Multiperspective Automotive Labeling
2020 IEEE 34TH INTERNATIONAL PARALLEL AND DISTRIBUTED PROCESSING SYMPOSIUM WORKSHOPS (IPDPSW 2020)(2020)
摘要
Supervised machine learning techniques inherently rely on datasets to be trained. With image datasets traditionally being annotated by humans, many advancements in image annotation tools have been made to ensure creation of rich datasets with accurate labels. Nevertheless, users still find it challenging to create and use their own datasets with labels that reflect their problem domain. We propose a streamlined labeling process that aligns multiperspective images and allows a transition from a labeled perspective to other perspectives. The main goal of this work is to reduce the human effort required for labeling vehicle images under favorable conditions where the image perspectives are correlated and one or more perspectives are known. A case study is described and analyzed to show the effectiveness of the process, as well as constraints and limitations when applied to other cases.
更多查看译文
关键词
training dataset, multiperspective images, supervised machine learning, automotive labeling, supervised learning
AI 理解论文
溯源树
样例
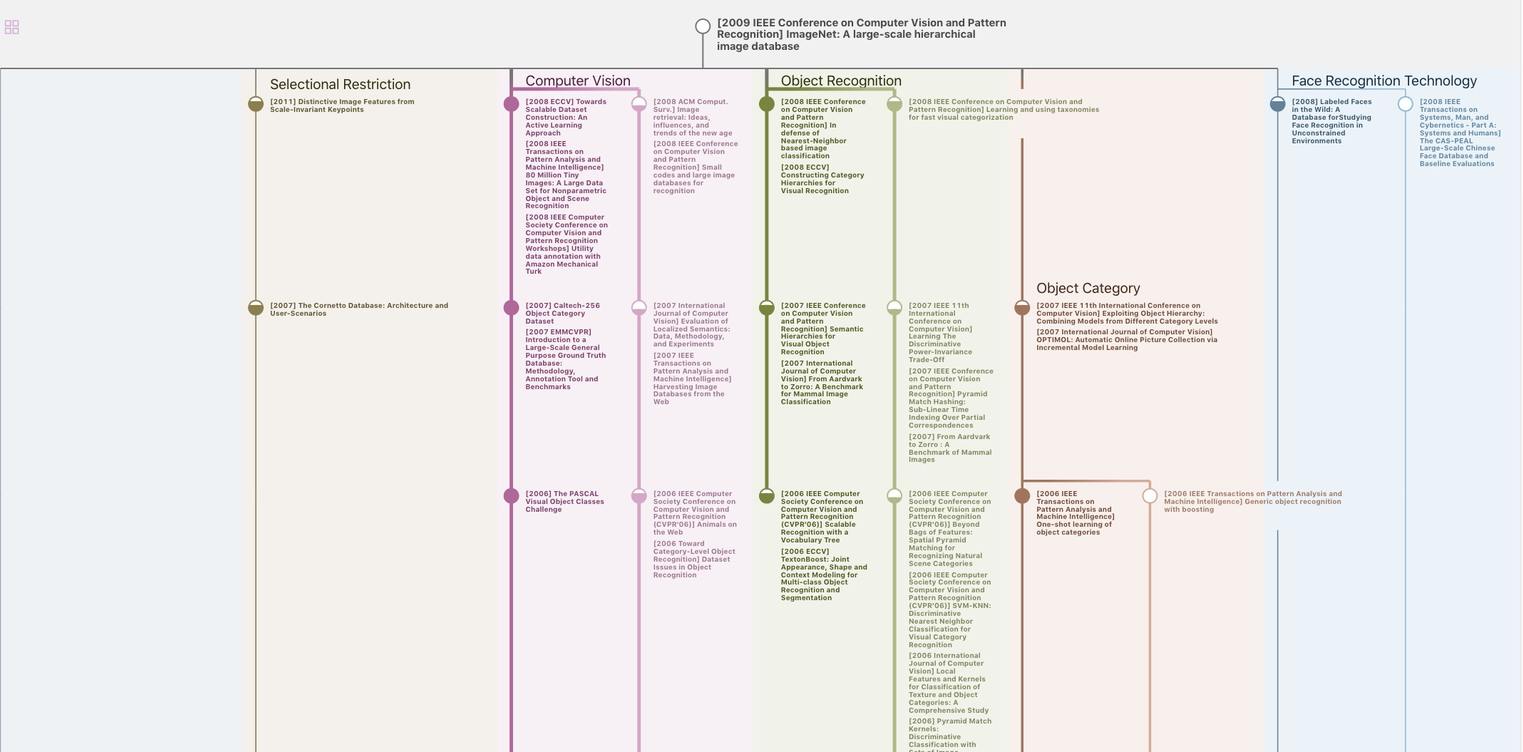
生成溯源树,研究论文发展脉络
Chat Paper
正在生成论文摘要