PolygraphMR: Enhancing the Reliability and Dependability of CNNs
2020 50th Annual IEEE/IFIP International Conference on Dependable Systems and Networks (DSN)(2020)
摘要
Deep neural networks (DNNs) are now starting to emerge in mission critical applications including autonomous vehicles and precision medicine. An important question is the dependability of DNNs and trustworthiness of their predictions. Considering the irreparable damage that can be caused by mispredictions, assessment of their potential misbehavior is necessary for safe deployment. In this paper, we first show the deficiency of current confidence-based methods as reliability measurement, and assess the effectiveness of traditional architecture reliability methods such as modular redundancy (MR). Then, we propose PolygraphMR and show that the combination of input preprocessing, smarter decision policies, and inclusion of prediction confidences can substantially improve the effectiveness of MR for DNNs. Next, we show how to prohibit explosive growth in the cost of MR by the help of reduced-precision designs and staged activations. Across six benchmarks, PolygraphMR detects an average of 33.5% of the baseline mispredictions with less than 2x overhead.
更多查看译文
关键词
Reliability, Machine vision, Computer performance
AI 理解论文
溯源树
样例
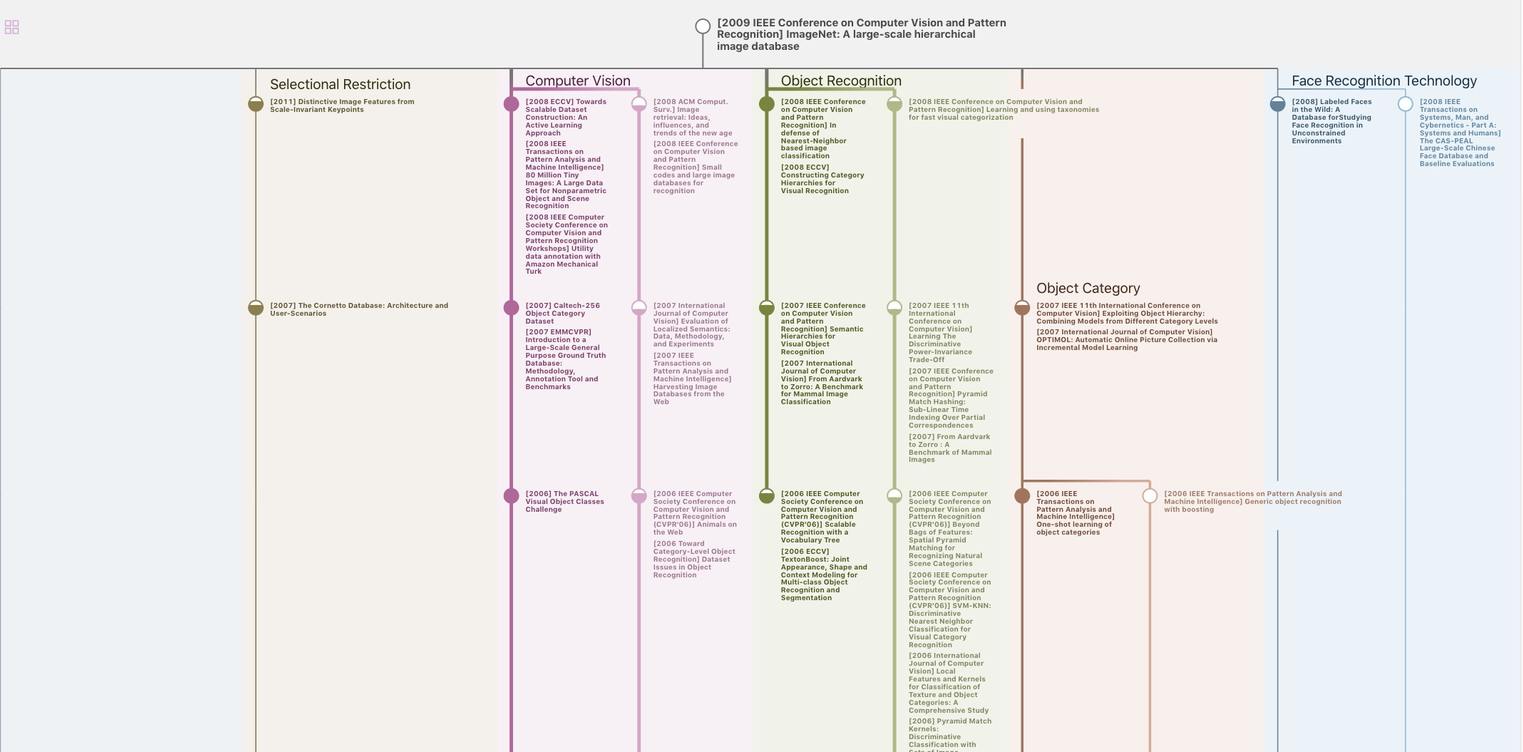
生成溯源树,研究论文发展脉络
Chat Paper
正在生成论文摘要