Semi-supervised Sentiment Analysis for Chinese Stock Texts in Scarce Labeled Data Scenario and Price Prediction.
CCIR(2020)
摘要
The application of neural network in stock prediction is developing rapidly these years because of its excellency in series data processing. However, as most of research are conducted in English, data sources and labeled data are inadequate in Chinese. Especially for natural language processing tasks in specific domain where specialized labeled data are required to train models to adapt to terminology processing, specialized labeled Chinese in text data are very scarce, such as financial text data. To tackle this challenge, we proposed a semi-supervised learning method to generate well-labeled data and train BERT, a leading natural language processing model, to obtain a trained sentiment machine. Then we got stock-related text data sentiment score based on this machine and further combine the sentiment score and other transaction data as inputs for different neural networks to predict stock price. The experimental results on a large scale of Chinese stock data and texts showed that our proposed method successfully improved prediction accuracy compared to other established methods. Besides, we also examined our method’s applicability combined with different neural networks when predicting different types of stock.
更多查看译文
AI 理解论文
溯源树
样例
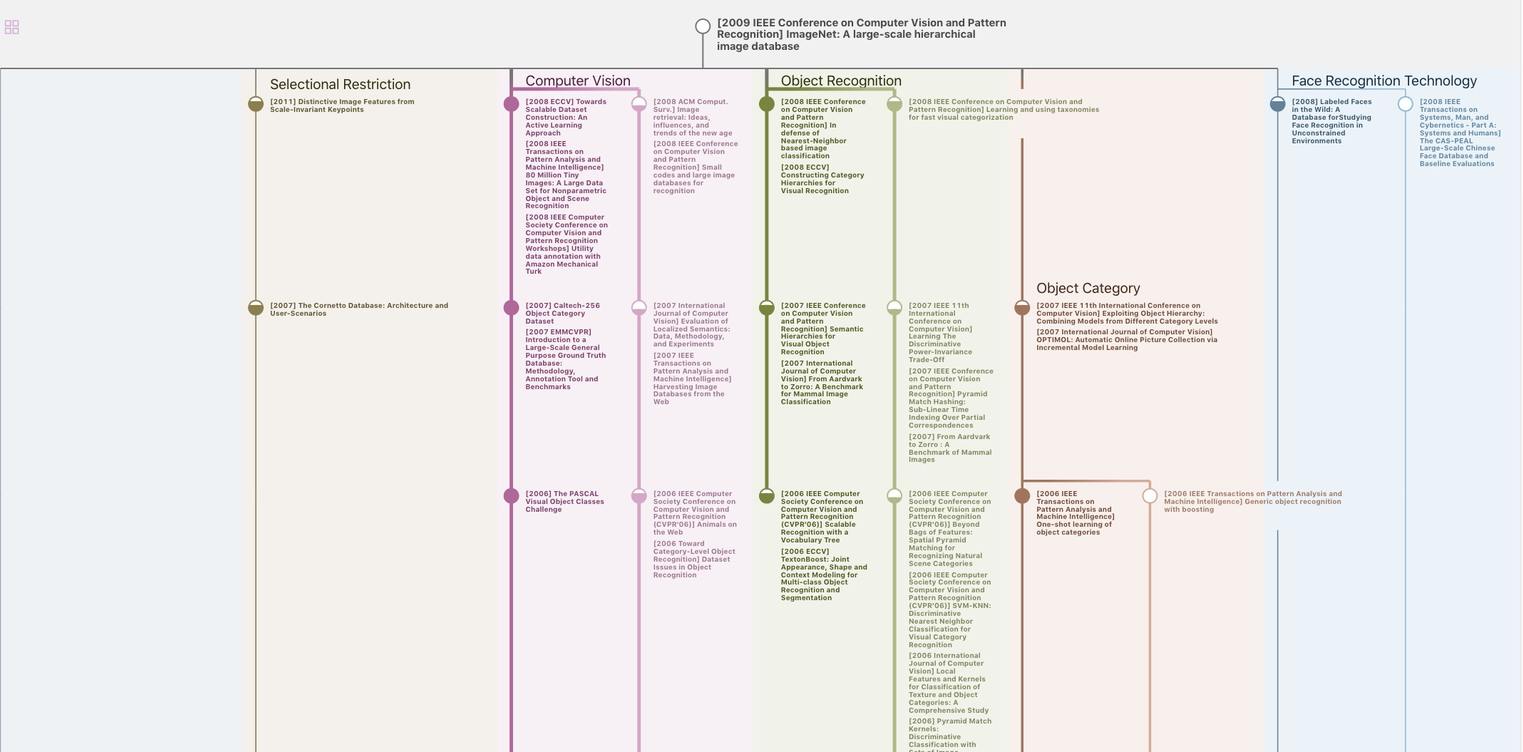
生成溯源树,研究论文发展脉络
Chat Paper
正在生成论文摘要