Dehaze of Cataractous Retinal Images Using an Unpaired Generative Adversarial Network
IEEE Journal of Biomedical and Health Informatics(2020)
摘要
Cataracts are the leading cause of visual impairment worldwide. Examination of the retina through cataracts using a fundus camera is challenging and error-prone due to degraded image quality. We sought to develop an algorithm to dehaze such images to support diagnosis by either ophthalmologists or computer-aided diagnosis systems. Based on the generative adversarial network (GAN) concept, we designed two neural networks: CataractSimGAN and CataractDehazeNet. CataractSimGAN was intended for the synthesis of cataract-like images through unpaired clear retinal images and cataract images. CataractDehazeNet was trained using pairs of synthesized cataract-like images and the corresponding clear images through supervised learning. With two networks trained independently, the number of hyper-parameters was reduced, leading to better performance. We collected 400 retinal images without cataracts and 400 hazy images from cataract patients as the training dataset. Fifty cataract images and the corresponding clear images from the same patients after surgery comprised the test dataset. The clear images after surgery were used for reference to evaluate the performance of our method. CataractDehazeNet was able to enhance the degraded image from cataract patients substantially and to visualize blood vessels and the optic disc, while actively suppressing the artifacts common in application of similar methods. Thus, we developed an algorithm to improve the quality of the retinal images acquired from cataract patients. We achieved high structure similarity and fidelity between processed images and images from the same patients after cataract surgery.
更多查看译文
关键词
Aged,Aged, 80 and over,Algorithms,Cataract,Cataract Extraction,Deep Learning,Female,Humans,Image Processing, Computer-Assisted,Male,Middle Aged,Neural Networks, Computer,Retina
AI 理解论文
溯源树
样例
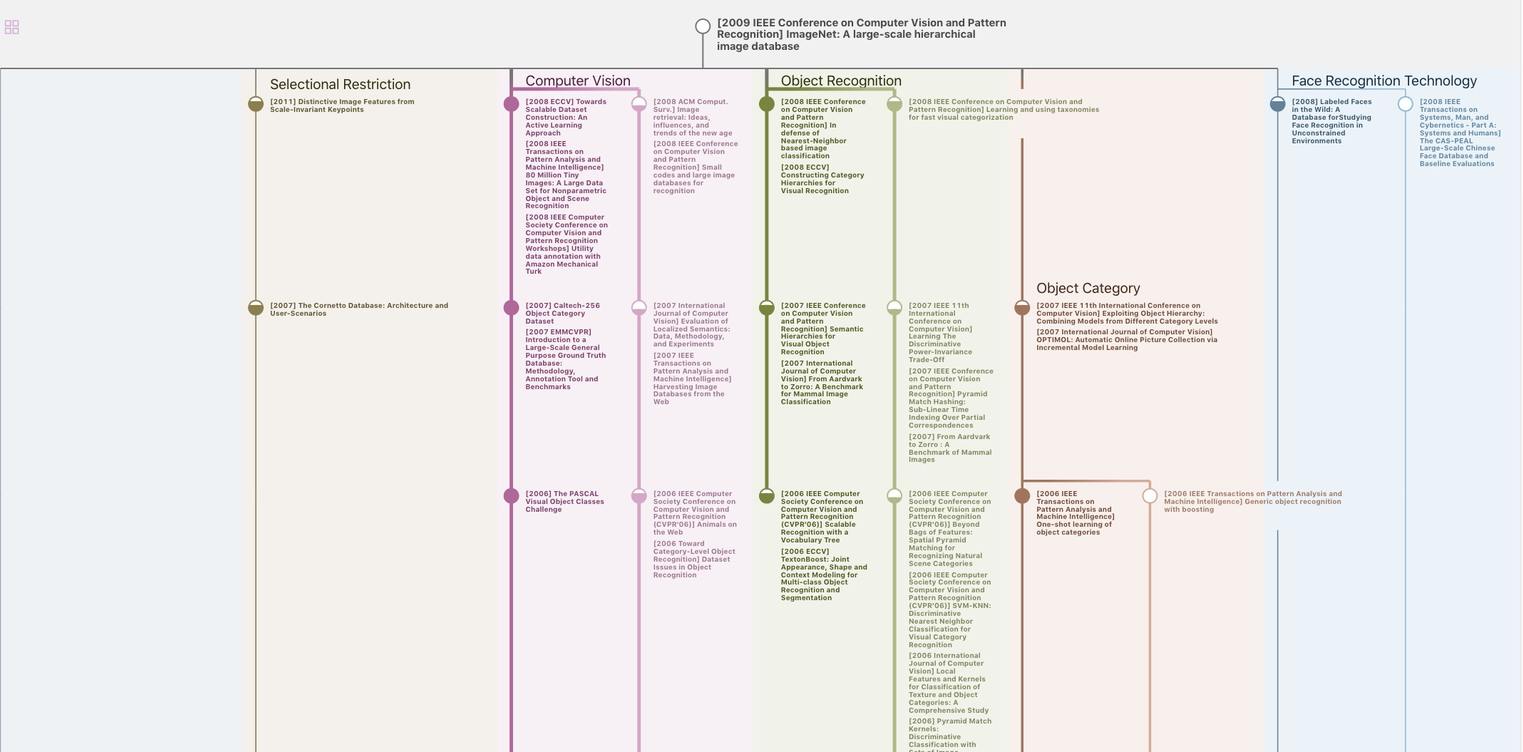
生成溯源树,研究论文发展脉络
Chat Paper
正在生成论文摘要