A Reliable Semi-Supervised Intrusion Detection Model: One Year Of Network Traffic Anomalies
ICC 2020 - 2020 IEEE INTERNATIONAL CONFERENCE ON COMMUNICATIONS (ICC)(2020)
摘要
Despite the promising results of machine learning for network-based intrusion detection, current techniques are not widely deployed in real-world environments. In general, proposed detection models quickly become obsolete, thus, generating unreliable classifications over time. In this paper, we propose a new reliable model for semi-supervised intrusion detection that uses a verification technique to provide reliable classifications over time, even in the absence of model updates. Additionally, we cope with this verification technique with semi-supervised learning to autonomously update the underlying machine learning models without human assistance. Our experiments consider a full year of real network traffic and demonstrate that our solution maintains the accuracy rate over time without model updates while rejecting only 10.6% of instances on average. Moreover, when autonomous (non-human-assisted) model updates are performed, the average rejection rate drops to just 3.2% without affecting the accuracy of our solution.
更多查看译文
关键词
Intrusion Detection, Machine Learning, Classification Reliability, Semi-Supervised Co-Learning
AI 理解论文
溯源树
样例
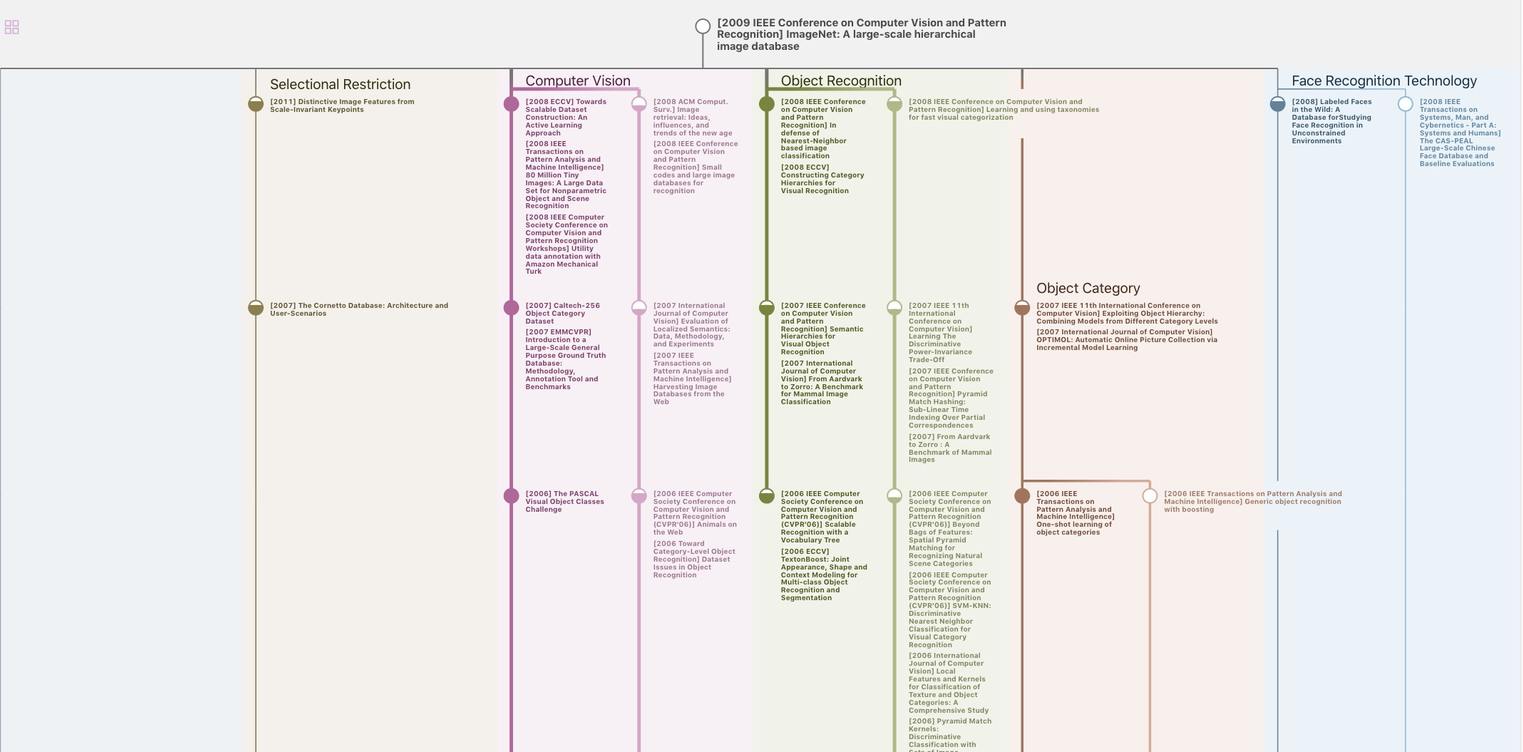
生成溯源树,研究论文发展脉络
Chat Paper
正在生成论文摘要