Conditional multiple point simulation with a texture synthesis algorithm
IAMG 2009 Conference, Stanford University(2009)
摘要
Simulation of categorical variables has seen a fast development with multiple point geostatistical simulation techniques. Conventional approaches such as sequential indicator simulation, truncated Gaussian and pluriGaussian simulation are based on variograms which summarize the relationship between pairs of points separated by a distance, but cannot account for complex relationships of multiple points simultaneously. In addition to this difficulty, truncated Gaussian and pluriGaussian simulation require computing the conditioning data and variograms for the Gaussian variables from the categorical data, which is cumbersome for practitioners. Object based models can capture the intricacy of geological features, but may fail to condition to hard data.These limitations can be overcome by considering multiple point simulation that uses pattern statistics. Inference is no longer done from a set of scattered hard data, but is based on a training image that depicts the spatial structure that is interpreted by the geologist. The patterns from this training image must be anchored to the hard data. Several methods have been proposed to provide conditional realizations respecting the spatial patterns of a training image. The single normal equation simulation (snesim) is probably the most popular (Strebelle, 2002) and has been the basis for the development of several variants. Some alternatives have also been proposed, such as a growth algorithm, a Gibbs Sampler based approach, simulated annealing and neural networks (Ortiz, 2008).
更多查看译文
AI 理解论文
溯源树
样例
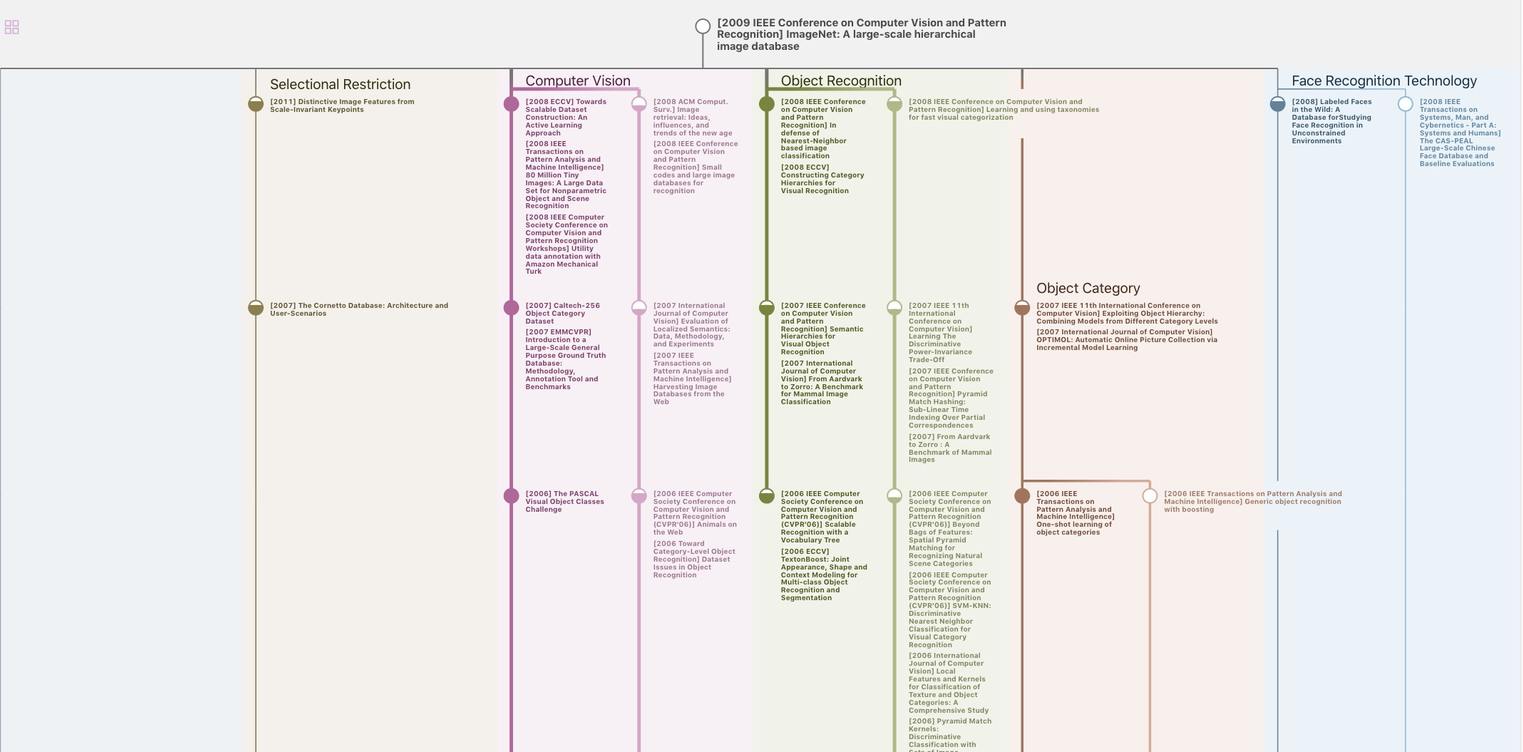
生成溯源树,研究论文发展脉络
Chat Paper
正在生成论文摘要