A framework to build bespoke auto tuners with structured Bayesian optimisation
user-5efd71244c775ed682ed8a03(2017)
摘要
Due to their complexity, modern computer systems expose many configuration parameters which users must manually tune to maximise the performance of their applications. To relieve users of this burden, auto-tuning has emerged as an alternative in which a black-box optimiser iteratively evaluates configurations to find efficient ones. A popular auto-tuning technique is Bayesian optimisation, which uses the results to incrementally build a probabilistic model of the impact of the parameters on performance. This allows the optimisation to quickly focus on efficient regions of the configuration space. Unfortunately, for many computer systems, either the configuration space is too large to develop a good model, or the time to evaluate performance is too long to be executed many times.
更多查看译文
AI 理解论文
溯源树
样例
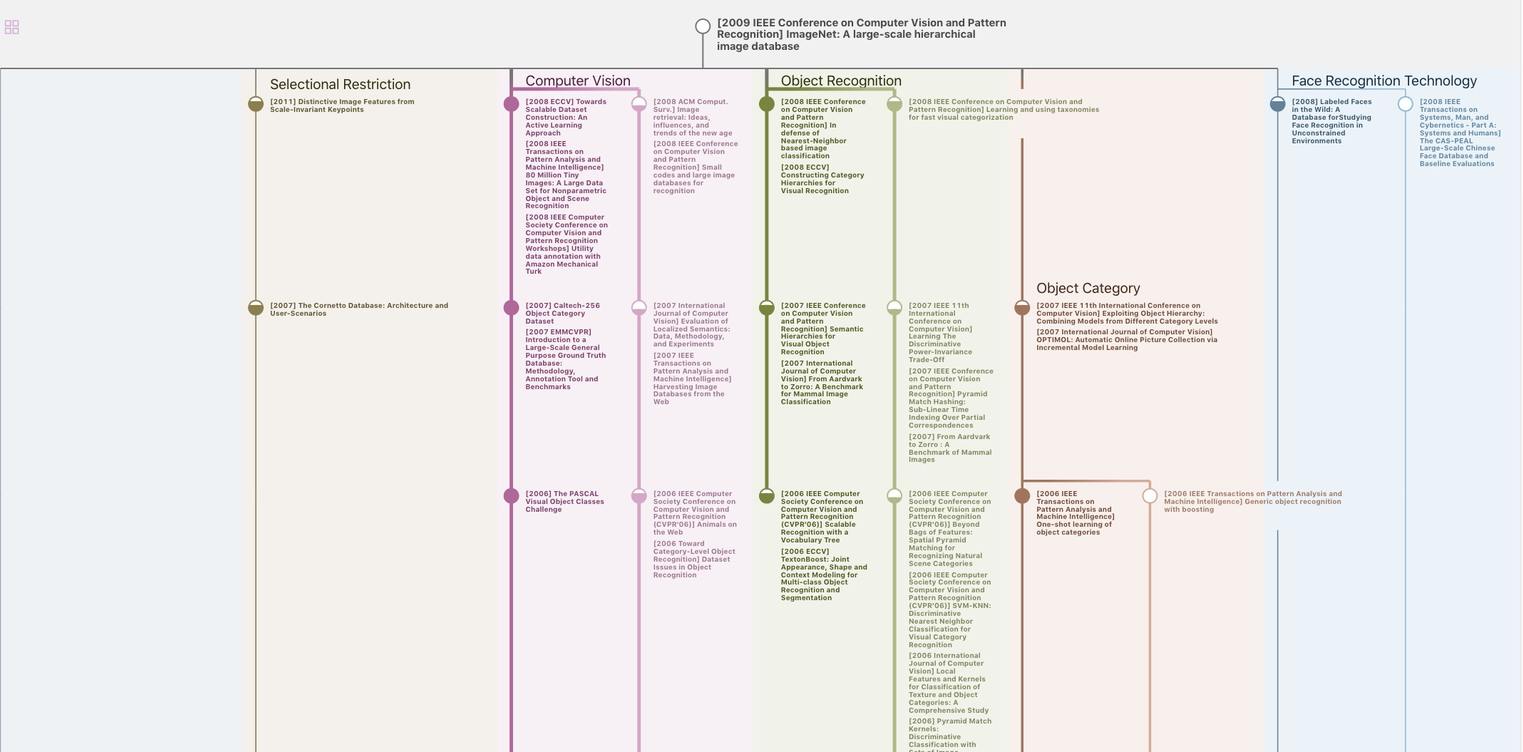
生成溯源树,研究论文发展脉络
Chat Paper
正在生成论文摘要