Combination of Peri-Tumoral and Intra-Tumoral Radiomic Features on Bi-Parametric MRI Accurately Stratifies Prostate Cancer Risk: A Multi-Site Study.
CANCERS(2020)
摘要
Background:Prostate cancer (PCa) influences its surrounding habitat, which tends to manifest as different phenotypic appearances on magnetic resonance imaging (MRI). This region surrounding the PCa lesion, or the peri-tumoral region, may encode useful information that can complement intra-tumoral information to enable better risk stratification.Purpose: To evaluate the role of peri-tumoral radiomic features on bi-parametric MRI (T2-weighted and Diffusion-weighted) to distinguish PCa risk categories as defined by D'Amico Risk Classification System.Materials and Methods: We studied a retrospective, HIPAA-compliant, 4-institution cohort of 231 PCa patients (n= 301 lesions) who underwent 3T multi-parametric MRI prior to biopsy. PCa regions of interest (ROIs) were delineated on MRI by experienced radiologists following which peri-tumoral ROIs were defined. Radiomic features were extracted within the intra- and peri-tumoral ROIs. Radiomic features differentiating low-risk from: (1) high-risk (L-vs.-H), and (2) (intermediate- and high-risk (L-vs.-I + H)) lesions were identified. Using a multi-institutional training cohort of 151 lesions (D1,N =116 patients), machine learning classifiers were trained using peri- and intra-tumoral features individually and in combination. The remaining 150 lesions (D2,N =115 patients) were used for independent hold-out validation and were evaluated using Receiver Operating Characteristic (ROC) analysis and compared with PI-RADS v2 scores.Results: Validation on D2 using peri-tumoral radiomics alone resulted in areas under the ROC curve (AUCs) of 0.84 and 0.73 for the L-vs.-H and L-vs.-I + H classifications, respectively. The best combination of intra- and peri-tumoral features resulted in AUCs of 0.87 and 0.75 for the L-vs.-H and L-vs.-I + H classifications, respectively. This combination improved the risk stratification results by 3-6% compared to intra-tumoral features alone. Our radiomics-based model resulted in a 53% accuracy in differentiating L-vs.-H compared to PI-RADS v2 (48%), on the validation set.Conclusion: Our findings suggest that peri-tumoral radiomic features derived from prostate bi-parametric MRI add independent predictive value to intra-tumoral radiomic features for PCa risk assessment.
更多查看译文
关键词
radiomics,prostate cancer,MRI,artificial intelligence,PIRADS,machine learning,peritumoral region
AI 理解论文
溯源树
样例
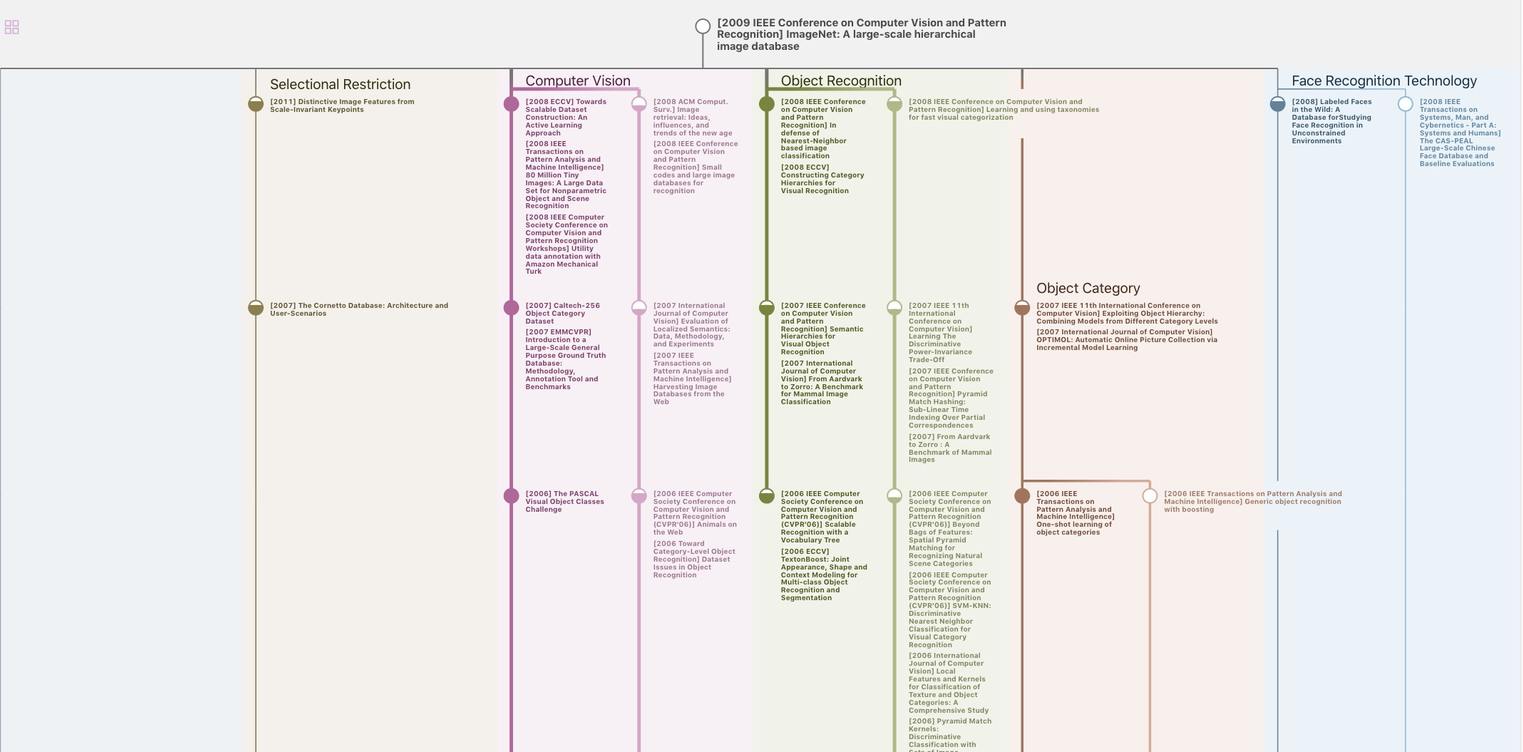
生成溯源树,研究论文发展脉络
Chat Paper
正在生成论文摘要