Morphological Characterization Of Functional Brain Imaging By Isosurface Analysis In Parkinson'S Disease
INTERNATIONAL JOURNAL OF NEURAL SYSTEMS(2020)
摘要
Finding new biomarkers to model Parkinson's Disease (PD) is a challenge not only to help discerning between Healthy Control (HC) subjects and patients with potential PD but also as a way to measure quantitatively the loss of dopaminergic neurons mainly concentrated at substantia nigra. Within this context, this work presented here tries to provide a set of imaging features based on morphological characteristics extracted from I[123]-Ioflupane SPECT scans to discern between HC and PD participants in a balanced set of 386 scans from Parkinson's Progression Markers Initiative (PPMI) database. These features, obtained from isosurfaces of each scan at different intensity levels, have been classified through the use of classical Machine Learning classifiers such as Support-Vector-Machines (SVM) or Naive Bayesian and compared with the results obtained using a Multi-Layer Perceptron (MLP).The proposed system, based on a Mann-Whitney-Wilcoxon U-Test for feature selection and the SVM approach, yielded a 97.04% balanced accuracy when the performance was evaluated using a 10-fold cross-validation. This proves the reliability of these biomarkers, especially those related to sphericity, center of mass, number of vertices, 2D-projected perimeter or the 2D-projected eccentricity, among others, but including both internal and external isosurfaces.
更多查看译文
关键词
Parkinson's disease, neuroimaging, machine learning, isosurfaces, Parkinson's Progression Markers Initiative (PPMI), Single Photon Emission Computed Tomography (SPECT), Computer-Aided-Diagnosis (CAD), supervised learning
AI 理解论文
溯源树
样例
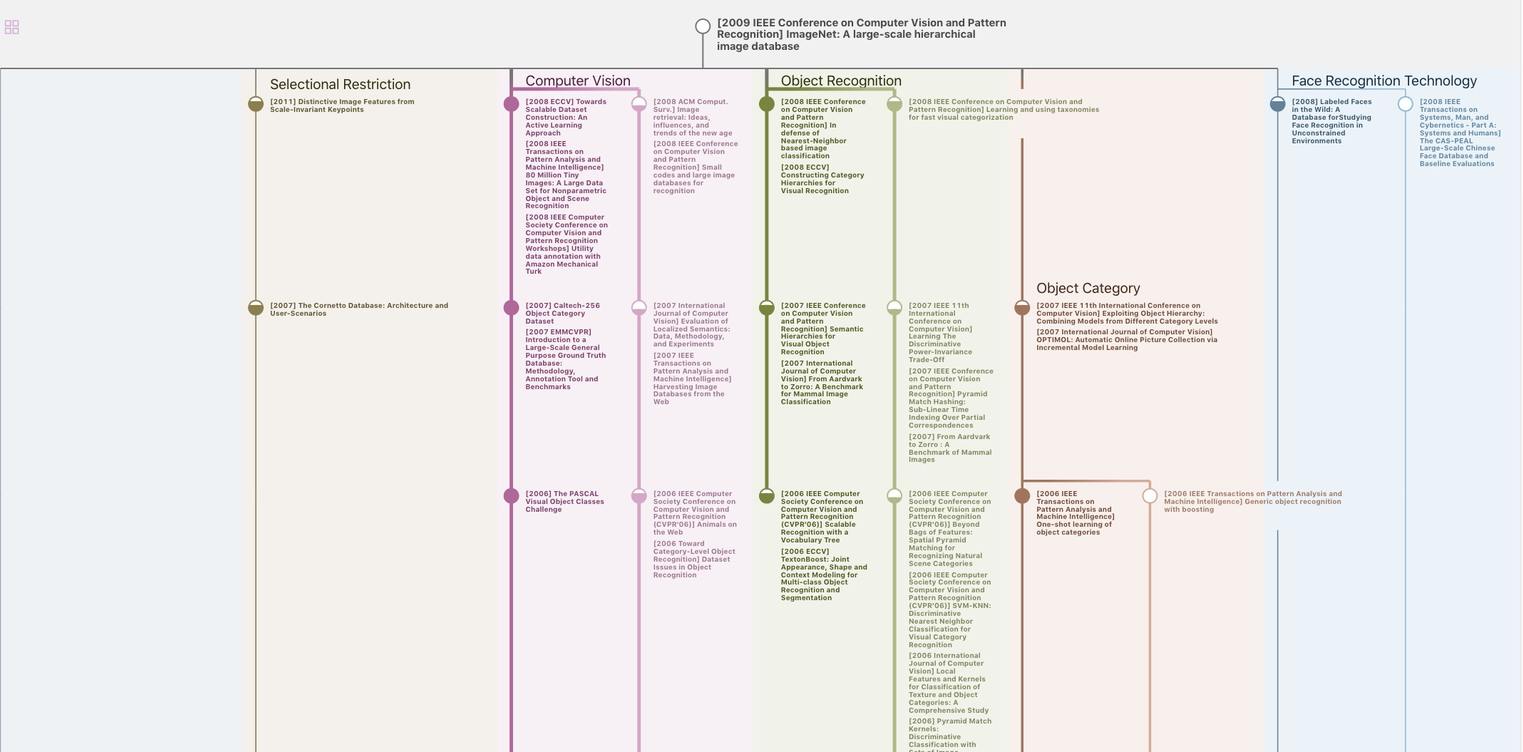
生成溯源树,研究论文发展脉络
Chat Paper
正在生成论文摘要