A learning style classification approach based on deep belief network for large-scale online education
Journal of Cloud Computing(2020)
摘要
With the rapidly growing demand for large-scale online education and the advent of big data, numerous research works have been performed to enhance learning quality in e-learning environments. Among these studies, adaptive learning has become an increasingly important issue. The traditional classification approaches analyze only the surface characteristics of students but fail to classify students accurately in terms of deep learning features. Meanwhile, these approaches are unable to analyze these high-dimensional learning behaviors in massive amounts of data. Hence, we propose a learning style classification approach based on the deep belief network (DBN) for large-scale online education to identify students’ learning styles and classify them. The first step is to build a learning style model and identify indicators of learning style based on the experiences of experts; then, relate the indicators to the different learning styles. We improve the DBN model and identify a student’s learning style by analyzing each individual’s learning style features using the improved DBN. Finally, we verify the DBN result by conducting practical experiments on an actual educational dataset. The various learning styles are determined by soliciting questionnaires from students based on the ILS theory by Felder and Soloman (1996) and the Readiness for Education At a Distance Indicator. Then, we utilized those data to train our DBNLS model. The experimental results indicate that the proposed DBNLS method has better accuracy than do the traditional approaches.
更多查看译文
关键词
Large-scale online education, Adaptive learning, Deep belief network, Learning style, High-dimensional
AI 理解论文
溯源树
样例
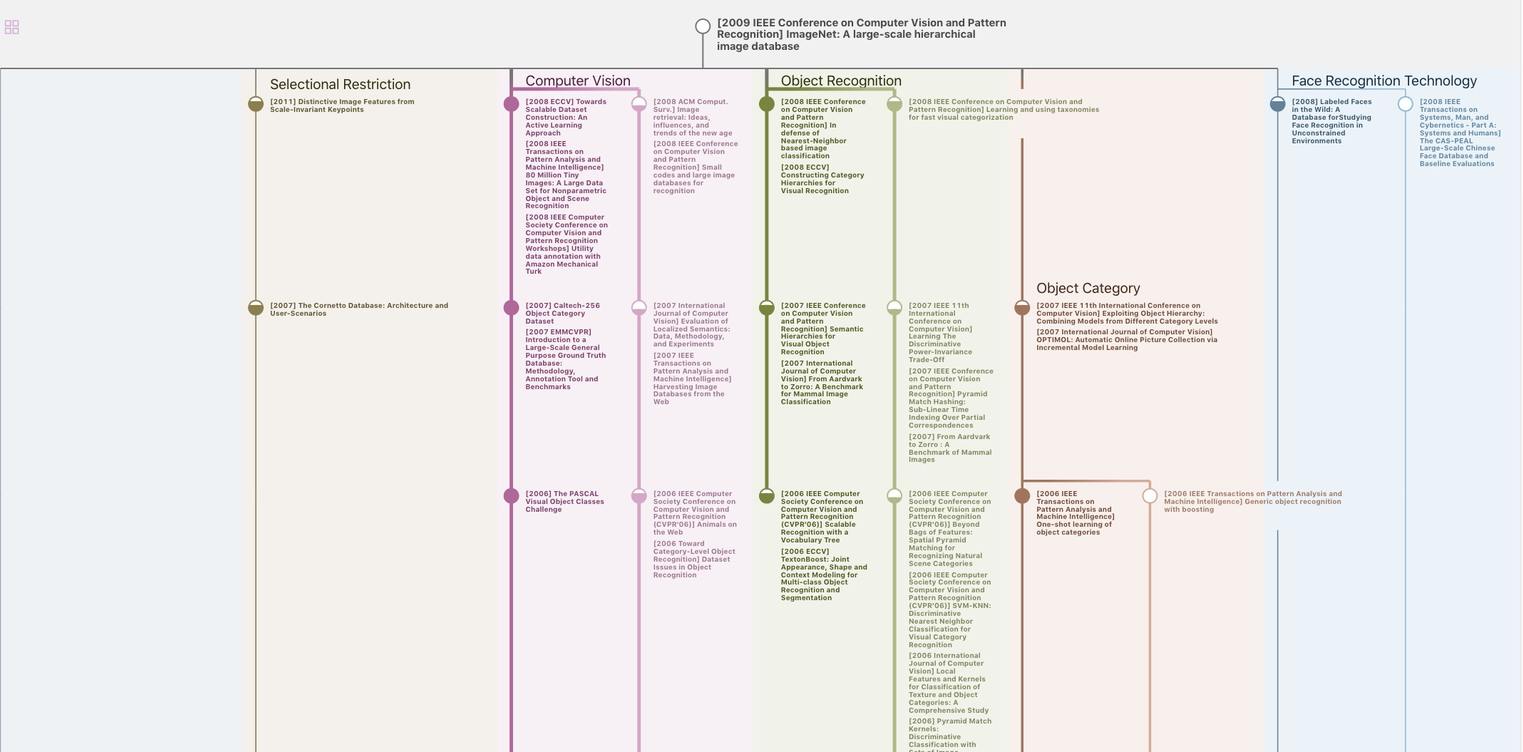
生成溯源树,研究论文发展脉络
Chat Paper
正在生成论文摘要