MIDAS: Multi-agent Interaction-aware Decision-making with Adaptive Strategies for Urban Autonomous Navigation
2021 IEEE INTERNATIONAL CONFERENCE ON ROBOTICS AND AUTOMATION (ICRA 2021)(2021)
摘要
Autonomous navigation in crowded, complex urban environments requires interacting with other agents on the road. A common solution to this problem is to use a prediction model to guess the likely future actions of other agents. While this is reasonable, it leads to overly conservative plans because it does not explicitly model the mutual influence of the actions of interacting agents. This paper builds a reinforcement learning-based method named MIDAS where an Ego agent learns to affect the control actions of other cars in urban driving scenarios. MIDAS uses an attention mechanism to handle an arbitrary number of other agents and includes a "driver-type" parameter to learn a single policy that works across different planning objectives. We build a simulation environment that enables diverse interaction experiments with a large number of agents and develop methods for quantitatively studying the safety, efficiency, and interaction among vehicles. MIDAS is validated using extensive experiments and we show that it (i) can work across different road geometries, (ii) results in an adaptive Ego policy that can be tuned easily to satisfy different performance criteria, such as aggressive or cautious driving, (iii) is robust to changes in the driving policies of external agents, and (iv) is safer and more efficient than existing approaches to interaction-aware decision-making. Code available here.
更多查看译文
关键词
interaction-aware driving, urban autonomous navigation, reinforcement learning
AI 理解论文
溯源树
样例
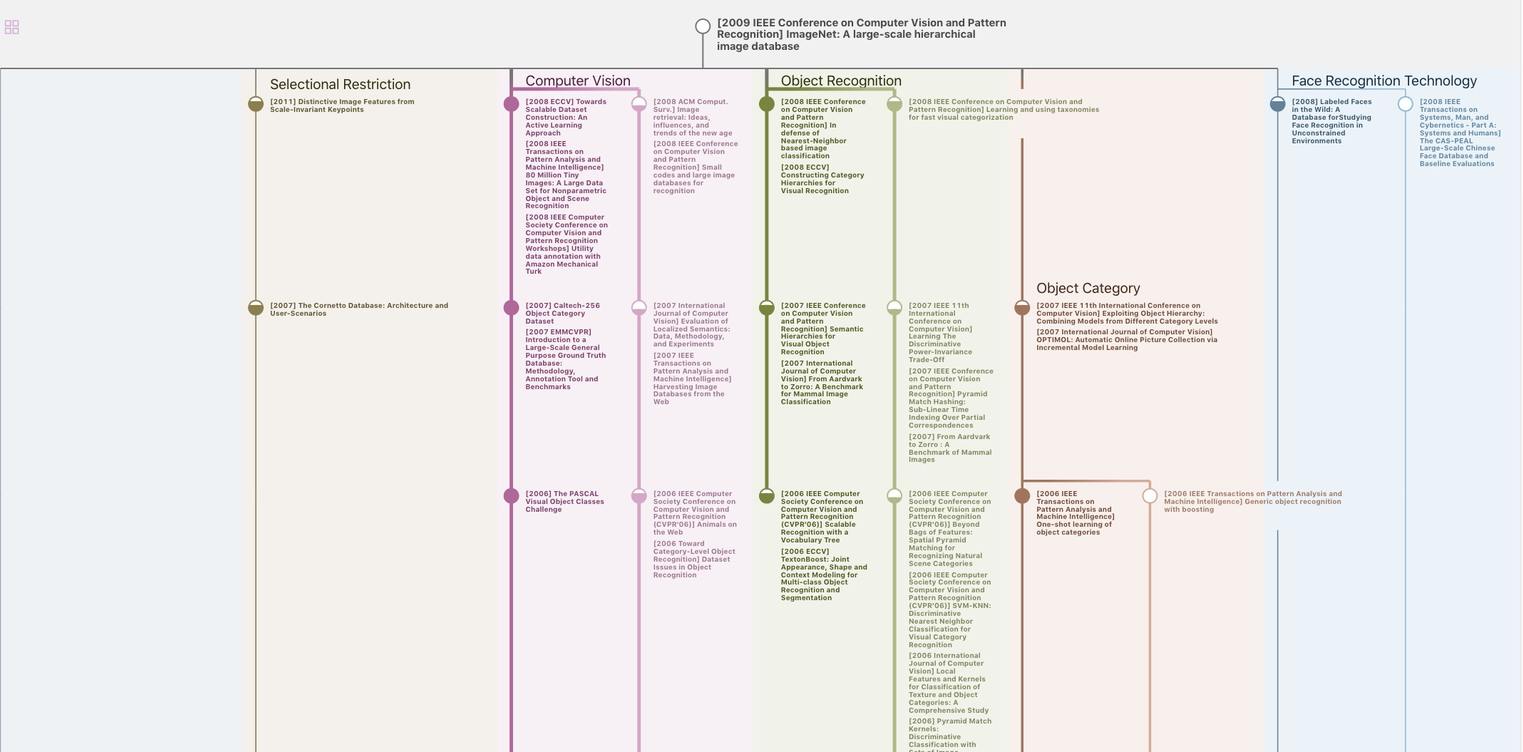
生成溯源树,研究论文发展脉络
Chat Paper
正在生成论文摘要