Cloudscout: A Deep Neural Network For On-Board Cloud Detection On Hyperspectral Images
REMOTE SENSING(2020)
摘要
The increasing demand for high-resolution hyperspectral images from nano and microsatellites conflicts with the strict bandwidth constraints for downlink transmission. A possible approach to mitigate this problem consists in reducing the amount of data to transmit to ground through on-board processing of hyperspectral images. In this paper, we propose a customConvolutional Neural Network (CNN)deployed for a nanosatellite payload to select images eligible for transmission to ground, calledCloudScout. The latter is installed on the Hyperscout-2, in the frame of the Phisat-1 ESA mission, which exploits a hyperspectral camera to classify cloud-covered images and clear ones. The images transmitted to ground are those that present less than 70% of cloudiness in a frame. We train and test the network against an extracted dataset from the Sentinel-2 mission, which was appropriately pre-processed to emulate the Hyperscout-2 hyperspectral sensor. On the test set we achieve 92% ofaccuracywith 1% ofFalse Positives (FP). The Phisat-1 mission will start in 2020 and will operate for about 6 months. It represents the first in-orbit demonstration of Deep Neural Network (DNN) for data processing on the edge. The innovation aspect of our work concerns not only cloud detection but in general low power, low latency, and embedded applications. Our work should enable a new era of edge applications and enhance remote sensing applications directly on-board satellite.
更多查看译文
关键词
earth observation,on-board,microsat,mission,nanosat,hyperspectral images,AI on the edge,CNN
AI 理解论文
溯源树
样例
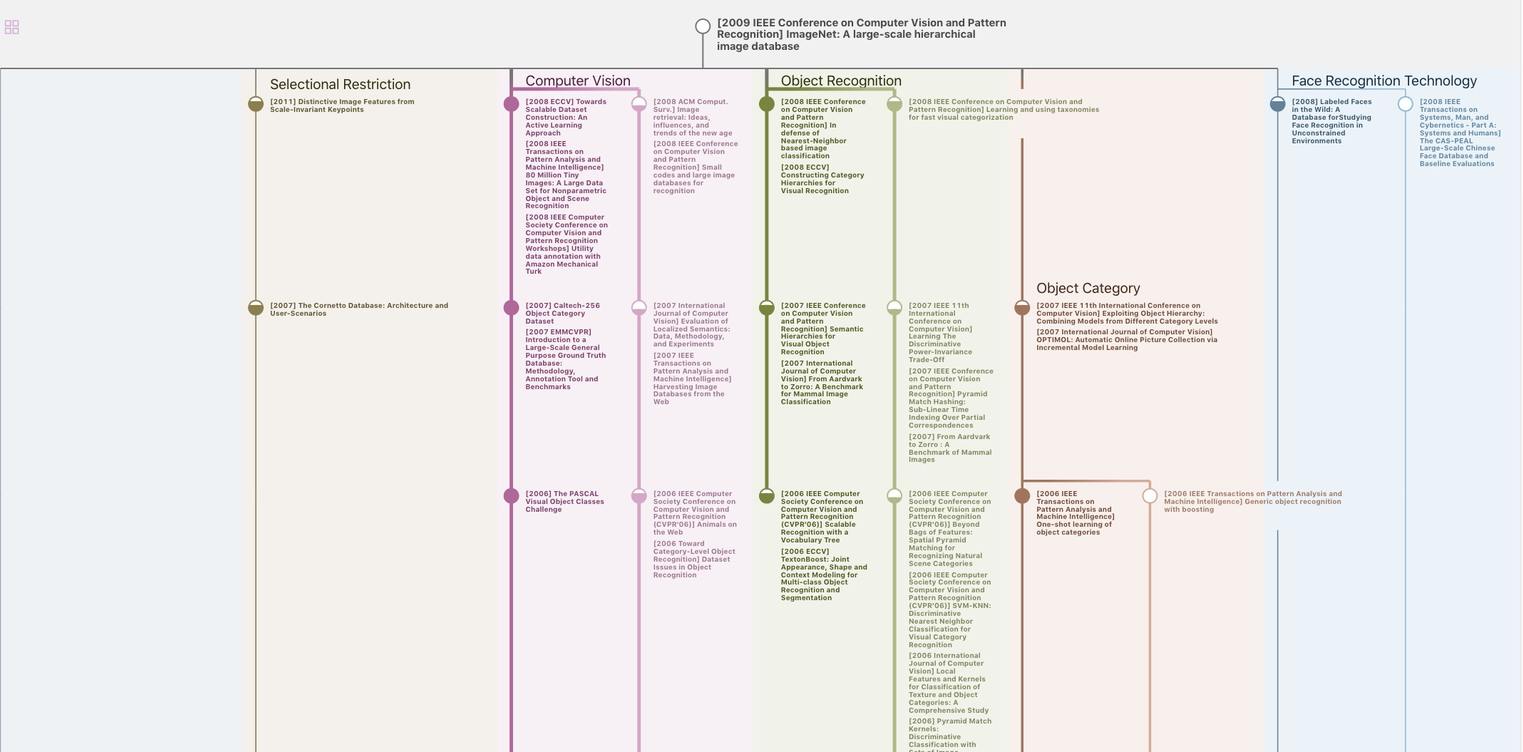
生成溯源树,研究论文发展脉络
Chat Paper
正在生成论文摘要