Extrapolating false alarm rates in automatic speaker verification
INTERSPEECH(2020)
摘要
Automatic speaker verification (ASV) vendors and corpus providers would both benefit from tools to reliably extrapolate performance metrics for large speaker populations without collecting new speakers. We address false alarm rate extrapolation under a worst-case model whereby an adversary identifies the closest impostor for a given target speaker from a large population. Our models are generative and allow sampling new speakers. The models are formulated in the ASV detection score space to facilitate analysis of arbitrary ASV systems.
更多查看译文
关键词
speaker verification, false alarm rate, closest impostor, black-box attack, PLDA, implicit generative models
AI 理解论文
溯源树
样例
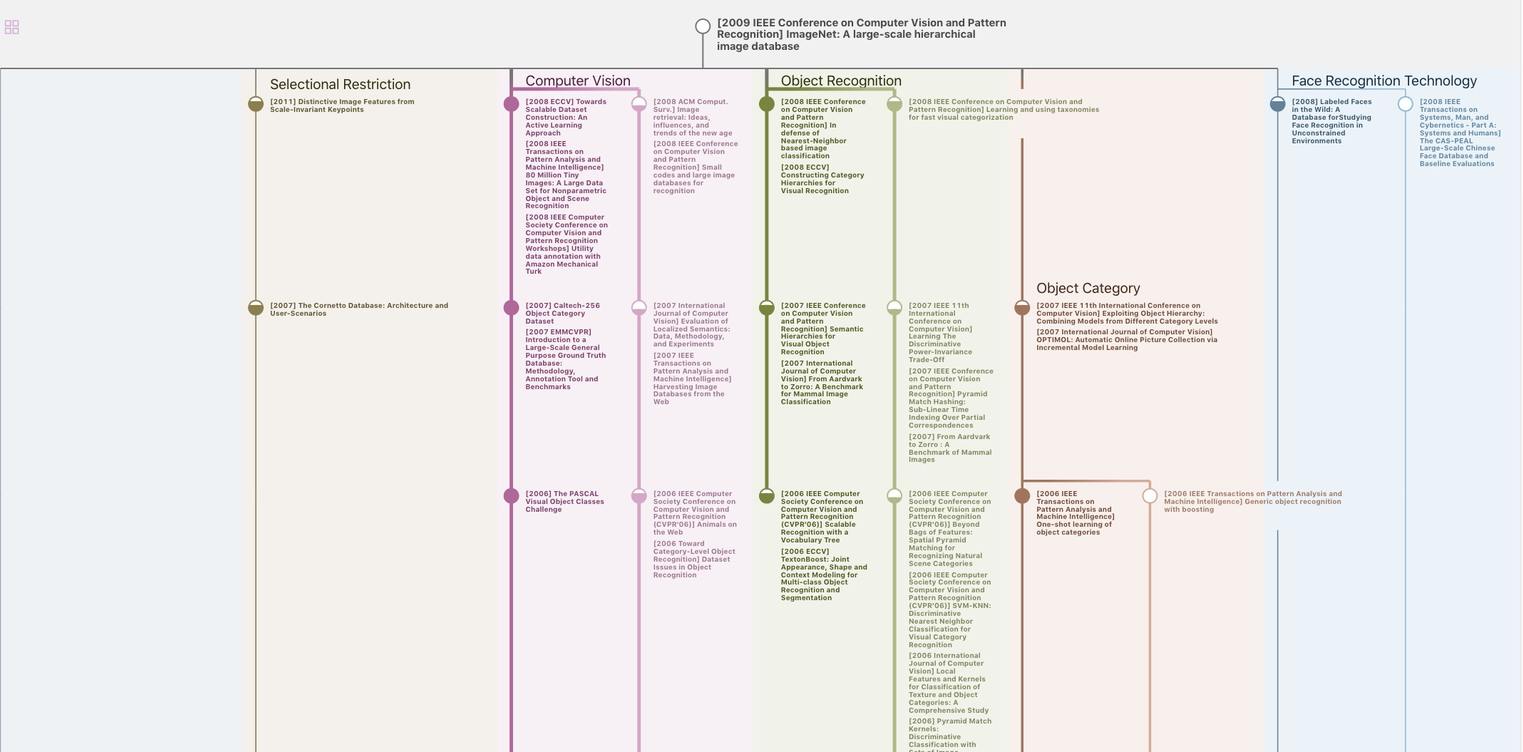
生成溯源树,研究论文发展脉络
Chat Paper
正在生成论文摘要