Benign Overfitting and Noisy Features
arxiv(2020)
摘要
Modern machine learning often operates in the regime where the number of parameters is much higher than the number of data points, with zero training loss and yet good generalization, thereby contradicting the classical bias-variance trade-off. This \textit{benign overfitting} phenomenon has recently been characterized using so called \textit{double descent} curves where the risk undergoes another descent (in addition to the classical U-shaped learning curve when the number of parameters is small) as we increase the number of parameters beyond a certain threshold. In this paper, we examine the conditions under which \textit{Benign Overfitting} occurs in the random feature (RF) models, i.e. in a two-layer neural network with fixed first layer weights. We adopt a new view of random feature and show that \textit{benign overfitting} arises due to the noise which resides in such features (the noise may already be present in the data and propagate to the features or it may be added by the user to the features directly) and plays an important implicit regularization role in the phenomenon.
更多查看译文
关键词
Double descent,Errors-in-variables,Kernel methods,Minimax learning rate,Random feature models
AI 理解论文
溯源树
样例
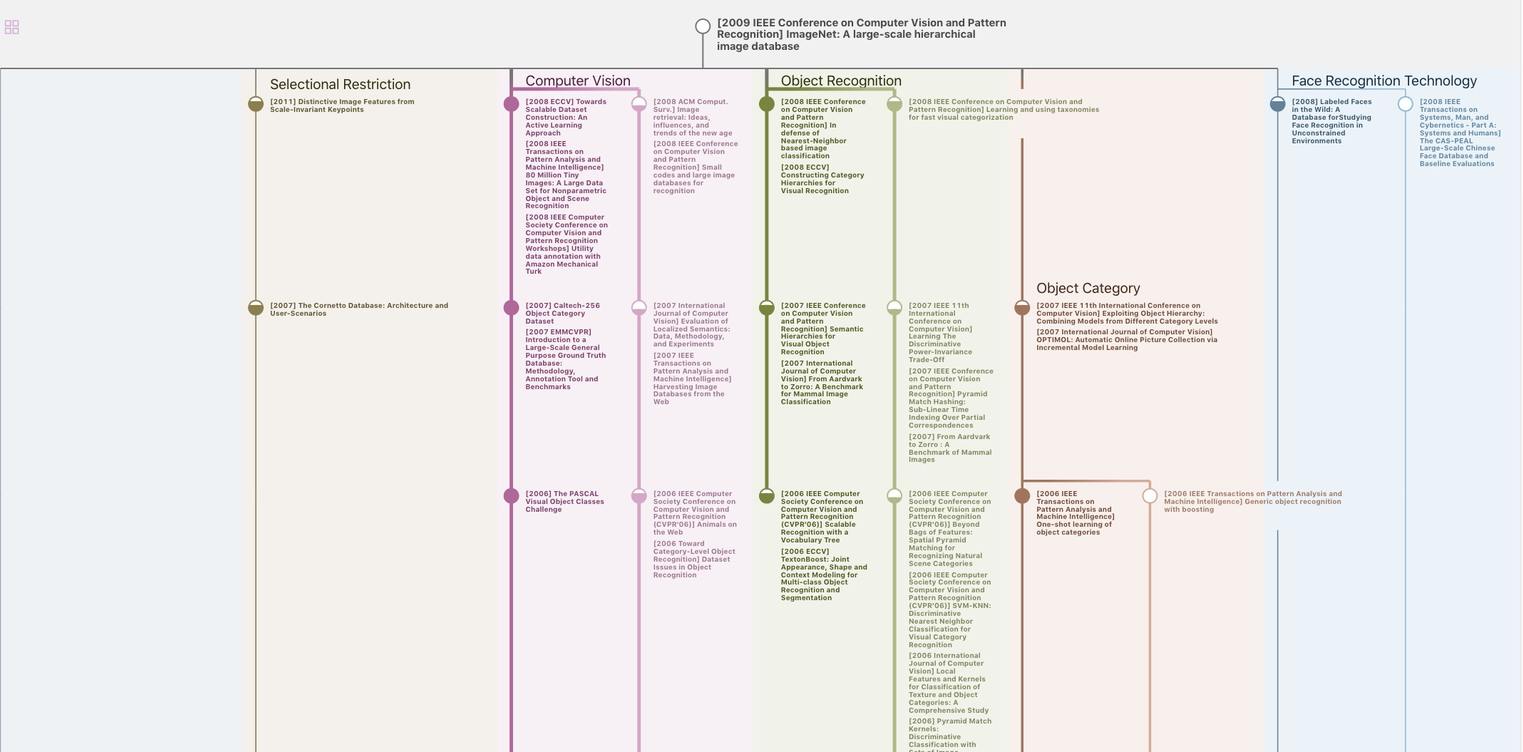
生成溯源树,研究论文发展脉络
Chat Paper
正在生成论文摘要