Hard Negative Samples Emphasis Tracker without Anchors
MM '20: The 28th ACM International Conference on Multimedia Seattle WA USA October, 2020(2020)
摘要
Trackers based on Siamese network have shown tremendous success, because of their balance between accuracy and speed. Nevertheless, with tracking scenarios becoming more and more sophisticated, most existing Siamese-based approaches ignore the addressing of the problem that distinguishes the tracking target from hard negative samples in the tracking phase. The features learned by these networks lack of discrimination, which significantly weakens the robustness of Siamese-based trackers and leads to suboptimal performance. To address this issue, we propose a simple yet efficient hard negative samples emphasis method, which constrains Siamese network to learn features that are aware of hard negative samples and enhance the discrimination of embedding features. Through a distance constraint, we force to shorten the distance between exemplar vector and positive vectors, meanwhile, enlarge the distance between exemplar vector and hard negative vectors. Furthermore, we explore a novel anchor-free tracking framework in a per-pixel prediction fashion, which can significantly reduce the number of hyper-parameters and simplify the tracking process by taking full advantage of the representation of convolutional neural network. Extensive experiments on six standard benchmark datasets demonstrate that the proposed method can perform favorable results against state-of-the-art approaches.
更多查看译文
关键词
Visual Tracking, Siamese Network, Hard Negative Samples, Anchor-free Tracker
AI 理解论文
溯源树
样例
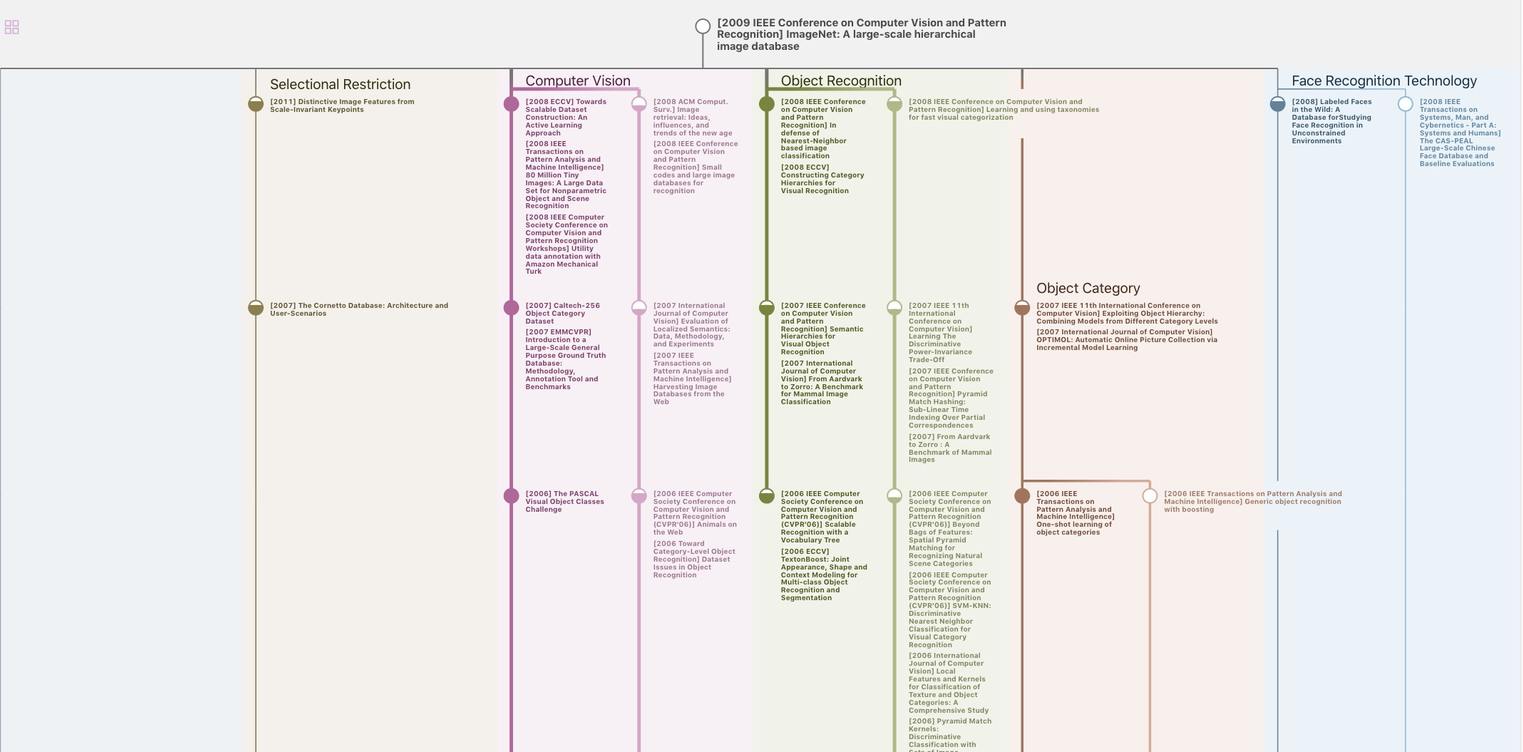
生成溯源树,研究论文发展脉络
Chat Paper
正在生成论文摘要