Human Feedback as Action Assignment in Interactive Reinforcement Learning
ACM Transactions on Autonomous and Adaptive Systems(2020)
摘要
Teaching by demonstrations and teaching by assigning rewards are two popular methods of knowledge transfer in humans. However, showing the right behaviour (by demonstration) may appear more natural to a human teacher than assessing the learner’s performance and assigning a reward or punishment to it. In the context of robot learning, the preference between these two approaches has not been studied extensively. In this article, we propose a method that replaces the traditional method of reward assignment with action assignment (which is similar to providing a demonstration) in interactive reinforcement learning. The main purpose of the suggested action is to compute a reward by seeing if the suggested action was followed by the self-acting agent or not. We compared action assignment with reward assignment via a user study conducted over the web using a two-dimensional maze game. The logs of interactions showed that action assignment significantly improved users’ ability to teach the right behaviour. The survey results showed that both action and reward assignment seemed highly natural and usable, reward assignment required more mental effort, repeatedly assigning rewards and seeing the agent disobey commands caused frustration in users, and many users desired to control the agent’s behaviour directly.
更多查看译文
关键词
Interactive machine learning,learning from human teachers,reinforcement learning,reward shaping
AI 理解论文
溯源树
样例
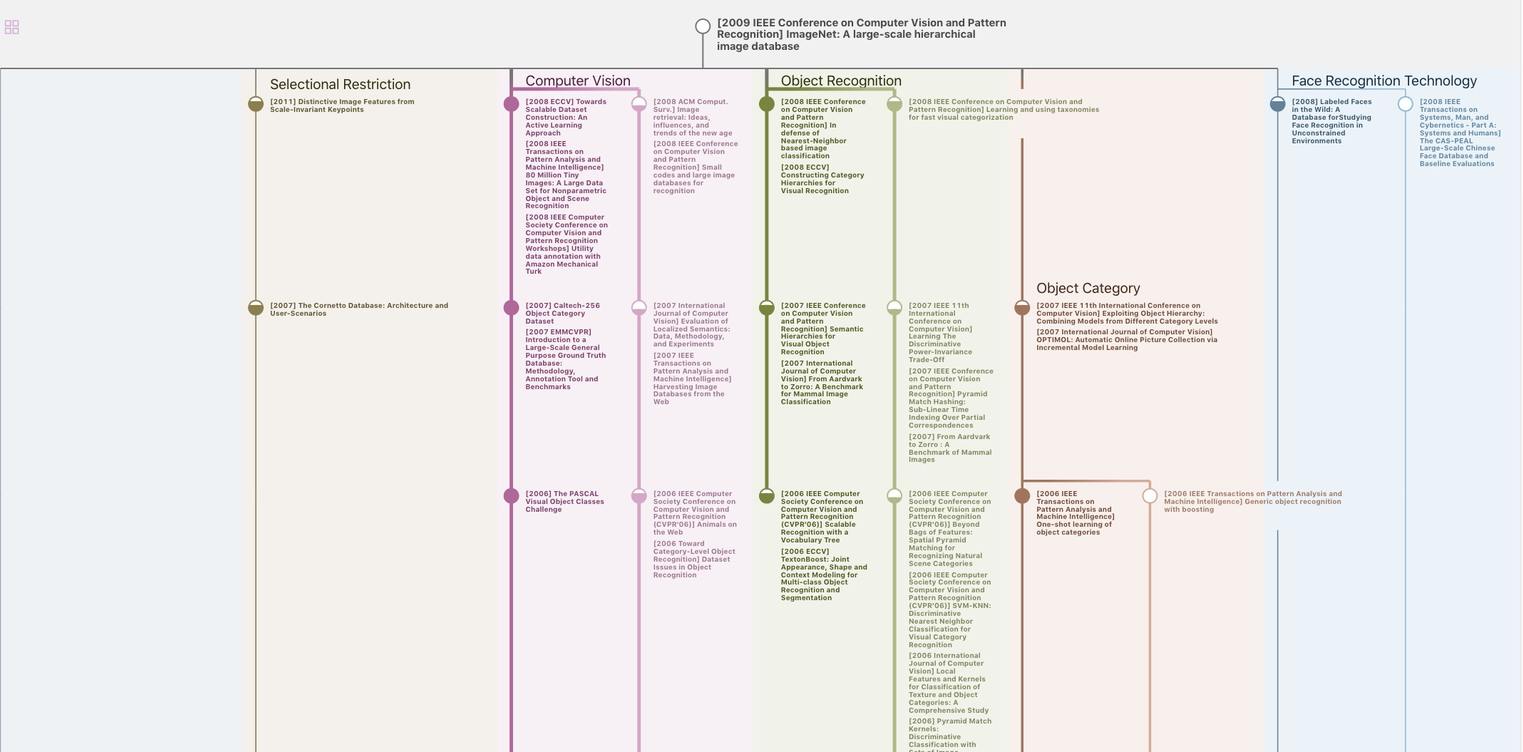
生成溯源树,研究论文发展脉络
Chat Paper
正在生成论文摘要