Hardware and software techniques for sparse deep neural networks
Hardware Architectures for Deep Learning(2020)
摘要
Over the past four decades, every generation of processors has delivered 2× performance boost, as predicted by Moore’s law [1]. Ironically, the end of Moore’s law occurred at almost the same time as computationally intensive deep learning algorithms were emerging. Deep neural networks (DNNs) offer state-of-the-art solutions for many applications, including computer vision, speech recognition, and natural language processing. However, this is just the tip of the iceberg. Deep learning is taking over many classic machine-learning applications and also creating new markets, such as autonomous vehicles, which will tremendously amplify the demand for even more computational power.Hardware specialization was an effective response to meet the computational demands by devising efficient hardware architectures rather than relying on transistors’ characteristics improvement. In the last decade, many startups have emerged to make specialized hardware accelerators only for running DNNs efficiently [2]. The market also welcomes these changes, eg, the sale of Application Specific Integrated Circuits, ASICs, and Fields Programmable Gate Arrays, FPGAs, in the global data centers for deep learning computation increased from almost 0% in 2016 to 25% in 2018 [3]. However, there is still a need to further accelerate the hardware for two main reasons. First, in the next decade, the rise of internet of things will significantly increase the number of smart devices and sensors on the edge and the service requirements in the cloud, and DNN algorithms are expected to be heavily employed in both. Second, the main incentive for hardware buyers is the …
更多查看译文
关键词
neural networks,hardware,software techniques
AI 理解论文
溯源树
样例
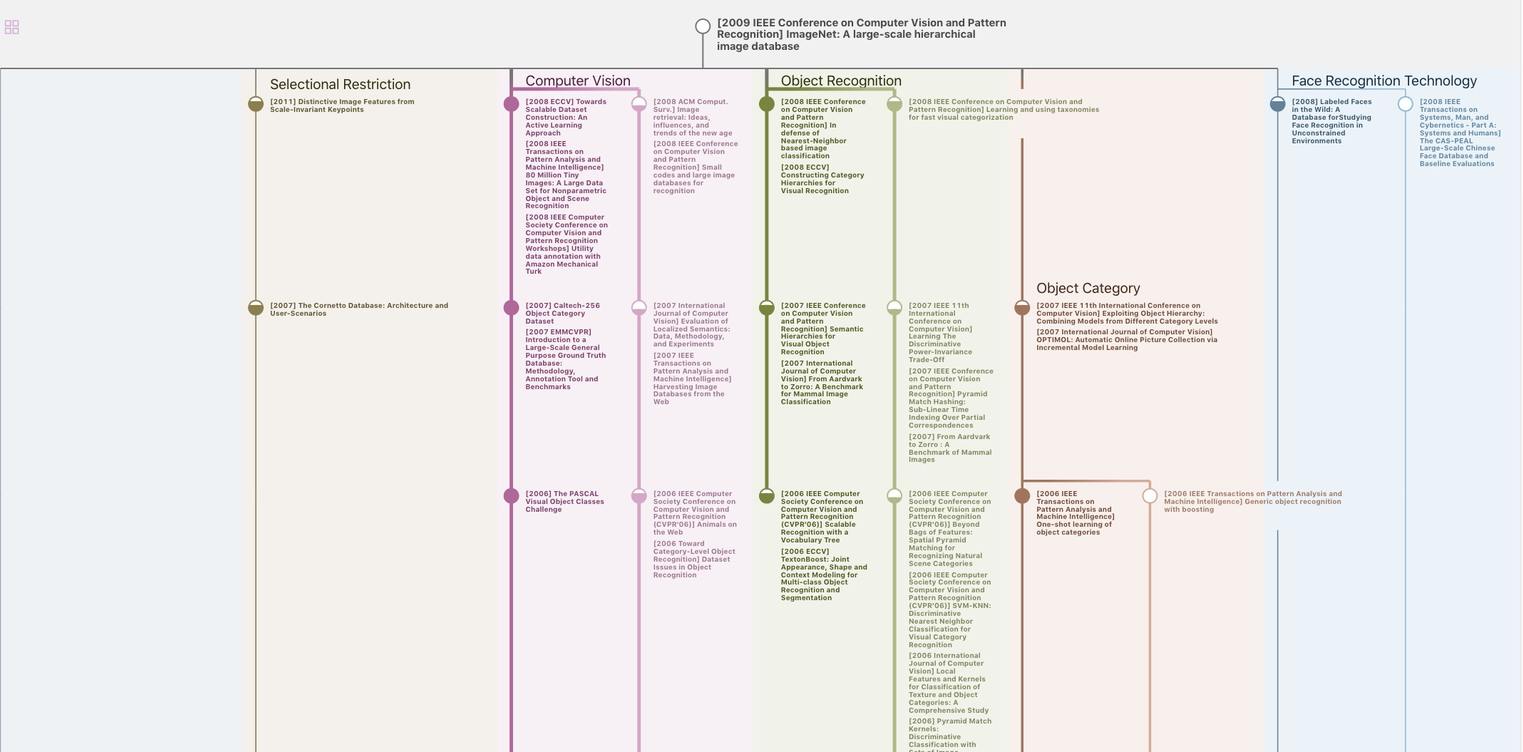
生成溯源树,研究论文发展脉络
Chat Paper
正在生成论文摘要