Self-supervised Denoising via Diffeomorphic Template Estimation: Application to Optical Coherence Tomography
OMIA@MICCAI(2020)
摘要
Optical Coherence Tomography (OCT) is pervasive in both the research and clinical practice of Ophthalmology. However, OCT images are strongly corrupted by noise, limiting their interpretation. Current OCT denoisers leverage assumptions on noise distributions or generate targets for training deep supervised denoisers via averaging of repeat acquisitions. However, recent self-supervised advances allow the training of deep denoising networks using only repeat acquisitions without clean targets as ground truth, reducing the burden of supervised learning. Despite the clear advantages of self-supervised methods, their use is precluded as OCT shows strong structural deformations even between sequential scans of the same subject due to involuntary eye motion. Further, direct nonlinear alignment of repeats induces correlation of the noise between images. In this paper, we propose a joint diffeomorphic template estimation and denoising framework which enables the use of self-supervised denoising for motion deformed repeat acquisitions, without empirically registering their noise realizations. Strong qualitative and quantitative improvements are achieved in denoising OCT images, with generic utility in any imaging modality amenable to multiple exposures.
更多查看译文
关键词
diffeomorphic template estimation
AI 理解论文
溯源树
样例
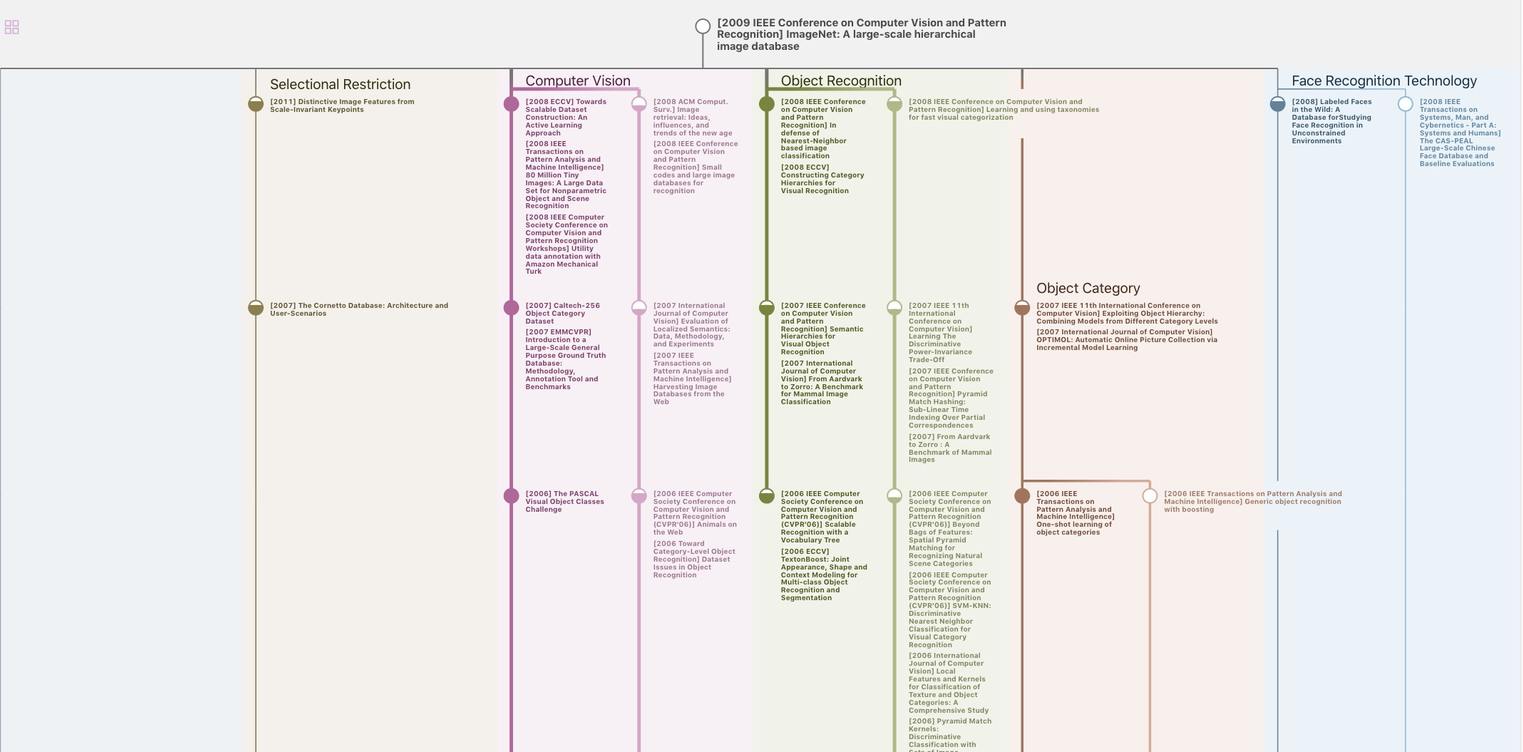
生成溯源树,研究论文发展脉络
Chat Paper
正在生成论文摘要