Decentralized Learning With Lazy and Approximate Dual Gradients
IEEE Transactions on Signal Processing(2021)
摘要
This paper develops algorithms for decentralized machine learning over a network, where data are distributed, computation is localized, and communication is restricted between neighbors. A line of recent research in this area focuses on improving both computation and communication complexities. The methods SSDA and MSDA (Scaman et al., 2017) have optimal communication complexity when the objective is smooth and strongly convex, and are simple to derive. However, they require solving a subproblem at each step, so both the required accuracy of subproblem solutions and total computational complexities are uncertain. We propose new algorithms that instead of solving a subproblem, run warm-started Katyusha for a small, fixed number of steps. In addition, when previous information is sufficiently useful, a local rule will decide even to skip a round of communication, leading to extra savings. We show that our algorithms are efficient in both computation and communication, provably reducing the communication and computation complexities of SSDA and MSDA. In numerical experiments, our algorithms achieve significant computation and communication reduction compared with the state-of-the-art.
更多查看译文
关键词
Decentralized machine learning,extra communication reduction,cheap subproblem procedures
AI 理解论文
溯源树
样例
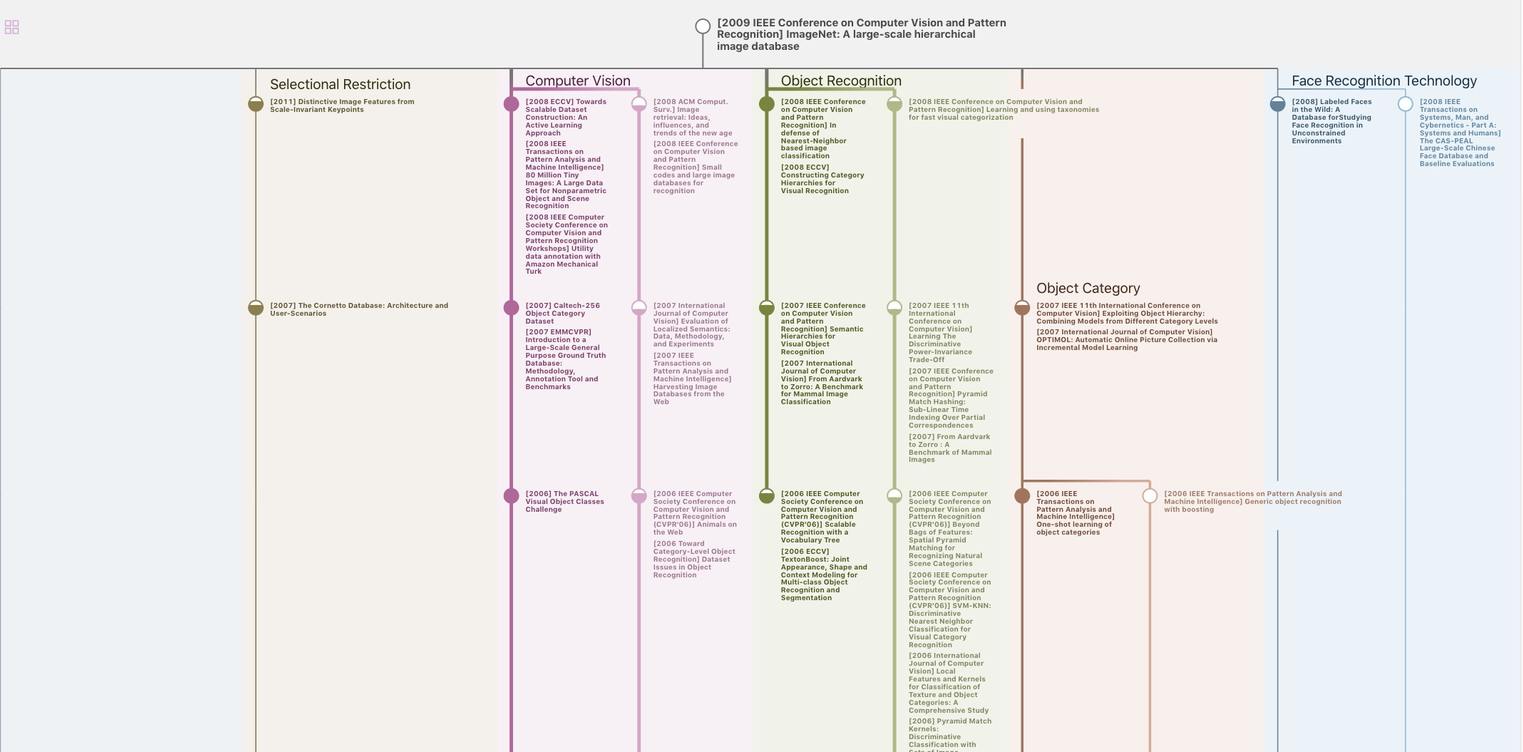
生成溯源树,研究论文发展脉络
Chat Paper
正在生成论文摘要