Incremental Meta-Learning via Indirect Discriminant Alignment
arXiv preprint arXiv:2002.04162(2020)
摘要
Majority of the modern meta-learning methods for few-shot classification tasks operate in two phases: a meta-training phase where the meta-learner learns a generic representation by solving multiple few-shot tasks sampled from a large dataset and a testing phase, where the meta-learner leverages its learnt internal representation for a specific few-shot task involving classes which were not seen during the meta-training phase. To the best of our knowledge, all such meta-learning methods use a single base dataset for meta-training to sample tasks from and do not adapt the algorithm after meta-training. This strategy may not scale to real-world use-cases where the meta-learner does not potentially have access to the full meta-training dataset from the very beginning and we need to update the meta-learner in an incremental fashion when additional training data becomes available. Through our experimental setup, we develop a notion of incremental learning during the meta-training phase of meta-learning and propose a method which can be used with multiple existing metric-based meta-learning algorithms. Experimental results on benchmark dataset show that our approach performs favorably at test time as compared to training a model with the full meta-training set and incurs negligible amount of catastrophic forgetting
更多查看译文
关键词
meta-learning
AI 理解论文
溯源树
样例
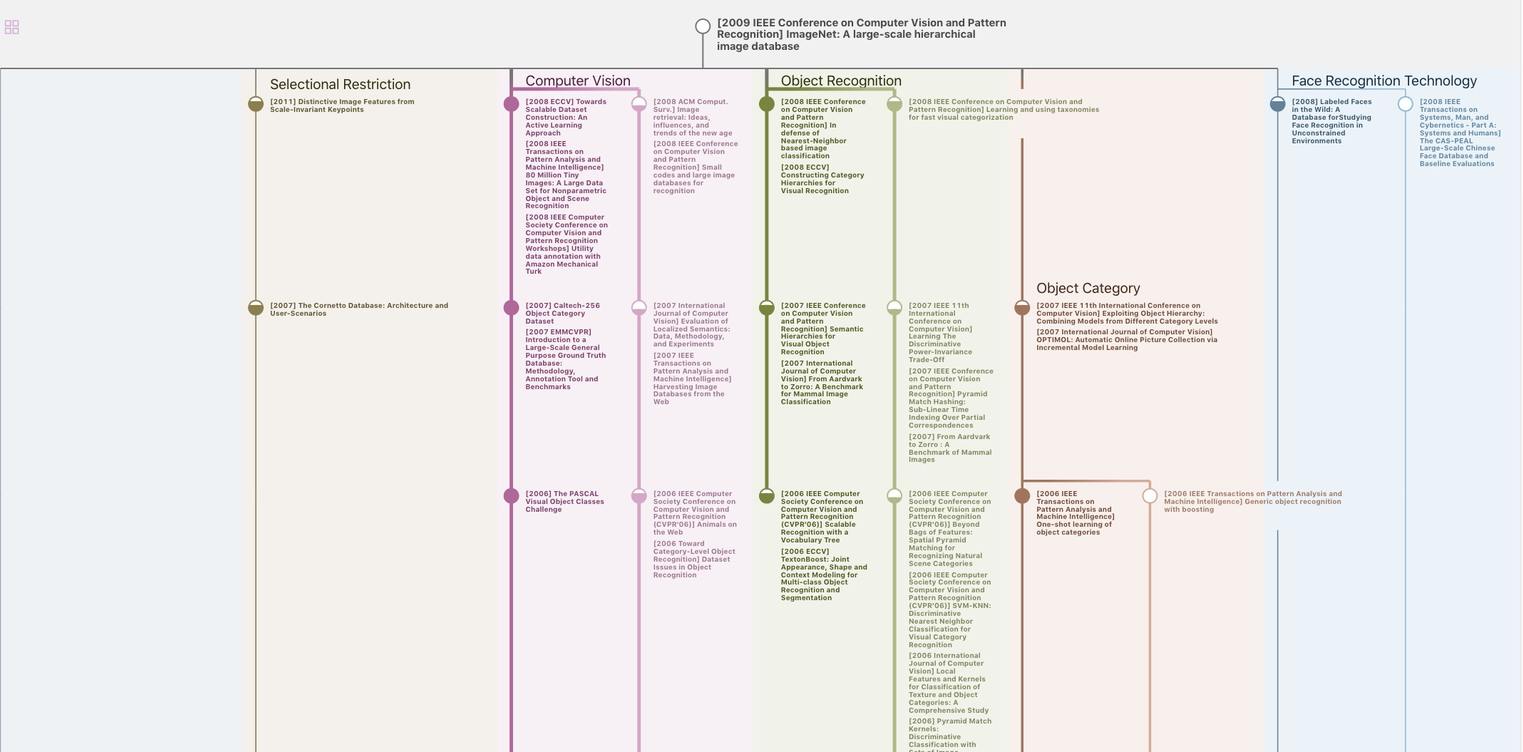
生成溯源树,研究论文发展脉络
Chat Paper
正在生成论文摘要