API-Net: Robust Generative Classifier via a Single Discriminator
ECCV(2020)
摘要
Robustness of deep neural network classifiers has been attracting increased attention. As for the robust classification problem, a generative classifier typically models the distribution of inputs and labels, and thus can better handle off-manifold examples at the cost of a concise structure. On the contrary, a discriminative classifier only models the conditional distribution of labels given inputs, but benefits from effective optimization owing to its succinct structure. This work aims for a solution of generative classifiers that can profit from the merits of both. To this end, we propose an Anti-Perturbation Inference (API) method, which searches for anti-perturbations to maximize the lower bound of the joint log-likelihood of inputs and classes. By leveraging the lower bound to approximate Bayes’ rule, we construct a generative classifier Anti-Perturbation Inference Net (API-Net) upon a single discriminator. It takes advantage of the generative properties to tackle off-manifold examples while maintaining a succinct structure for effective optimization. Experiments show that API successfully neutralizes adversarial perturbations, and API-Net consistently outperforms state-of-the-art defenses on prevailing benchmarks, including CIFAR-10, MNIST, and SVHN.(Our code is available at github.com/dongxinshuai/API-Net.).
更多查看译文
关键词
Deep learning,Neural networks,Adversarial defense,Adversarial training,Generative classifier
AI 理解论文
溯源树
样例
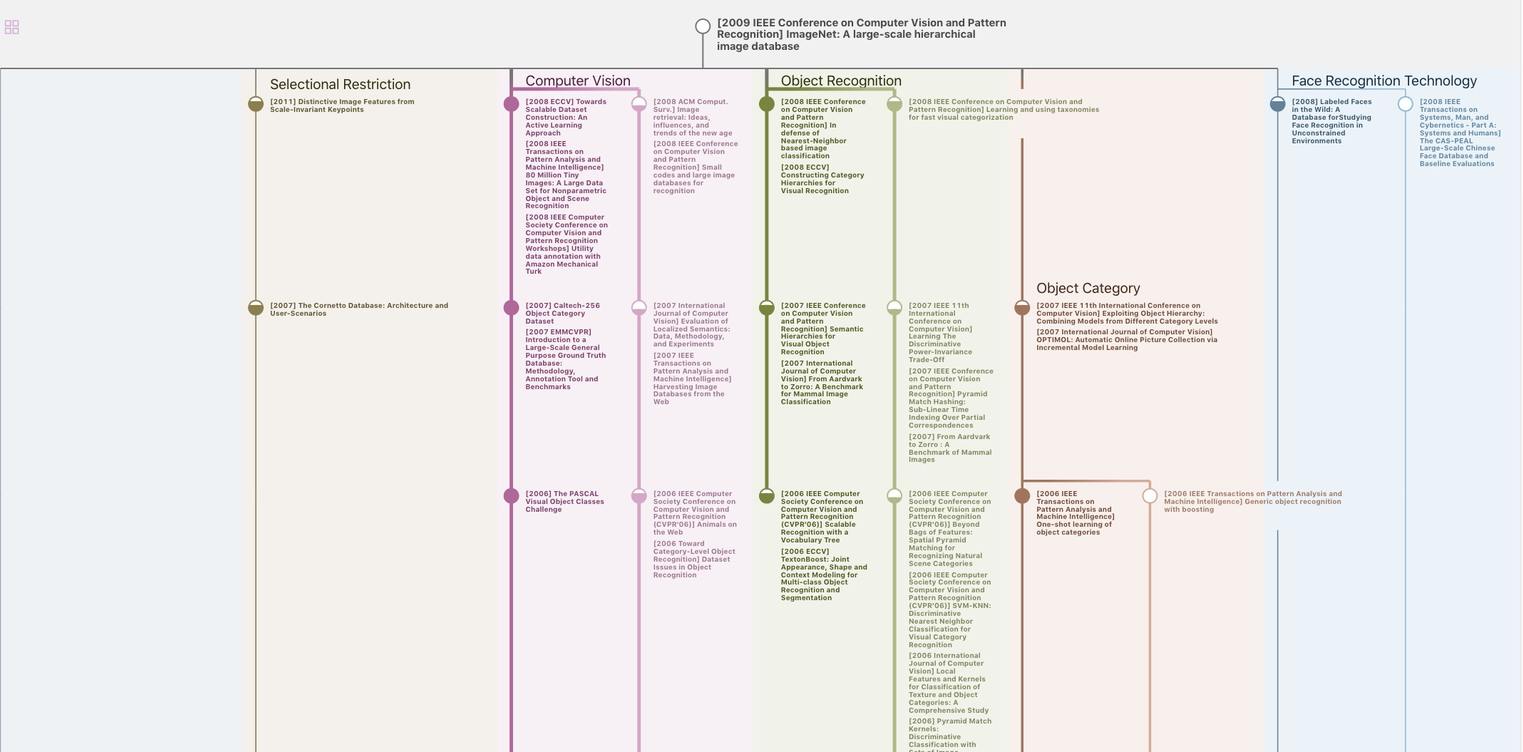
生成溯源树,研究论文发展脉络
Chat Paper
正在生成论文摘要