Drug-target binding quantitatively predicts optimal antibiotic dose levels in quinolones.
PLOS COMPUTATIONAL BIOLOGY(2020)
摘要
Author summary Antibiotic efficacy is traditionally assessed with a single value, the minimal inhibitory concentration, despite its limited predictive power. Dose-response curves that assess efficacy over a range of antibiotic concentrations are promising, but extremely work intensive. Using the well-characterized antibiotics ciprofloxacin and ampicillin as proof-of-concept, we provide a mathematical framework that allows predicting optimal dosing of antibiotics based on drug-target binding parameters. These could be measured early in drug development and can also change when bacteria become resistant. Thus, we can predict the probability that resistant strains are selected at a given dosing regimen without isolating and testing a multitude of resistant strains. Predicting optimal dosing with early accessible experimental data can reduce the prevalent trial-and-error approaches and thereby the number of antibiotic candidates that fail late in drug development. Antibiotic resistance is rising and we urgently need to gain a better quantitative understanding of how antibiotics act, which in turn would also speed up the development of new antibiotics. Here, we describe a computational model (COMBAT-COmputational Model of Bacterial Antibiotic Target-binding) that can quantitatively predict antibiotic dose-response relationships. Our goal is dual: We address a fundamental biological question and investigate how drug-target binding shapes antibiotic action. We also create a tool that can predict antibiotic efficacy a priori. COMBAT requires measurable biochemical parameters of drug-target interaction and can be directly fitted to time-kill curves. As a proof-of-concept, we first investigate the utility of COMBAT with antibiotics belonging to the widely used quinolone class. COMBAT can predict antibiotic efficacy in clinical isolates for quinolones from drug affinity (R-2>0.9). To further challenge our approach, we also do the reverse: estimate the magnitude of changes in drug-target binding based on antibiotic dose-response curves. We overexpress target molecules to infer changes in antibiotic-target binding from changes in antimicrobial efficacy of ciprofloxacin with 92-94% accuracy. To test the generality of our approach, we use the beta-lactam ampicillin to predict target molecule occupancy at MIC from antimicrobial action with 90% accuracy. Finally, we apply COMBAT to predict antibiotic concentrations that can select for resistance due to novel resistance mutations. Using ciprofloxacin and ampicillin as well defined test cases, our work demonstrates that drug-target binding is a major predictor of bacterial responses to antibiotics. This is surprising because antibiotic action involves many additional effects downstream of drug-target binding. In addition, COMBAT provides a framework to inform optimal antibiotic dose levels that maximize efficacy and minimize the rise of resistant mutants.
更多查看译文
AI 理解论文
溯源树
样例
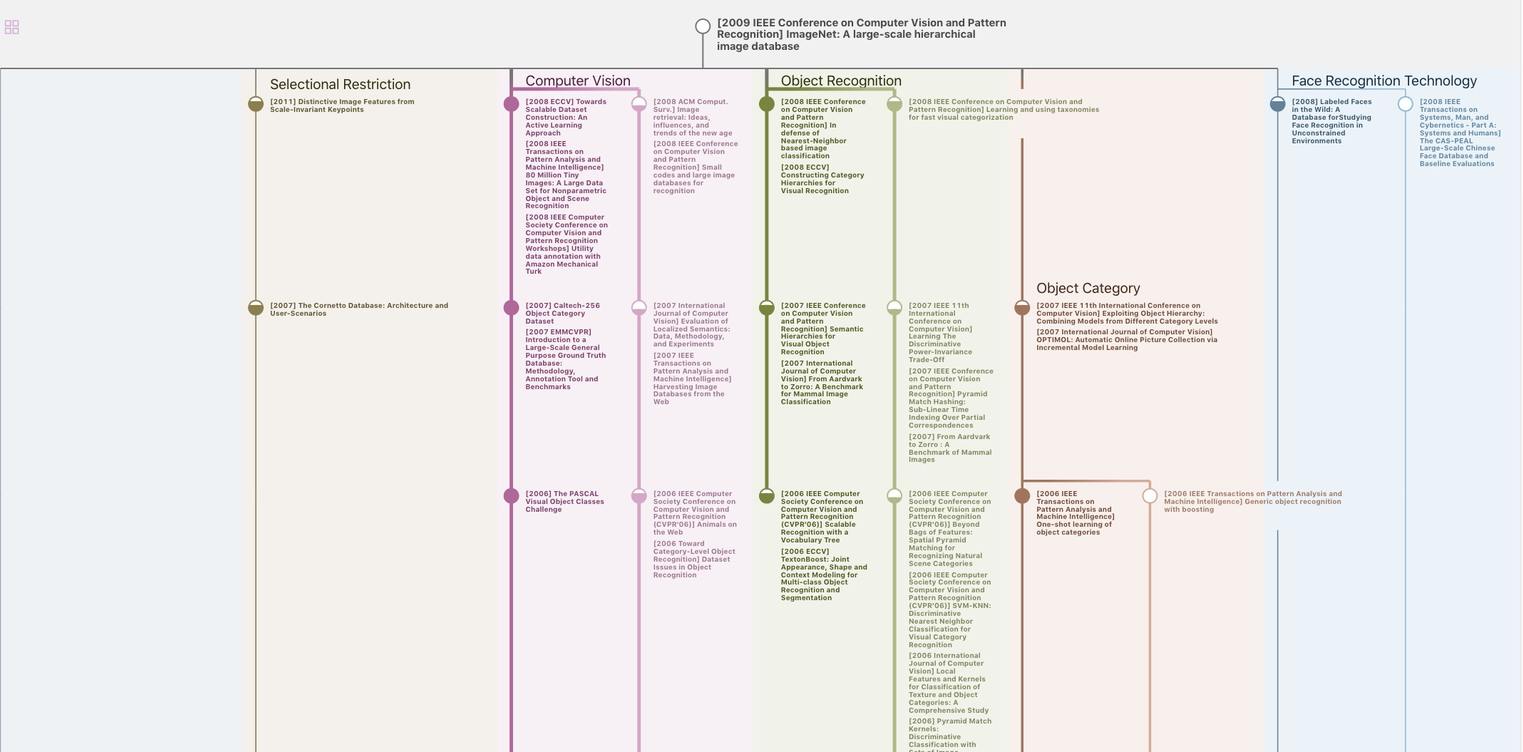
生成溯源树,研究论文发展脉络
Chat Paper
正在生成论文摘要