Shape and texture-based radiomics signature on CT effectively discriminates benign from malignant renal masses
EUROPEAN RADIOLOGY(2020)
摘要
Objectives Using a radiomics framework to quantitatively analyze tumor shape and texture features in three dimensions, we tested its ability to objectively and robustly distinguish between benign and malignant renal masses. We assessed the relative contributions of shape and texture metrics separately and together in the prediction model. Materials and methods Computed tomography (CT) images of 735 patients with 539 malignant and 196 benign masses were segmented in this retrospective study. Thirty-three shape and 760 texture metrics were calculated per tumor. Tumor classification models using shape, texture, and both metrics were built using random forest and AdaBoost with tenfold cross-validation. Sensitivity analyses on five sub-cohorts with respect to the acquisition phase were conducted. Additional sensitivity analyses after multiple imputation were also conducted. Model performance was assessed using AUC. Results Random forest classifier showed shape metrics featuring within the top 10% performing metrics regardless of phase, attaining the highest variable importance in the corticomedullary phase. Convex hull perimeter ratio is a consistently high-performing shape feature. Shape metrics alone achieved an AUC ranging 0.64–0.68 across multiple classifiers, compared with 0.67–0.75 and 0.68–0.75 achieved by texture-only and combined models, respectively. Conclusion Shape metrics alone attain high prediction performance and high variable importance in the combined model, while being independent of the acquisition phase (unlike texture). Shape analysis therefore should not be overlooked in its potential to distinguish benign from malignant tumors, and future radiomics platforms powered by machine learning should harness both shape and texture metrics. Key Points • Current radiomics research is heavily weighted towards texture analysis, but quantitative shape metrics should not be ignored in their potential to distinguish benign from malignant renal tumors. • Shape metrics alone can attain high prediction performance and demonstrate high variable importance in the combined shape and texture radiomics model. • Any future radiomics platform powered by machine learning should harness both shape and texture metrics, especially since tumor shape (unlike texture) is independent of the acquisition phase and more robust from the imaging variations.
更多查看译文
关键词
Tomography, X-ray computed,Kidney neoplasms,Data analysis,Machine learning,Image Processing, radiomics
AI 理解论文
溯源树
样例
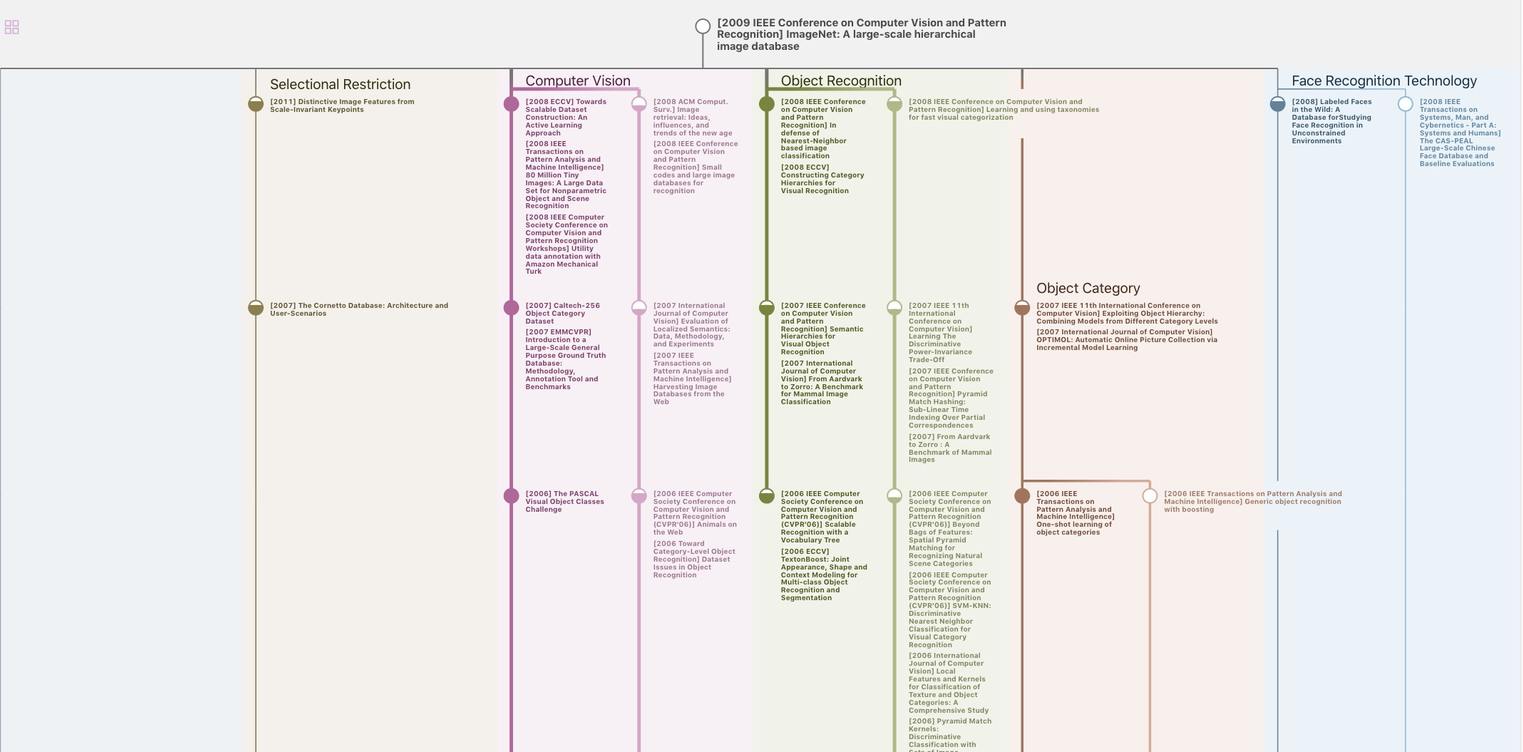
生成溯源树,研究论文发展脉络
Chat Paper
正在生成论文摘要