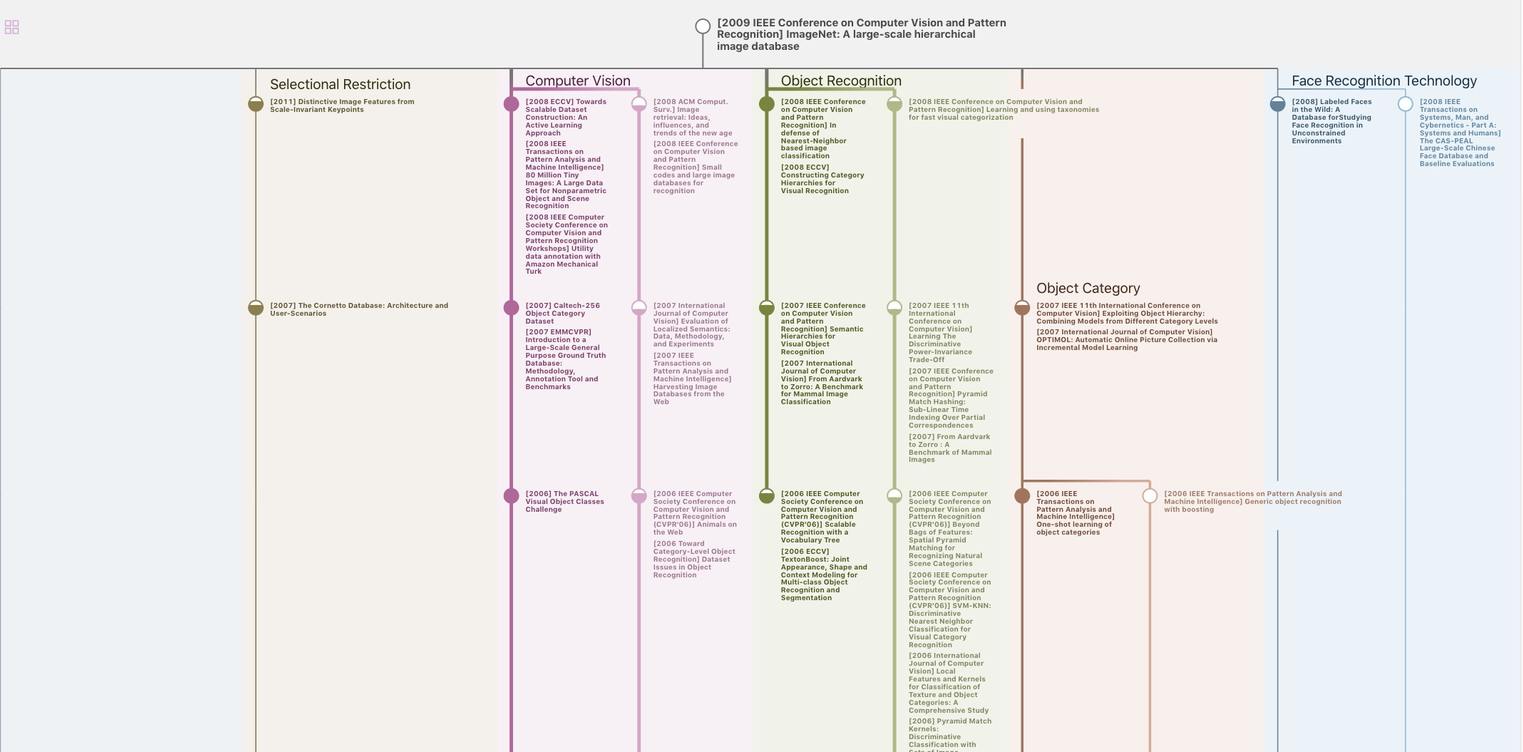
生成溯源树,研究论文发展脉络
Chat Paper
正在生成论文摘要