RUN-UP: Accelerated multishot diffusion-weighted MRI reconstruction using an unrolled network with U-Net as priors
MAGNETIC RESONANCE IN MEDICINE(2021)
摘要
Purpose To accelerate and improve multishot diffusion-weighted MRI reconstruction using deep learning. Methods An unrolled pipeline containing recurrences of model-based gradient updates and neural networks was introduced for accelerating multishot DWI reconstruction with shot-to-shot phase correction. The network was trained to predict results of jointly reconstructed multidirection data using single-direction data as input. In vivo brain and breast experiments were performed for evaluation. Results The proposed method achieves a reconstruction time of 0.1 second per image, over 100-fold faster than a shot locally low-rank reconstruction. The resultant image quality is comparable to the target from the joint reconstruction with a peak signal-to-noise ratio of 35.3 dB, a normalized root-mean-square error of 0.0177, and a structural similarity index of 0.944. The proposed method also improves upon the locally low-rank reconstruction (2.9 dB higher peak signal-to-noise ratio, 29% lower normalized root-mean-square error, and 0.037 higher structural similarity index). With training data from the brain, this method also generalizes well to breast diffusion-weighted imaging, and fine-tuning further reduces aliasing artifacts. Conclusion A proposed data-driven approach enables almost real-time reconstruction with improved image quality, which improves the feasibility of multishot DWI in a wide range of clinical and neuroscientific studies.
更多查看译文
关键词
convolution neural network, multishot diffusion-weighted imaging, phase variation, unrolled network
AI 理解论文
溯源树
样例
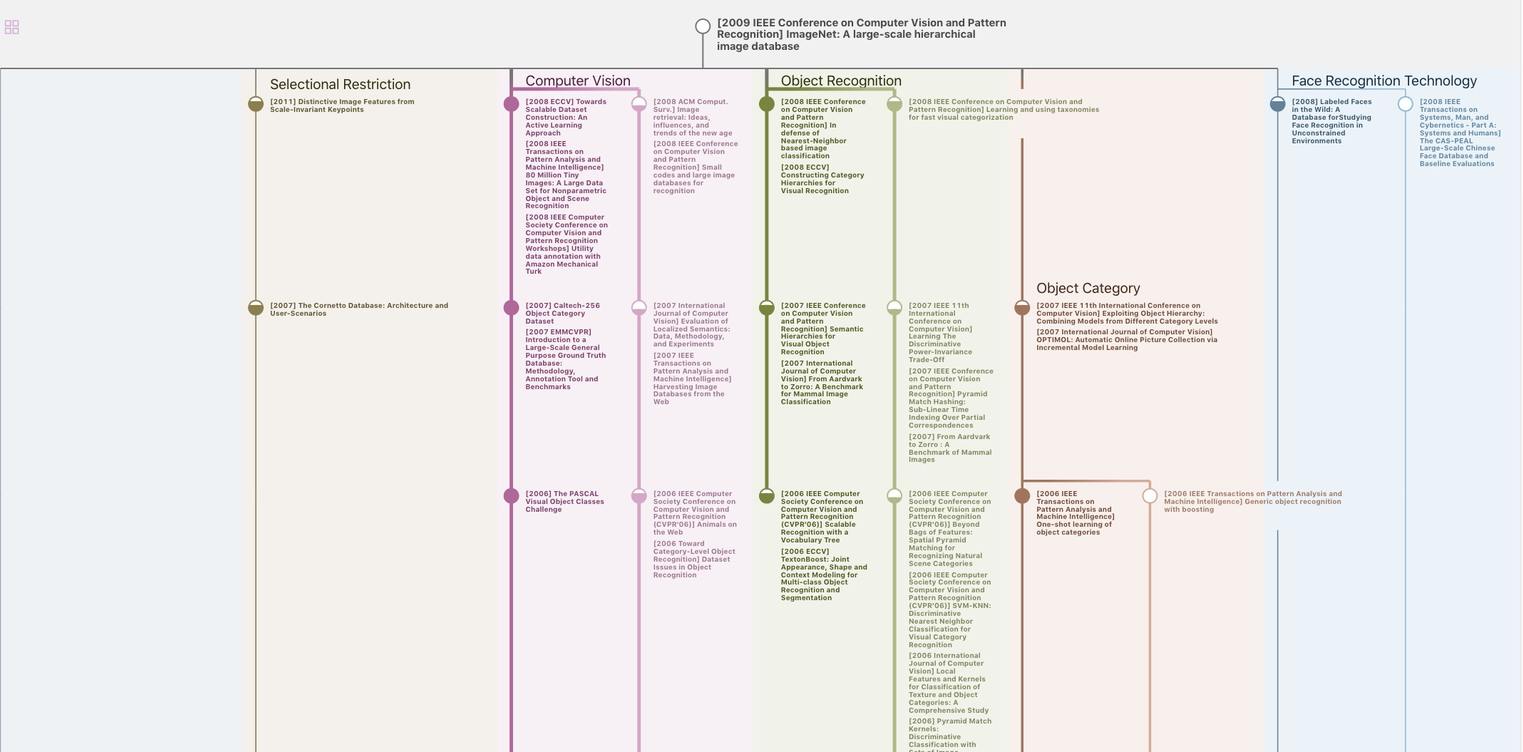
生成溯源树,研究论文发展脉络
Chat Paper
正在生成论文摘要