Context Encoding for Video Retrieval with Contrastive Learning
arxiv(2020)
摘要
Content-based video retrieval plays an important role in areas such as video recommendation, copyright protection, etc. Existing video retrieval methods mainly extract frame-level features independently, therefore lack of efficient aggregation of features between frames, and it is difficult to effectively deal with poor quality frames, such as frames with motion blur, out of focus, etc. In this paper, we propose CECL (Context Encoding for video retrieval with Contrastive Learning), a video representation learning framework that aggregates the context information of frame-level descriptors, and a supervised contrastive learning method that performs automatic hard negative mining, and utilizes the memory bank mechanism to increase the capacity of negative samples. Extensive experiments are conducted on multi video retrieval tasks, such as FIVR, CC_WEB_VIDEO and EVVE. The proposed method shows a significant performance advantage (~17% mAP on FIVR-200K) over state-of-the-art methods with video-level features, and deliver competitive results with much lower computational cost when compared with frame-level features.
更多查看译文
AI 理解论文
溯源树
样例
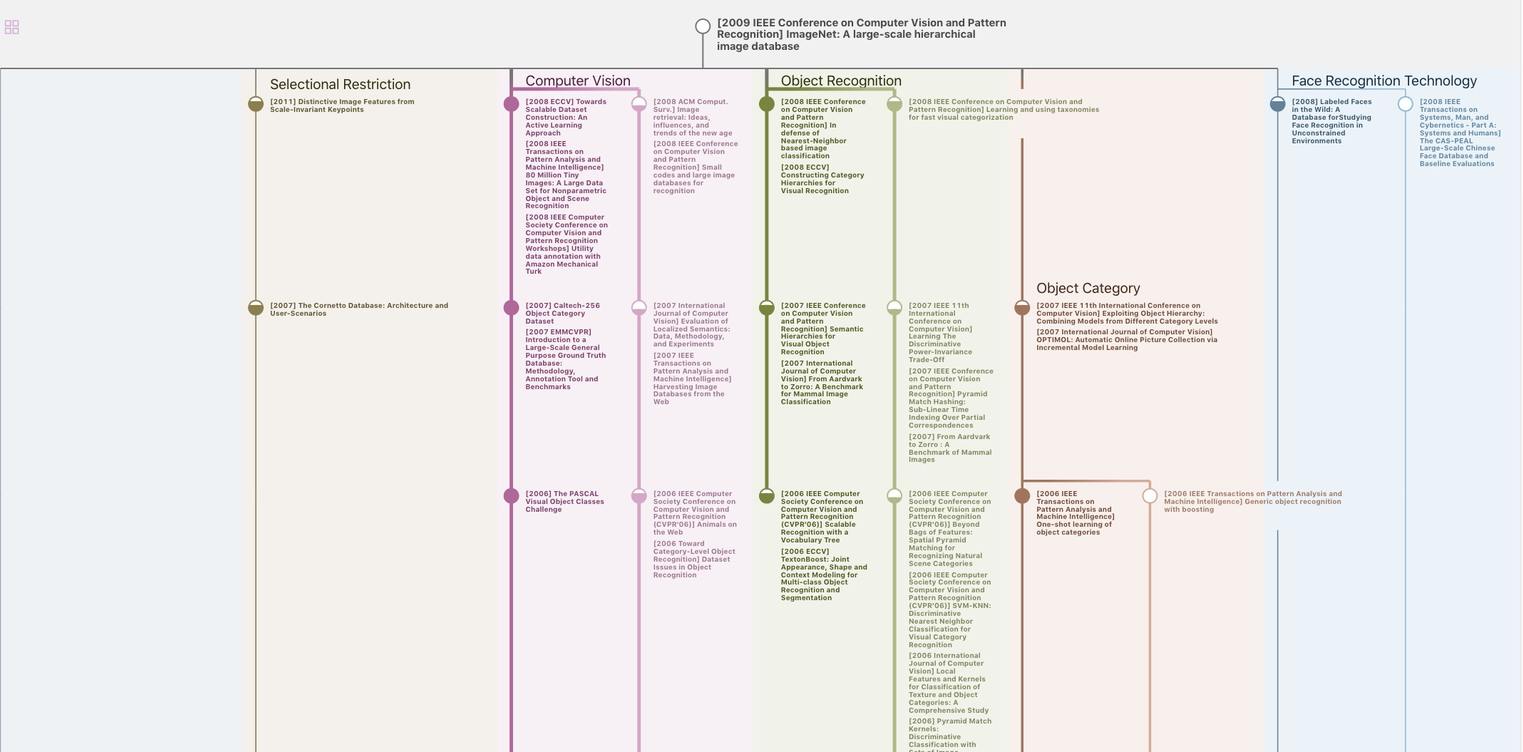
生成溯源树,研究论文发展脉络
Chat Paper
正在生成论文摘要