Decentralized Placement Of Data And Analytics In Wireless Networks For Energy-Efficient Execution
IEEE INFOCOM 2020 - IEEE CONFERENCE ON COMPUTER COMMUNICATIONS(2020)
摘要
We address energy-efficient placement of data and analytics components of composite analytics services on a wireless network to minimize execution-time energy consumption (computation and communication) subject to compute, storage and network resource constraints.We introduce an expressive analytics service hypergraph model for representing k-ary composability relationships (k >= 2) between various analytics and data components and leverage binary quadratic programming (BQP) to minimize the total energy consumption of a given placement of the analytics hypergraph nodes on the network subject to resource availability constraints. Then, after defining a potential energy functional phi(center dot) to model the affinities of analytics components and network resources using analogs of attractive and repulsive forces in physics, we propose a decentralized Metropolis Monte Carlo (MMC) sampling method which seeks to minimize Phi by moving analytics and data on the network. Although Phi is non-convex, using a potential game formulation, we identify conditions under which the algorithm provably converges to a local minimum energy equilibrium placement configuration.Trace-based simulations of the placement of a deep-neural-network analytics service on a realistic wireless network show that for smaller problem instances our MMC algorithm yields placements with total energy within a small factor of BQP and more balanced workload distributions; for larger problems, it yields low-energy configurations while the BQP approach fails.
更多查看译文
关键词
low-energy configurations,smaller problem instances our MMC algorithm yields placements,realistic wireless network show,deep-neural-network analytics service,local minimum energy equilibrium placement configuration,decentralized Metropolis Monte Carlo sampling method,network resources,potential energy,resource availability constraints,network subject,analytics hypergraph nodes,given placement,total energy consumption,leverage binary quadratic programming,data components,k-ary composability relationships,expressive analytics service hypergraph model,composite analytics services,analytics components,energy-efficient placement,energy-efficient execution,decentralized placement
AI 理解论文
溯源树
样例
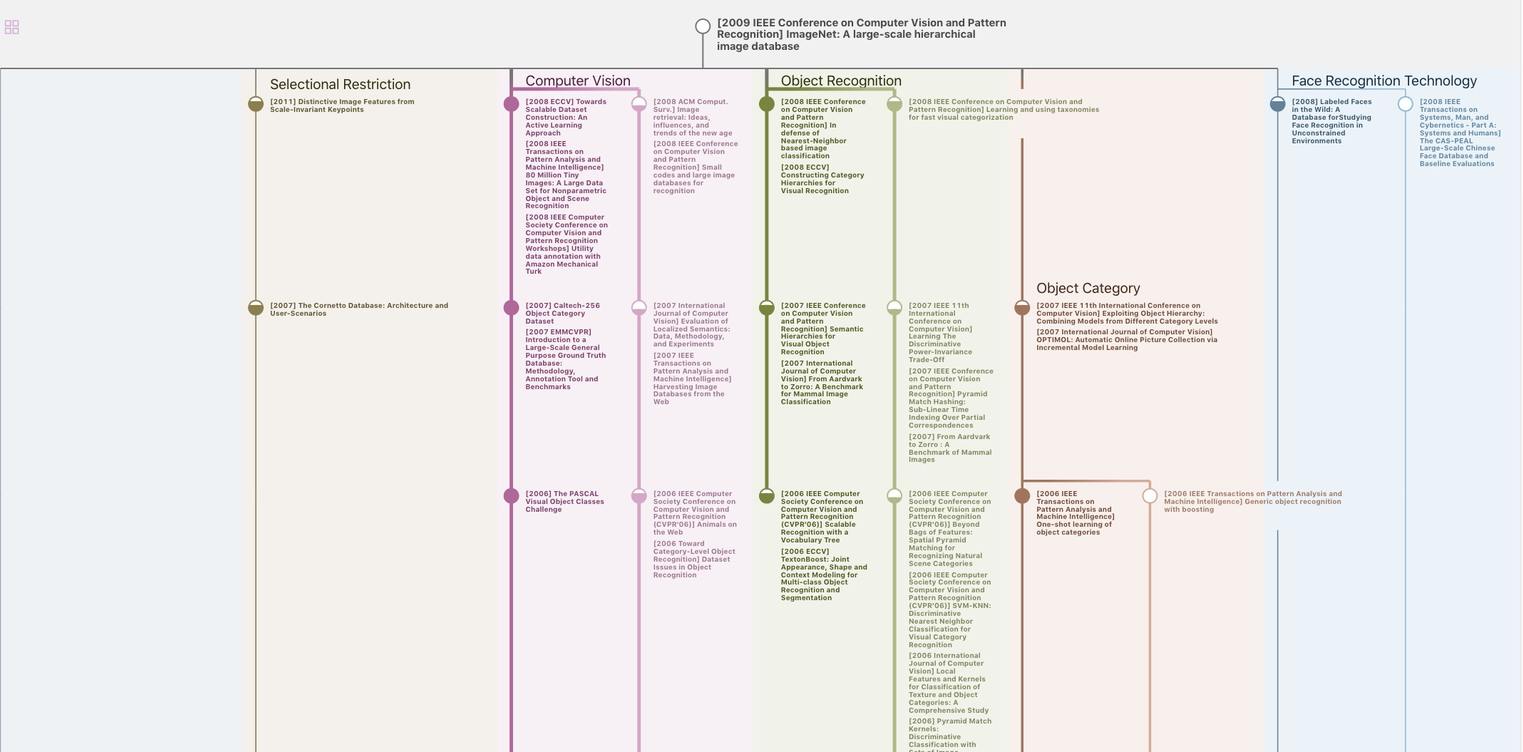
生成溯源树,研究论文发展脉络
Chat Paper
正在生成论文摘要