Stick: A Harmonious Fusion of Buffer-based and Learning-based Approach for Adaptive Streaming
IEEE Conference on Computer Communications(2020)
摘要
Off-the-shelf buffer-based approaches leverage a simple yet effective buffer-bound to control the adaptive bitrate (ABR) streaming system. Nevertheless, such approaches in standard parameters fail to always provide high quality of experience (QoE) video streaming services under all considered network conditions. Meanwhile, state-of-the-art learning-based ABR approach Pensieve outperforms existing schemes but is impractical to deploy. Therefore, how to harmoniously fuse the buffer-based and learning-based approach has become a key challenge for further enhancing ABR methods. In this paper, we propose Stick, an ABR algorithm that fuses the deep learning method and traditional buffer-based method. Stick utilizes the deep reinforcement learning (DRL) method to train the neural network, which outputs the buffer-bound to control the buffer-based approach for maximizing the QoE metric with different parameters. Trace-driven emulation illustrates that Stick betters Pensieve by 3.5% - 9.41% with an overhead reduction of 88%. Moreover, aiming to further reduce the computational costs while preserving the performances, we propose Trigger, a light-weighted neural network that determines whether the buffer-bound should be adjusted. Experimental results show that Stick+Trigger rivals or outperforms existing schemes in average QoE by 1.7%-28%, and significantly reduces the Stick’s computational overhead by 24%-61%. Meanwhile, we show that Trigger also helps other ABR schemes mitigate the overhead. Extensive results on real-world evaluation demonstrate the superiority of Stick over existing state-of-the-art approaches.
更多查看译文
关键词
existing state-of-the-art approaches,ABR schemes,Stick's computational overhead,Stick+Trigger rivals,light-weighted neural network,Stick betters Pensieve,buffer-based approach,deep reinforcement learning method,traditional buffer-based method,deep learning method,ABR algorithm,enhancing ABR methods,state-of-the-art learning-based ABR approach Pensieve,considered network conditions,experience video streaming services,adaptive bitrate streaming system,simple yet effective buffer-bound,off-the-shelf buffer-based approaches leverage,adaptive streaming,harmonious fusion
AI 理解论文
溯源树
样例
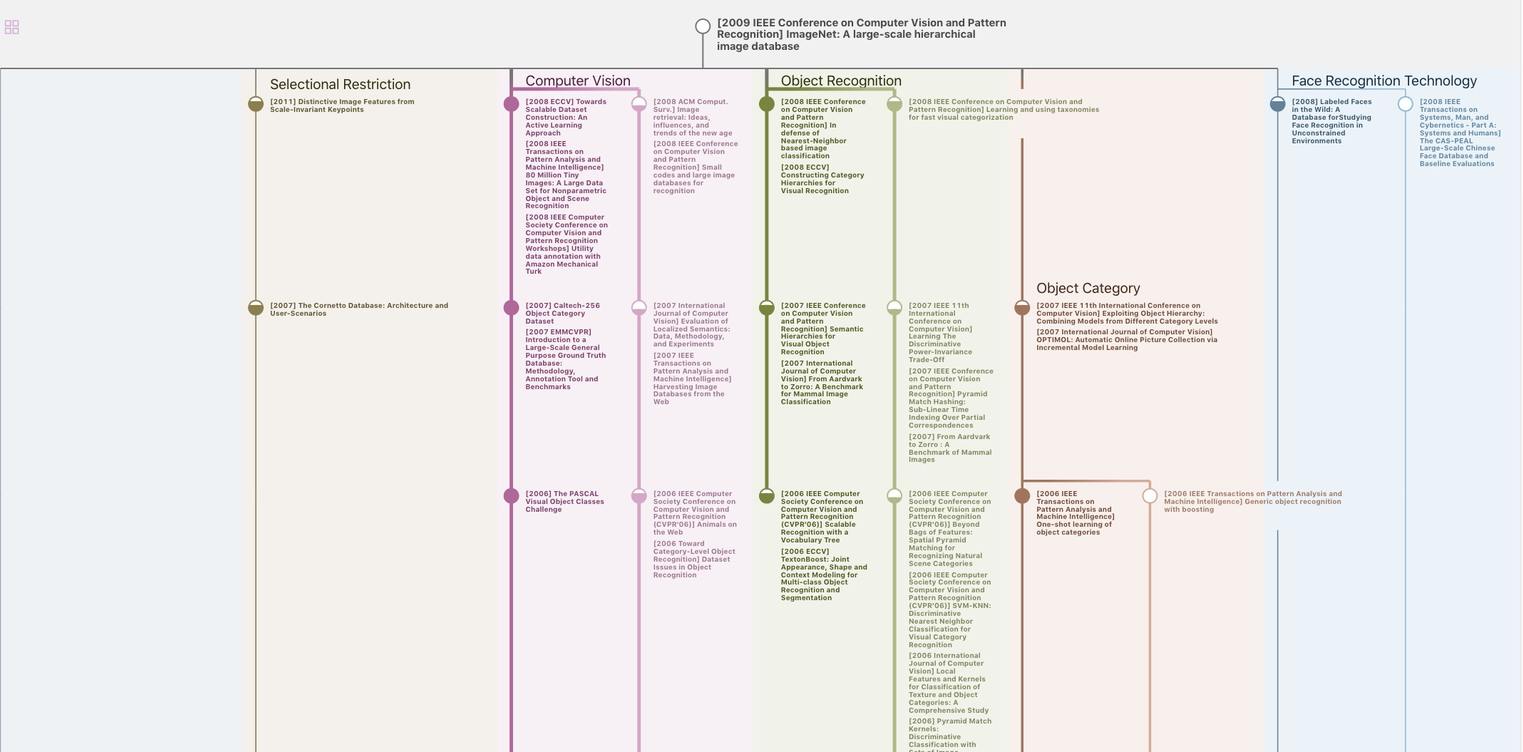
生成溯源树,研究论文发展脉络
Chat Paper
正在生成论文摘要