Structure Preserving Compressive Sensing MRI Reconstruction Using Generative Adversarial Networks.
2020 IEEE/CVF CONFERENCE ON COMPUTER VISION AND PATTERN RECOGNITION WORKSHOPS (CVPRW 2020)(2020)
摘要
Compressive sensing magnetic resonance imaging (CS-MRI) accelerates the acquisition of MR images by breaking the Nyquist sampling limit. In this work, a novel generative adversarial network (GAN) based framework for CS-MRI reconstruction is proposed. Leveraging a combination of patch-based discriminator and structural similarity index based loss, our model focuses on preserving high frequency content as well as fine textural details in the reconstructed image. Dense and residual connections have been incorporated in a U-net based generator architecture to allow easier transfer of information as well as variable network length. We show that our algorithm outperforms state-of-the-art methods in terms of quality of reconstruction and robustness to noise. Also, the reconstruction time, which is of the order of milliseconds, makes it highly suitable for real-time clinical use.
更多查看译文
关键词
U-net based generator architecture,reconstructed image,high frequency content,structural similarity index based loss,patch-based discriminator,CS-MRI reconstruction,novel generative adversarial network based framework,Nyquist sampling limit,MR images,compressive sensing magnetic resonance imaging,generative adversarial networks,structure preserving compressive sensing MRI reconstruction
AI 理解论文
溯源树
样例
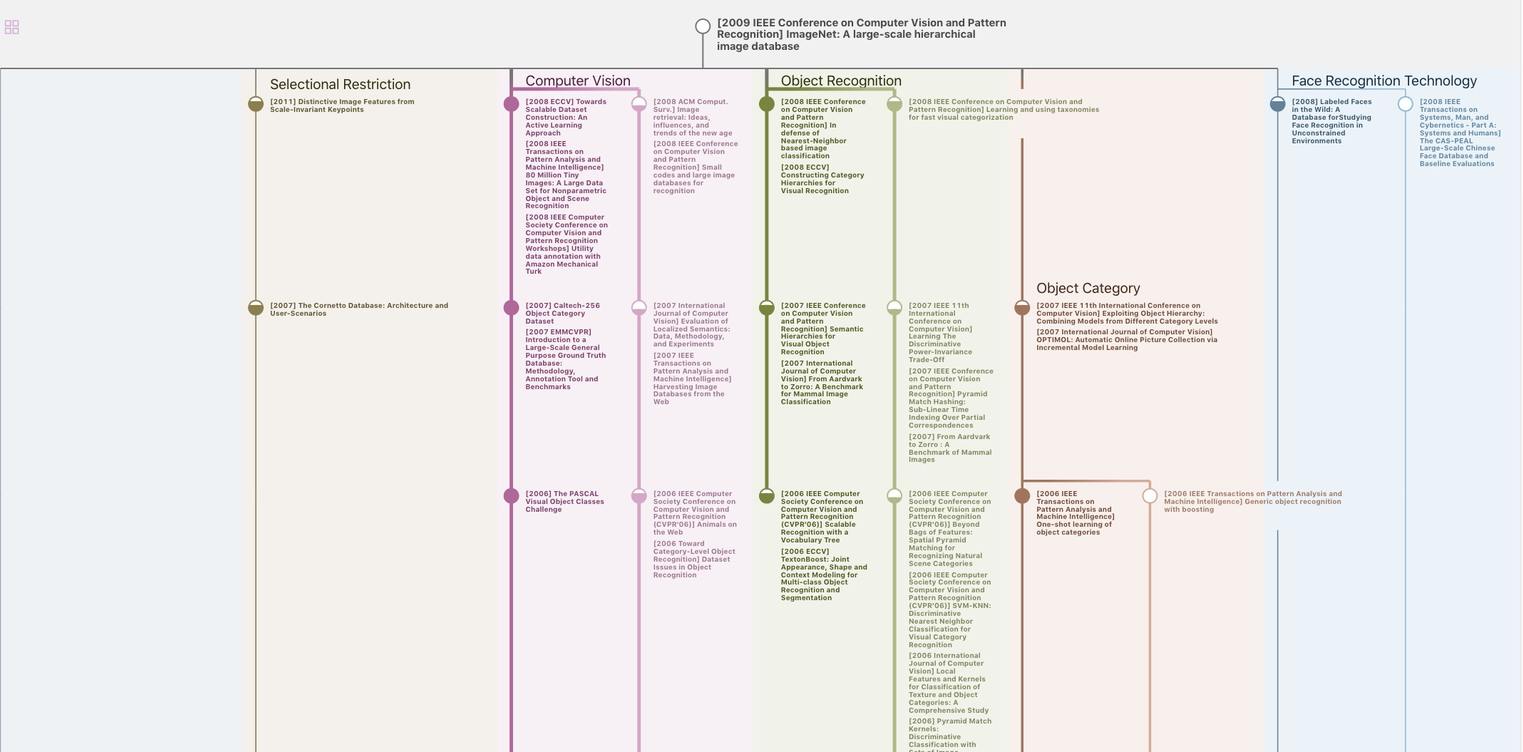
生成溯源树,研究论文发展脉络
Chat Paper
正在生成论文摘要