Learning Context-Adaptive Task Constraints For Robotic Manipulation
ROBOTICS AND AUTONOMOUS SYSTEMS(2021)
摘要
Constraint-based control approaches offer a flexible way to specify robotic manipulation tasks and execute them on robots with many degrees of freedom. However, the specification of task constraints and their associated priorities usually requires a human-expert and often leads to tailor-made solutions for specific situations. This paper presents our recent efforts to automatically derive task constraints for a constraint-based robot controller from data and adapt them with respect to previously unseen situations (contexts). We use a programming-by-demonstration approach to generate training data in multiple variations (context changes) of a given task. From this data we learn a probabilistic model that maps context variables to task constraints and their respective soft task priorities. We evaluate our approach with 3 different dual-arm manipulation tasks on an industrial robot and show that it performs better than comparable approaches with respect to reproduction accuracy in previously unseen contexts. (C) 2021 Elsevier B.V. All rights reserved.
更多查看译文
关键词
Context-adaptive control, Constraint-based robot control, Programming-by-demonstration, Gaussian mixture regression, Dual-arm manipulation
AI 理解论文
溯源树
样例
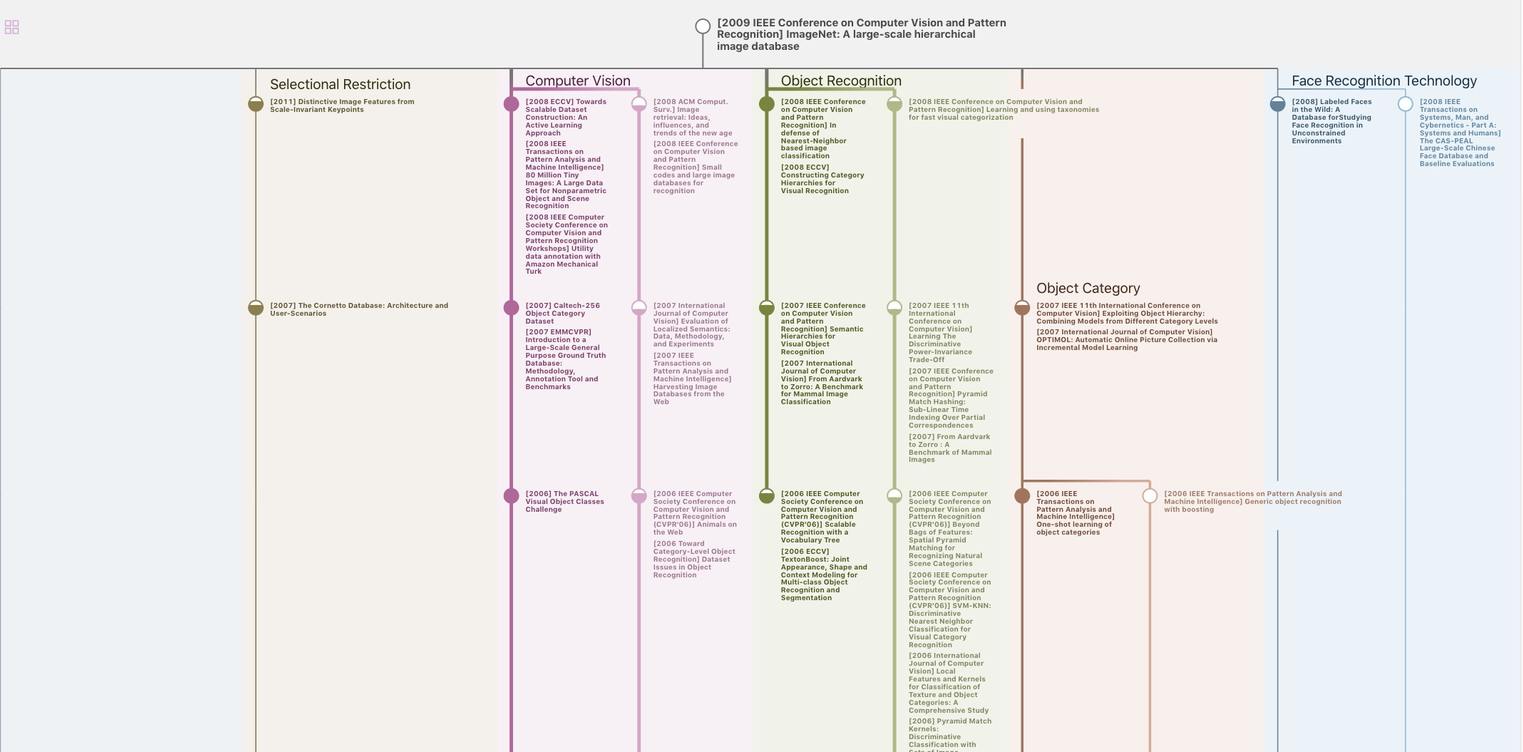
生成溯源树,研究论文发展脉络
Chat Paper
正在生成论文摘要