A Hybrid Analytical And Data-Driven Modeling Approach For Calibration Of Heavy-Duty Cartesian Robot
2020 IEEE/ASME INTERNATIONAL CONFERENCE ON ADVANCED INTELLIGENT MECHATRONICS (AIM)(2020)
摘要
Robot calibration is to enhance absolute positioning accuracy within robotic task space. Traditional method is to build the geometric error model and identify the deviation of kinematic parameters. In this paper, a hybrid analytical and data-driven non-geometric error modeling approach is proposed after the geometric error is compensated. It is intended to satisfy higher accuracy requirements when the heavy-duty Cartesian robot performs metal finishing tasks under different load conditions. Analytical and deterministic Beam Deformation model is built firstly to remove a large portion of the non-geometric error. By this means, data-driven Gaussian Process Regression model can achieve better prediction results due to the collected residual error data is closer to Gaussian distribution. The experimental result of platform tests demonstrates the effectiveness and robustness of the proposed method.
更多查看译文
关键词
metal finishing tasks,deterministic Beam Deformation model,datadriven Gaussian Process Regression model,collected residual error data,data-driven modeling approach,heavy-duty Cartesian robot,robot calibration,absolute positioning accuracy,robotic task space,geometric error model,higher accuracy requirements
AI 理解论文
溯源树
样例
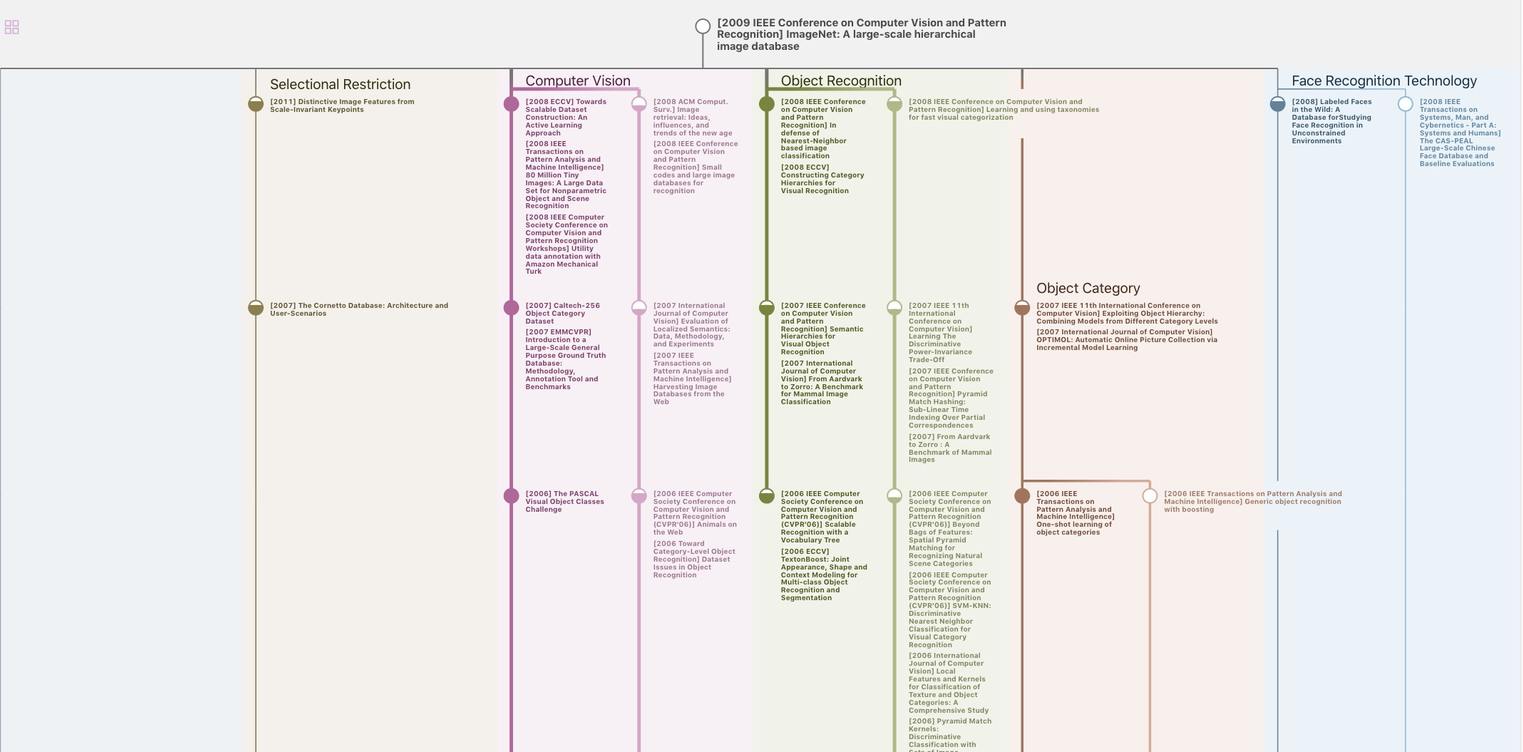
生成溯源树,研究论文发展脉络
Chat Paper
正在生成论文摘要