Renal Cell Carcinoma Detection and Subtyping with Minimal Point-Based Annotation in Whole-Slide Images
medical image computing and computer assisted intervention(2020)
摘要
Cancerous region detection and subtyping in whole-slide images (WSIs) are fundamental for renal cell carcinoma (RCC) diagnosis. The main challenge in the development of automated RCC diagnostic systems is the lack of large-scale datasets with precise annotations. In this paper, we propose a framework that employs a semi-supervised learning (SSL) method to accurately detect cancerous regions with a novel annotation method called Minimal Point-Based (Min-Point) annotation. The predicted results are efficiently utilized by a hybrid loss training strategy in a classification model for subtyping. The annotator only needs to mark a few cancerous and non-cancerous points in each WSI. The experiments on three significant subtypes of RCC proved that the performance of the cancerous region detector trained with the Min-Point annotated dataset is comparable to the classifiers trained on the dataset with full cancerous region delineation. In subtyping, the proposed model outperforms the model trained with only whole-slide diagnostic labels by 12% in terms of the testing f1-score. We believe that our “detect then classify” schema combined with the Min-Point annotation would set a standard for developing intelligent systems with similar challenges.
更多查看译文
关键词
renal,cell carcinoma,whole-slide whole-slide images,annotation,point-based
AI 理解论文
溯源树
样例
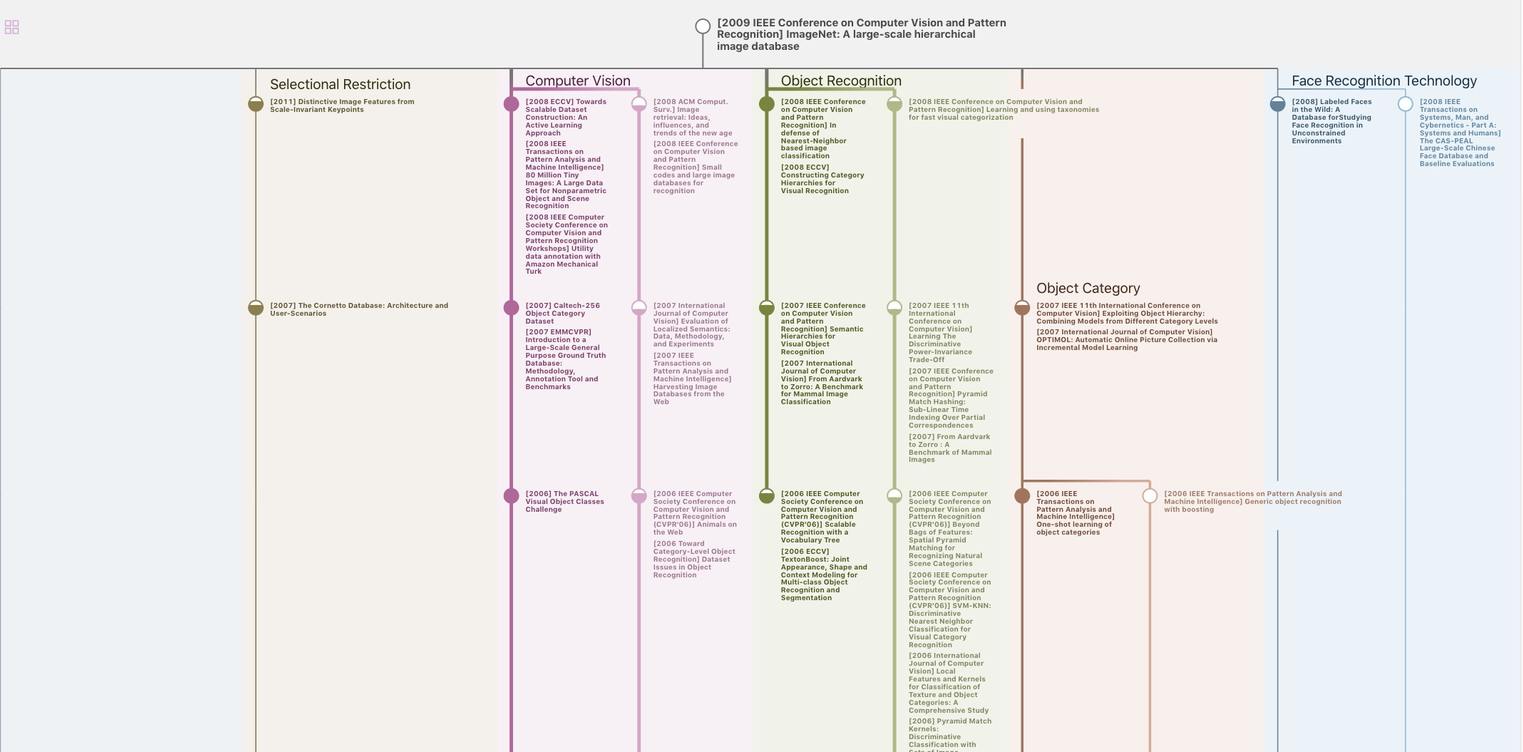
生成溯源树,研究论文发展脉络
Chat Paper
正在生成论文摘要