Energy-Efficient UAV Crowdsensing with Multiple Charging Stations by Deep Learning
IEEE INFOCOM 2020 - IEEE Conference on Computer Communications(2020)
摘要
Different from using human-centric mobile devices like smartphones, unmanned aerial vehicles (UAVs) can be utilized to form a new UAV crowdsensing paradigm, where UAVs are equipped with build-in high-precision sensors, to provide data collection services especially for emergency situations like earthquakes or flooding. In this paper, we aim to propose a new deep learning based framework to tackle the problem that a group of UAVs energy-efficiently and cooperatively collect data from low-level sensors, while charging the battery from multiple randomly deployed charging stations. Specifically, we propose a new deep model called "j-PPO+ConvNTM" which contains a novel spatiotemporal module "Convolution Neural Turing Machine" (ConvNTM) to better model long-sequence spatiotemporal data, and a deep reinforcement learning (DRL) model called "j-PPO", where it has the capability to make continuous (i.e., route planing) and discrete (i.e., either to collect data or go for charging) action decisions simultaneously for all UAVs. Finally, we perform extensive simulation to show its illustrative movement trajectories, hyperparameter tuning, ablation study, and compare with four other baselines.
更多查看译文
关键词
UAV crowdsensing,spatiotemporal modeling,deep reinforcement learning,charging stations
AI 理解论文
溯源树
样例
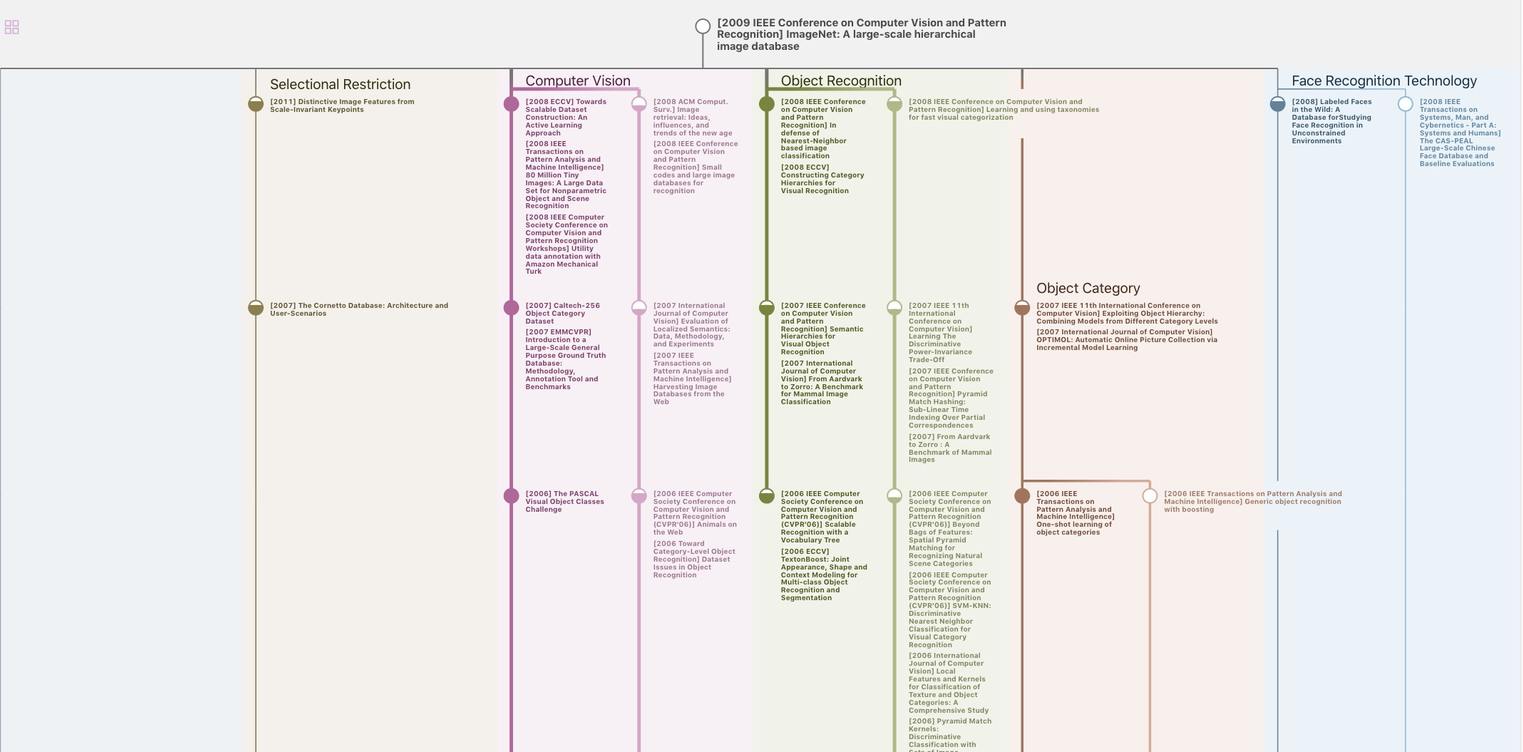
生成溯源树,研究论文发展脉络
Chat Paper
正在生成论文摘要