Non-reversible Markov chain Monte Carlo for sampling of districting maps
arXiv (Cornell University)(2020)
摘要
Evaluating the degree of partisan districting (Gerrymandering) in a statistical framework typically requires an ensemble of districting plans which are drawn from a prescribed probability distribution that adheres to a realistic and non-partisan criteria. In this article we introduce novel non-reversible Markov chain Monte-Carlo (MCMC) methods for the sampling of such districting plans which have improved mixing properties in comparison to previously used (reversible) MCMC algorithms. In doing so we extend the current framework for construction of non-reversible Markov chains on discrete sampling spaces by considering a generalization of skew detailed balance. We provide a detailed description of the proposed algorithms and evaluate their performance in numerical experiments.
更多查看译文
关键词
maps,non-reversible
AI 理解论文
溯源树
样例
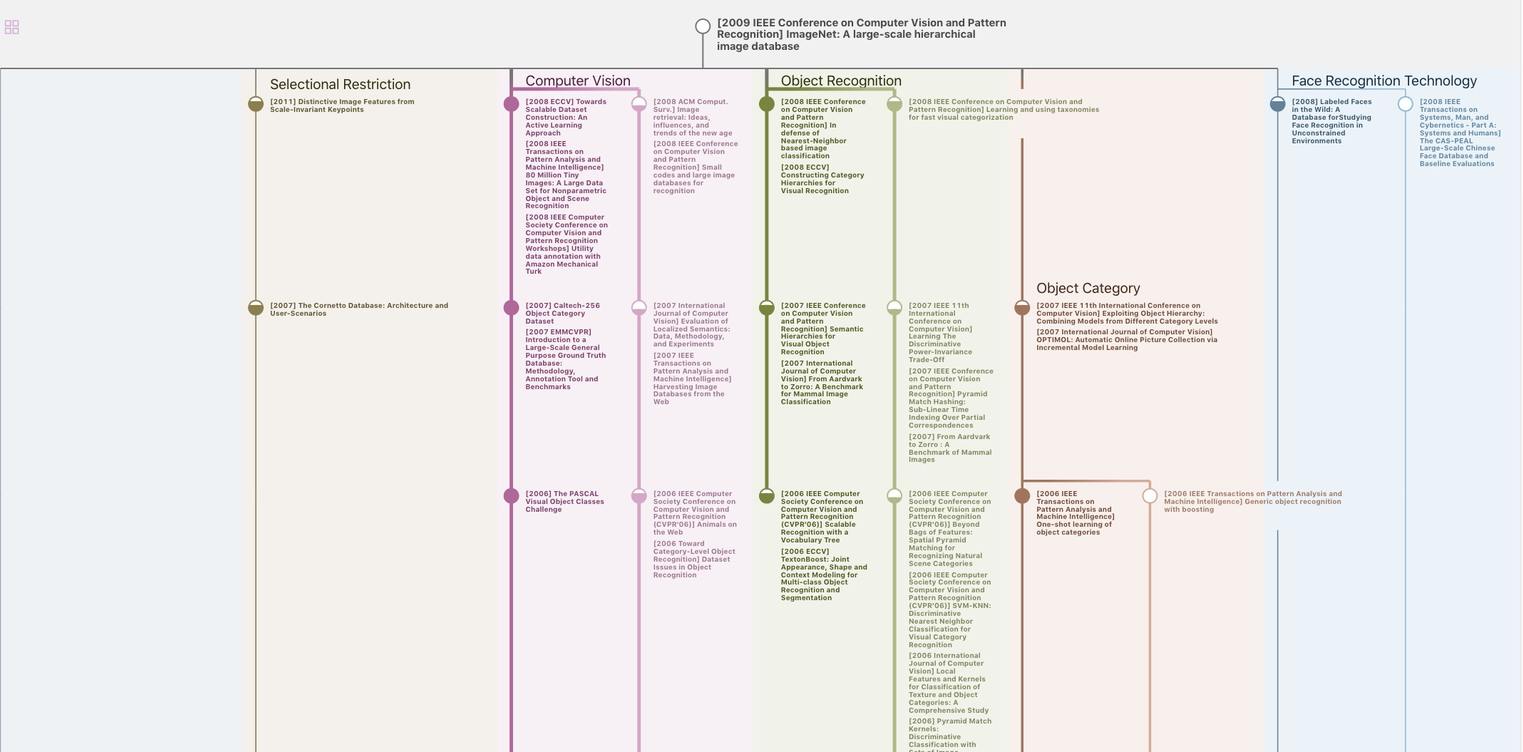
生成溯源树,研究论文发展脉络
Chat Paper
正在生成论文摘要