A New Spatial Count Data Model With Time-Varying Parameters
TRANSPORTATION RESEARCH PART B-METHODOLOGICAL(2021)
摘要
Recent crash frequency studies incorporate spatiotemporal correlations, but these studies have two key limitations - i) none of these studies accounts for temporal variation in model parameters; and ii) Gibbs sampler suffers from convergence issues due to non-conjugacy. To address the first limitation, we propose a new count data model that identifies the underlying temporal patterns of the regression parameters while simultaneously allowing for time-varying spatial correlation. The model is also extended to incorporate heterogeneity in non-temporal parameters across spatial units. We tackle the second shortcoming by deriving a Gibbs sampler that ensures conditionally conjugate posterior updates for all model parameters. To this end, we take the advantages of Polya-Gamma data augmentation and forward filtering backward sampling algorithm. After validating the properties of the Gibbs sampler in a Monte Carlo study, the advantages of the proposed specification are demonstrated in an empirical application to uncover relationships between crash frequency spanning across nine years and pavement characteristics. Model parameters exhibit practically significant temporal patterns (i.e., temporal instability). For example, the safety benefits of better pavement ride quality are estimated to increase over time.
更多查看译文
关键词
Negative-binomial regression, Dynamic linear models, Spatiotemporal dependence, Bayesian estimation, Polya-Gamma data augmentation
AI 理解论文
溯源树
样例
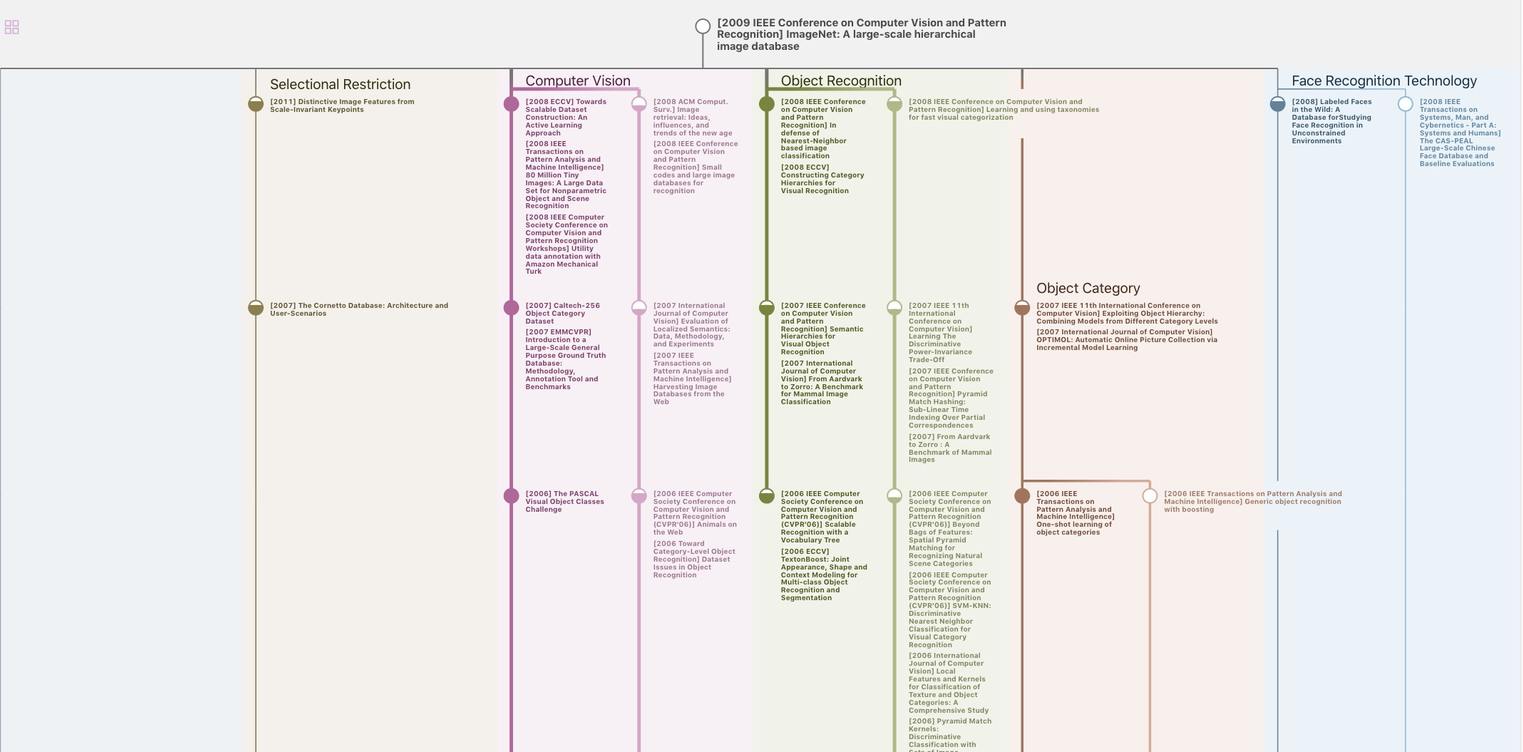
生成溯源树,研究论文发展脉络
Chat Paper
正在生成论文摘要