Parameter Identification and Assessment of Independence in Multivariate Statistical Modeling
user-5ef9583f4c775ed682ecca0f(2018)
摘要
We are interested in the extent to which, possibly causal, relationships can be statistically quantified from multivariate data obtained from a system of random variables. In the ideal setting, we would begin with refined knowledge of which variables in our system can causally impact one another and be in the position to perform randomized controlled experiments where any intervention is possible. Unfortunately this ideal is often unrealistic: in many important cases it is impossible to conduct an intervention, we cannot ethically ask a pregnant mother to start smoking or feasibly assign a country a new governmental system, and, additionally, a researcher may have little or no prior knowledge of how their system of variables interact. This dissertation studies two problems that arise as we depart from the experimental ideal. While scientists may not always be able to conduct a controlled experiment, thus only having observational data, they may they may be able to hypothesize or determine the directions in which causal relations point. For instance, ``mother smoking during first trimester of pregnancy'' may causally impact ``baby birth weight'' but, without time travel, certainly the reverse is impossible. Unfortunately causal relationships can be infeasible to estimate from observational data due to the presence of hidden confounding variables. In a more recent shift of paradigm, pioneered by researchers such as Judea Pearl, Jamie Robins, Don Rubin, and Peter Spirtes, causal knowledge is represented by a directed graph whose vertices are the variables in the system. These directed graphs have a corresponding mathematical formalism called structural …
更多查看译文
AI 理解论文
溯源树
样例
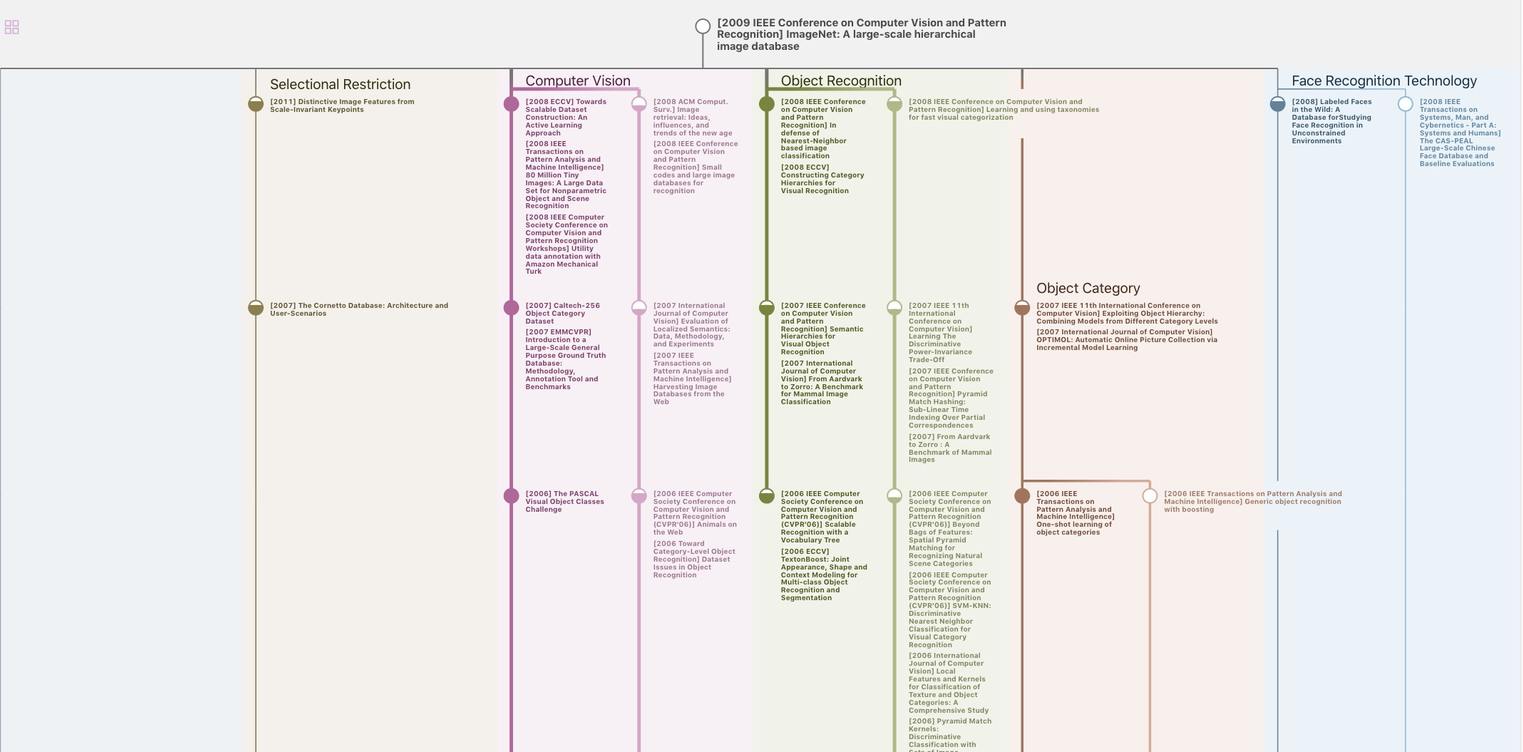
生成溯源树,研究论文发展脉络
Chat Paper
正在生成论文摘要