Cooperative Bi-path Metric for Few-shot Learning
MM '20: The 28th ACM International Conference on Multimedia Seattle WA USA October, 2020(2020)
摘要
Given base classes with sufficient labeled samples, the target of few-shot classification is to recognize unlabeled samples of novel classes with only a few labeled samples. Most existing methods only pay attention to the relationship between labeled and unlabeled samples of novel classes, which do not make full use of information within base classes. In this paper, we make two contributions to investigate the few-shot classification problem. First, we report a simple and effective baseline trained on base classes in the way of traditional supervised learning, which can achieve comparable results to the state of the art. Second, based on the baseline, we propose a cooperative bi-path metric for classification, which leverages the correlations between base classes and novel classes to further improve the accuracy. Experiments on two widely used benchmarks show that our method is a simple and effective framework, and a new state of the art is established in the few-shot classification field.
更多查看译文
关键词
learning,bi-path,few-shot
AI 理解论文
溯源树
样例
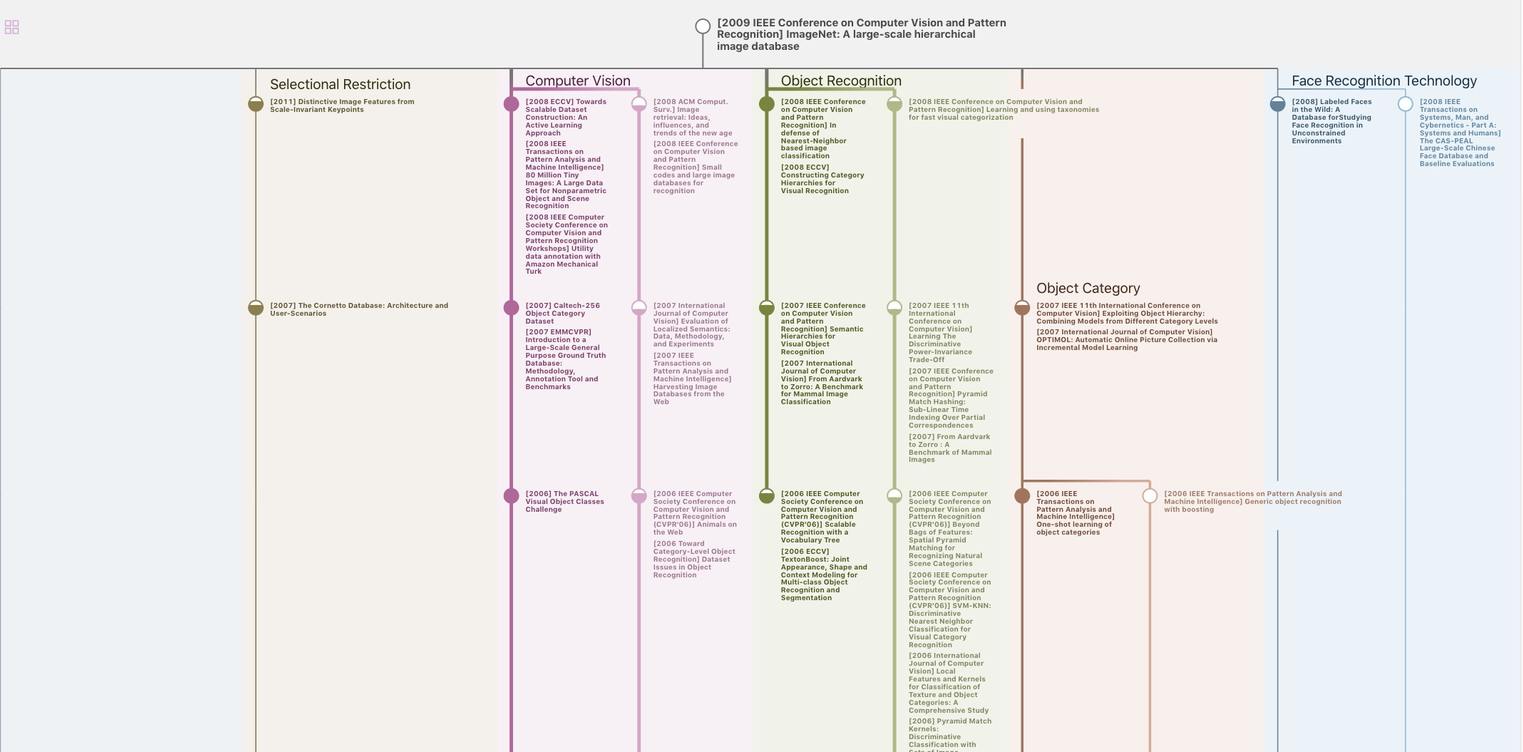
生成溯源树,研究论文发展脉络
Chat Paper
正在生成论文摘要