A partial imputation EM-algorithm to adjust the overestimated shape parameter of the Weibull distribution fitted to the clinical time-to-event data.
Computer methods and programs in biomedicine(2020)
摘要
BACKGROUND AND OBJECTIVES:Typical clinical data can suffer routine information loss when event times are rounded to the nearest day and right-censored at the end of follow-up. Because of the daily basis recording system, for the first 24 h, there are no events, which can damage the estimation of the Weibull survival model. Its estimation bias is inevitable since, for this short period, massive events might have occurred, the data is missing, and the fitted Weibull model is to show a steep slope. This phenomenon of estimation bias caused by the information loss caused by the problem of measurement resolution has not been properly discussed so far.
METHODS:We propose a partial imputation Expectation Maximization (PIEM)-algorithm to estimate missing lifetimes only for day 1 at the mode among the whole clinical follow-up days. Based on various Weibull distributions, we simulated clinical sets after rounding and censoring raw event times and prepared chimera sets by partially substituting the imputed lifetimes only for the 24 h at the mode among the entire clinical sets.
RESULTS:For shape parameter ≤ 1, almost all the 95% prediction intervals (PIs) of both parameters in the chimera sets include their true values, while those in the clinical sets miss most of the true shape parameters and some of the true scale parameters. Estimating a small proportion of missing data only for the 24 h period, while keeping the rest as they are, greatly reduces biases of both scale and shape parameters. For shape parameter >1, the chimera sets consistently outperform the clinical sets.
CONCLUSIONS:The PIEM-algorithm may be applied as an intuitive tool for time-to-event modeling of survival data with this kind of information loss.
更多查看译文
AI 理解论文
溯源树
样例
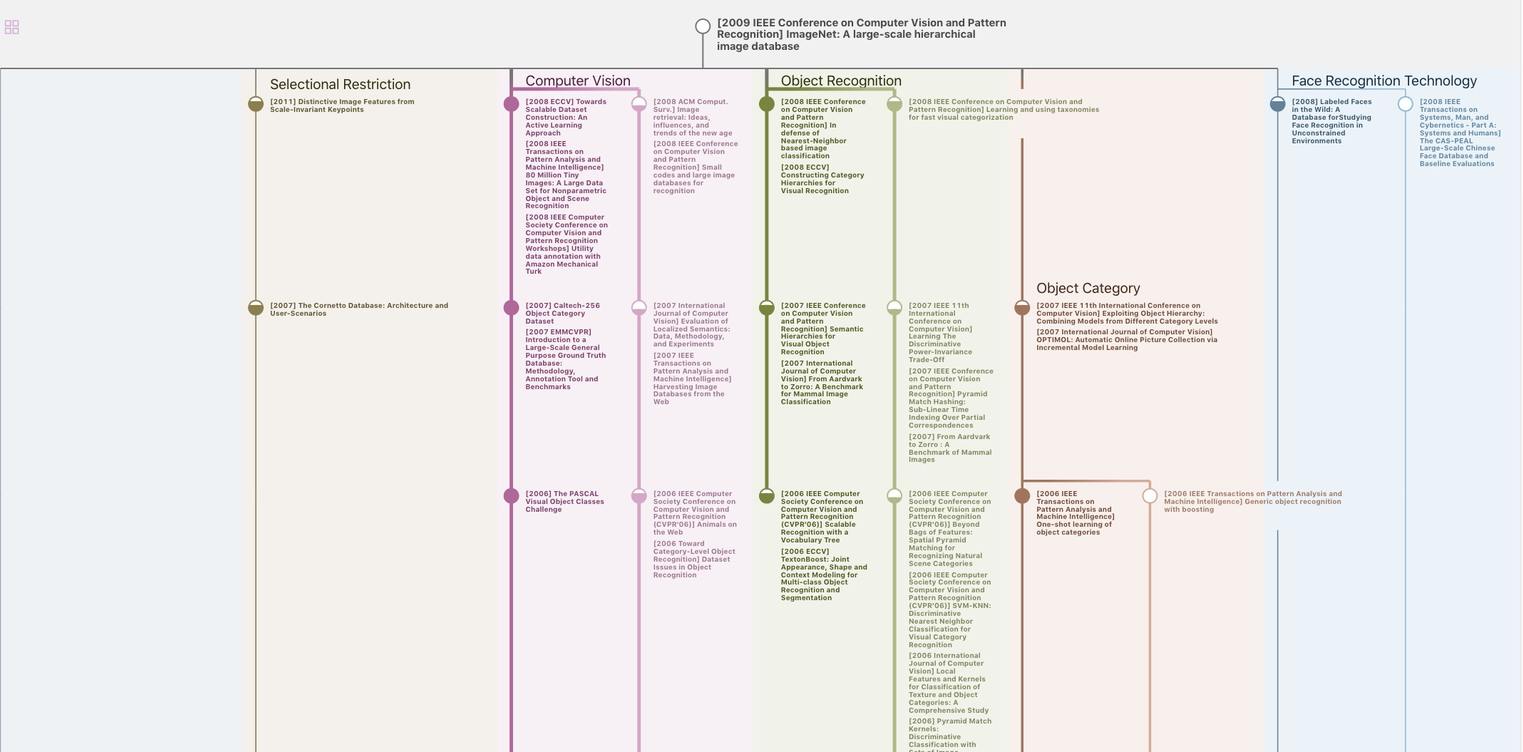
生成溯源树,研究论文发展脉络
Chat Paper
正在生成论文摘要