In-situ Terrain Classification and Estimation for NASA’s Humanoid Robot Valkyrie
2020 IEEE/ASME International Conference on Advanced Intelligent Mechatronics (AIM)(2020)
摘要
This research is aimed at investigating the interaction for a bipedal humanoid robot during locomotion on deformable terrains. The knowledge of deformable terrain properties has major implications in modelling the robot walking dynamics, which is the key to achieve stable gait patterns. We propose an in-situ terrain classification and estimation method in this paper to provide robots with such terrain properties. In particular, we introduce our method for classifying known terrains using recurrent neural networks (RNNs) and estimating the unknown terrain's stiffness adopting the Bernstein-Goriatchkin model. We present our experimental procedures and analysis of the resulting data using NASA's humanoid robot, Valkyrie, while it is performing a set of prespecified motions on different terrains, including gym foam mat, wood mulch, rubber mulch, and sand. It is concluded that using RNNs, we can efficiently classify the terrain types. For unknown terrains, our approach can estimate the terrain's stiffness that demonstrates support forces under deformation.
更多查看译文
关键词
robot walking dynamics,stable gait patterns,in-situ terrain classification,estimation method,known terrains,recurrent neural networks,RNNs,unknown terrain,Bernstein-Goriatchkin model,experimental procedures,different terrains,terrain types,unknown terrains,NASA's humanoid robot Valkyrie,bipedal humanoid robot,deformable terrains,deformable terrain properties
AI 理解论文
溯源树
样例
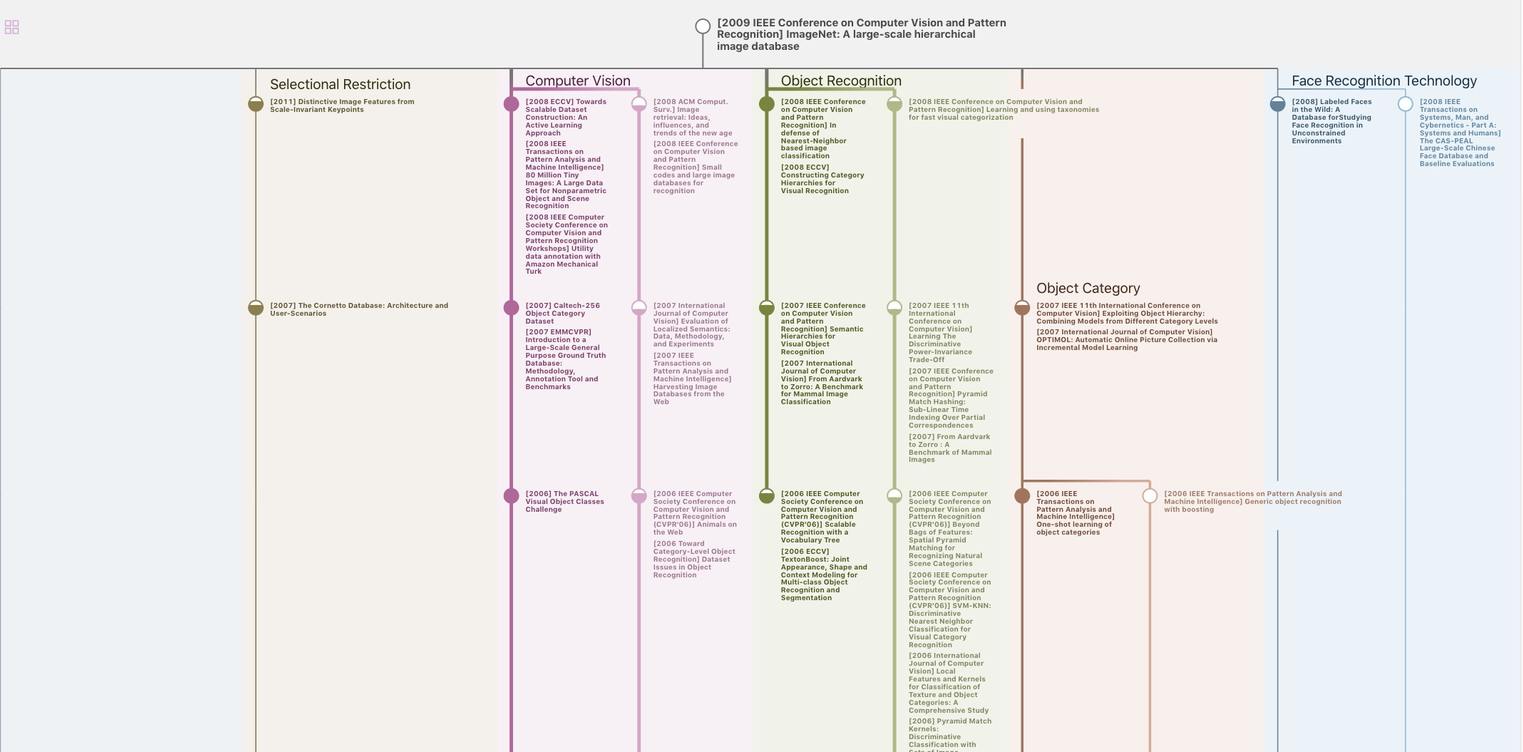
生成溯源树,研究论文发展脉络
Chat Paper
正在生成论文摘要