Tracking the State of Large Dynamic Networks Via Reinforcement Learning
IEEE Conference on Computer Communications(2020)
摘要
A Network Inventory Manager (NIM) is a software solution that scans, processes and records data about all devices in a network. We consider the problem faced by a NIM that can send out a limited number of probes to track changes in a large, dynamic network. The underlying change rate for the Network Elements (NEs) is unknown and may be highly non-uniform. The NIM should concentrate its probe budget on the NEs that change most frequently with the ultimate goal of minimizing the weighted Fraction of Stale Time (wFOST) of the inventory. However, the NIM cannot discover the change rate of a NE unless the NE is repeatedly probed.We develop and analyze two algorithms based on Reinforcement Learning to solve this exploration-vs-exploitation problem. The first is motivated by the Thompson Sampling method and the second is derived from the Robbins-Monro stochastic learning paradigm. We show that for a fixed probe budget, both of these algorithms produce a potentially unbounded improvement in terms of wFOST compared to the baseline algorithm that divides the probe budget equally between all NEs. Our simulations of practical scenarios show optimal performance in minimizing wFOST while discovering the change rate of the NEs.
更多查看译文
关键词
NIM,software solution,records data,dynamic network,underlying change rate,Network Elements,NE,nonuniform,Stale Time,wFOST,Reinforcement Learning,exploration-vs-exploitation problem,fixed probe budget,Network Inventory Manager
AI 理解论文
溯源树
样例
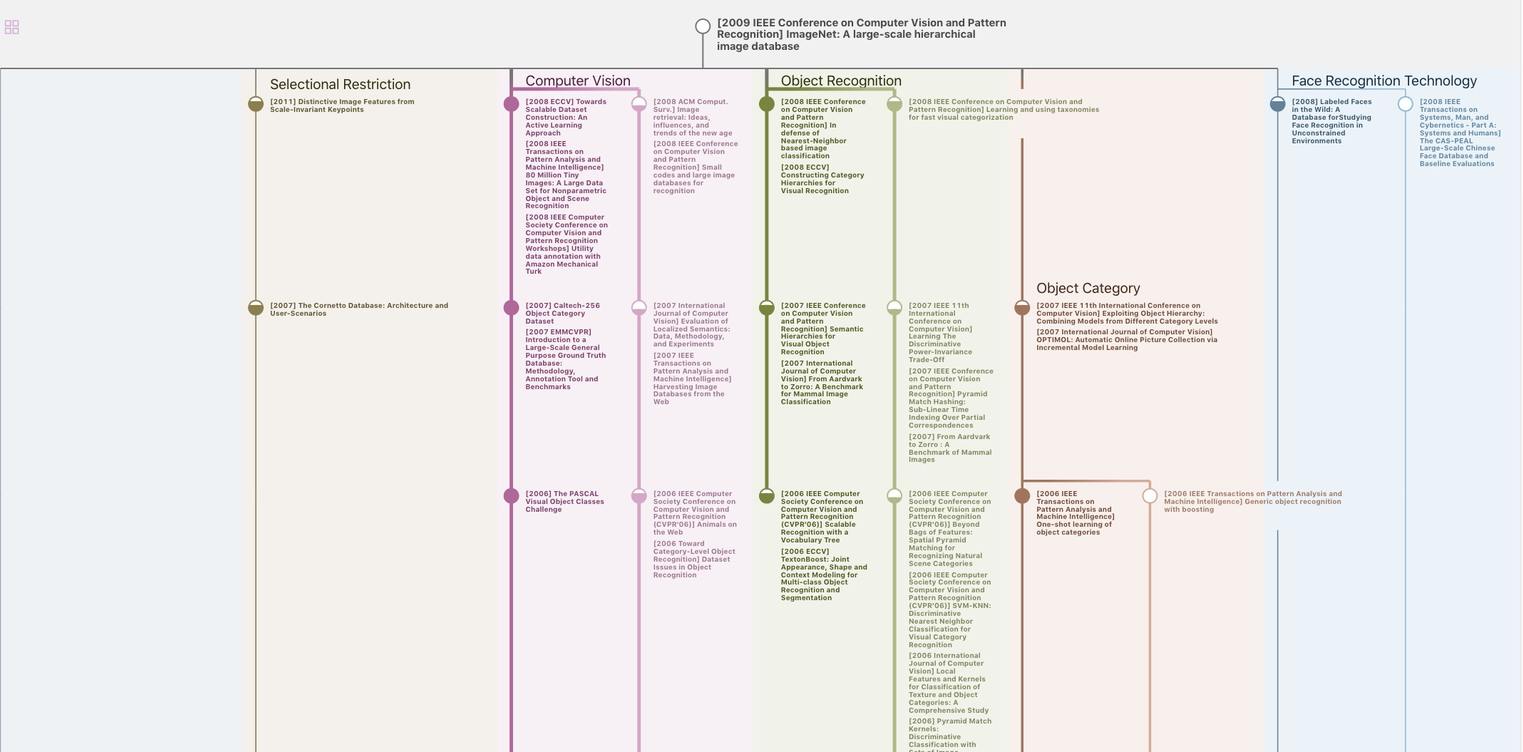
生成溯源树,研究论文发展脉络
Chat Paper
正在生成论文摘要