Time-Supervised Primary Object Segmentation
arxiv(2020)
摘要
We describe an unsupervised method to detect and segment portions of live scenes that, at some point in time, are seen moving as a coherent whole, which we refer to as primary objects. Our method first segments motions by minimizing the mutual information between partitions of the image domain, which bootstraps a static object detection model that takes a single image as input. The two models are mutually reinforced within a feedback loop, enabling extrapolation to previously unseen classes of objects. Our method requires video for training, but can be used on either static images or videos at inference time. As the volume of our training sets grows, more and more objects are seen moving, thus turning our method into unsupervised (or time-supervised) training to segment primary objects. The resulting system outperforms the state-of-the-art in both video object segmentation and salient object detection benchmarks, even when compared to methods that use explicit manual annotation.
更多查看译文
关键词
object,time-supervised
AI 理解论文
溯源树
样例
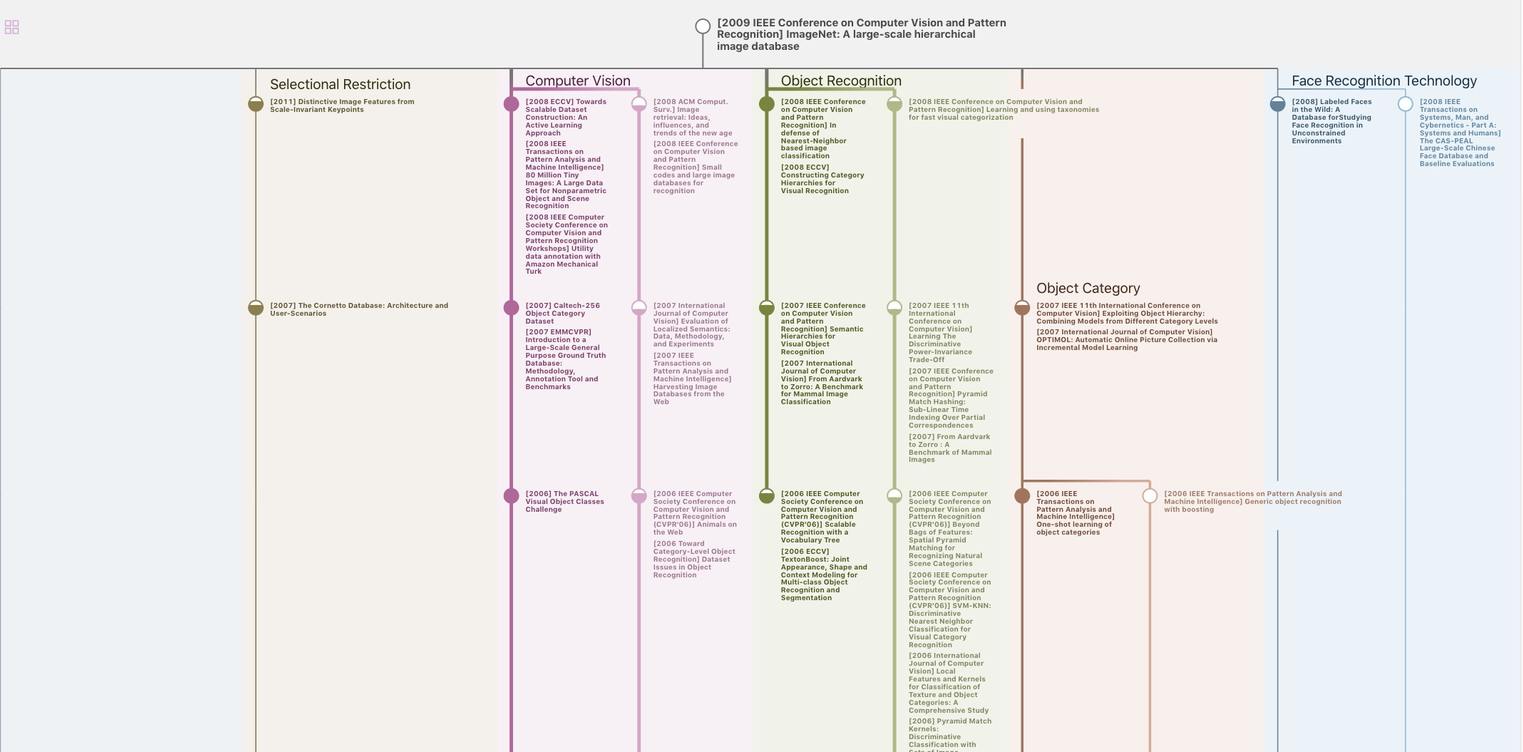
生成溯源树,研究论文发展脉络
Chat Paper
正在生成论文摘要