HeartTrack - Convolutional neural network for remote video-based heart rate monitoring.
CVPR Workshops(2020)
摘要
Detection and continuous monitoring of heart rate can help us identify clinical relevance of some cardiac symptoms. Over the last decade, a lot of attention has been paid to the development of the algorigthms for remote photoplethysmography (rPPG). As a result, we can now accurately monitor heart rate of still sitting subjects using data extracted from video feed. Aside from methods based on hand-crafted features, there have also been developed the more advanced learning-based rPPG algorithms. Deep learning methods usually require large amounts of data for training, however, biomedical data often suffers from lack of real-life data. To address these issues, we have developed a HeartTrack convolutional neural network for remote video-based heart rate tracking. This learning-based method has been trained on synthetic data to accurately estimate heart rate in different conditions. Moreover, here we provide two new rPPG datasets - MoLi-ppg-1 and MoLi-ppg-2 - that were recorded in complicated conditions that were close to the natural ones. The datasets include videos that feature moving and talking subjects, different types of lighting, various equipment, etc. We have used our new MoLi-ppg-1 and MoLi-ppg-2 datasets for algorithm training and testing, and the existing UBFC-RPPG dataset for the algorithm testing and comparison with other approaches. Our HeartTrack neural network shows state-of-the-art results on the UBFC-RPPG database (MAE=2.412, RMSE=3.368, R=0.983).
更多查看译文
关键词
rPPG datasets,MoLi-ppg-1 datasets,MoLi-ppg-2 datasets,remote video-based heart rate monitoring,continuous monitoring,remote photoplethysmography,video feed,hand-crafted features,advanced learning-based rPPG algorithms,biomedical data,real-life data,HeartTrack convolutional neural network,remote video-based heart rate tracking,learning-based method,synthetic data
AI 理解论文
溯源树
样例
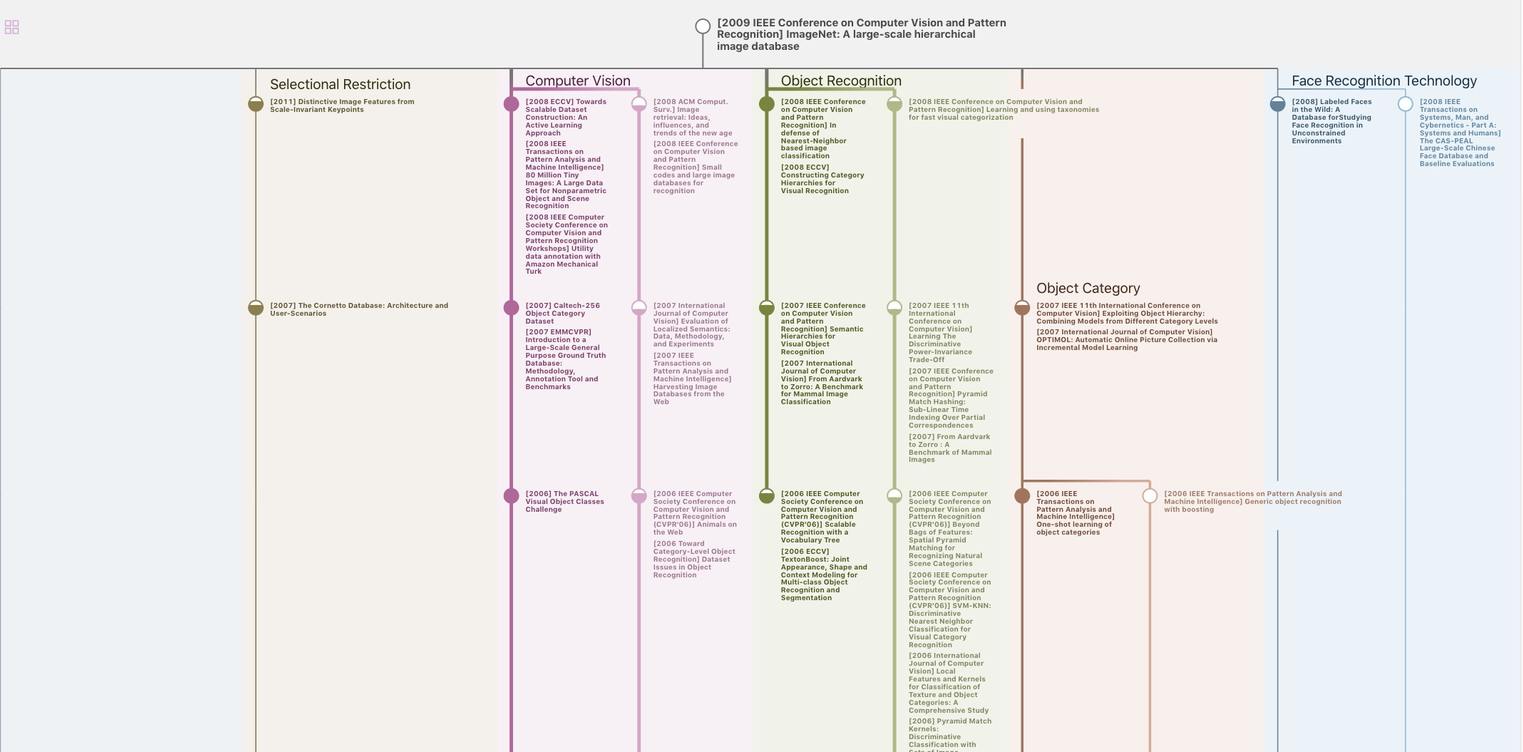
生成溯源树,研究论文发展脉络
Chat Paper
正在生成论文摘要